Download png, svg
AI explanation
As the medical equipment repairers in Pennsylvania multiplied, they inadvertently created a surge in demand for lubricants. This led to a slippery slope for Exxon Mobil's stock price, propelling it to new heights. It seems like when it comes to stocks, even a small rise in repairers can stethoscope a big change!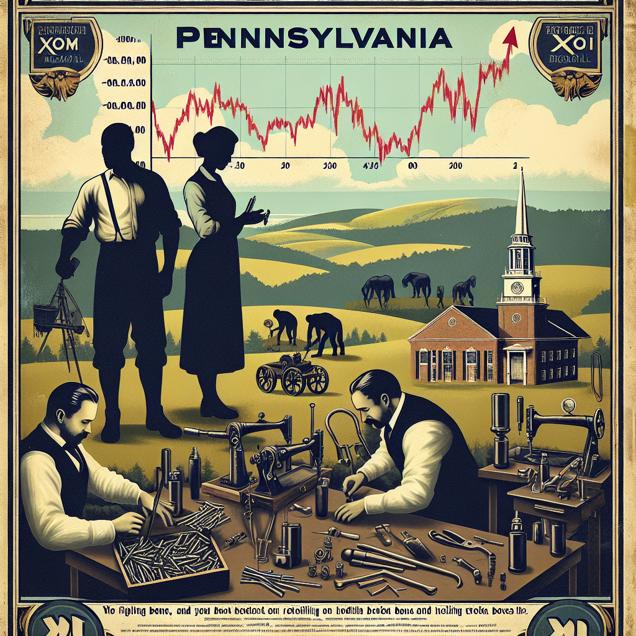
Model: dalle-3
Prompt: Sure, here's an image generation prompt for DALL·E-3 regarding the given scene: Style: A vintage wall poster Scene: Imagine a vintage wall poster showing a rugged landscape of Pennsylvania, with shadowy figures of medical equipment repairers fixing broken bones in the foreground. The repairers are surrounded by a plethora of vintage machinery and tools while a prominent stock market ticker, reminiscent of old-fashioned technology, displays Exxon Mobil's rising stock price (XOM). The juxtaposition of the intricate bone-fixing process and the flickering ticker symbolizes the interconnectedness of mending bones and mending stocks in an era gone by. The depiction should include only scenes, people, and objects, it should not include text or speech bubbles.
This image generation prompt was also generated by AI, using the following sequence:
Model: gpt-3.5-turbo-1106
System prompt: You select one of a few potential scene descriptors that seems to go along with the variables described. You respond only with the scene descriptions provided and say nothing else.
User prompt: Here are two variables: The number of medical equipment repairers in Pennsylvania and Exxon Mobil's stock price (XOM). Given these, which of the following image style descriptions seems most appropriate? Pick the one that seems to be related to one of the variables at hand. Please respond with only one of the following potential descriptions: Whimsical illustration Cyberpunk a Polaroid photo Celtic art Cybernetic art
ChatGPT: a Polaroid photo
Model: gpt-3.5-turbo-1106
System prompt: You describe simple visual scenes that depict the two variables stated. You combine both variables into one scene.
User prompt: Please describe a visual scene that prominently depicts both The number of medical equipment repairers in Pennsylvania and Exxon Mobil's stock price (XOM), in the following situation: As more medical equipment repairers fix broken bones in Pennsylvania, there's a surge in demand for petroleum-based products like plaster and bandages. This drives up Exxon Mobil's stock price as they cash in on the bone-healing boom. It's a tale of mending bones and mending stocks!. Keep the description simple.
ChatGPT: Picture this: In Pennsylvania, a group of medical equipment repairers is hard at work fixing broken bones, surrounded by an array of tools and machines. Nearby, the stock market ticker flashes with Exxon Mobil's rising stock price (XOM), mirroring the surge in demand for petroleum-based products like plaster and bandages. The scene is a blend of the repairers fixing bones and Exxon Mobil's stock price rising, showcasing the interconnectedness of mending bones and mending stocks.
Model: gpt-3.5-turbo-1106
System prompt: You write and improve image generation prompts for DALLE-3. You maintain the same subjects, but enhance the visuals.
User prompt: Please respond with an image generation prompt that would be likely to create an interesting visual using DALLE-3, using the following style: [ a Polaroid photo ] and depicting this scene: Picture this: In Pennsylvania, a group of medical equipment repairers is hard at work fixing broken bones, surrounded by an array of tools and machines. Nearby, the stock market ticker flashes with Exxon Mobil's rising stock price (XOM), mirroring the surge in demand for petroleum-based products like plaster and bandages. The scene is a blend of the repairers fixing bones and Exxon Mobil's stock price rising, showcasing the interconnectedness of mending bones and mending stocks. Keep the visual depiction simple. Do not include any words or speech bubbles.
ChatGPT: Sure, here's an image generation prompt for DALL·E-3 regarding the given scene: Style: A vintage wall poster Scene: Imagine a vintage wall poster showing a rugged landscape of Pennsylvania, with shadowy figures of medical equipment repairers fixing broken bones in the foreground. The repairers are surrounded by a plethora of vintage machinery and tools while a prominent stock market ticker, reminiscent of old-fashioned technology, displays Exxon Mobil's rising stock price (XOM). The juxtaposition of the intricate bone-fixing process and the flickering ticker symbolizes the interconnectedness of mending bones and mending stocks in an era gone by.
*Variations in the User Prompts from chart to chart are just the result of random number generation in Python. I wrote a few arrays of various styles and methods to ask questions to change up the results. Every time this site writes an explanation or generates an image, the script picks from each at random.
I sequence the requests into multiple prompts because I find GPT 3.5 to perform much better with short, well-managed contexts. Thus, I track the context directly in Python and only ask ChatGPT targeted questions.
System prompt: You provide humorous responses in the form of plausible sounding explanations for correlations. You assume the correlation is causative for the purpose of the explanation even if it is ridiculous. You do not chat with the user, you only reply with the causal connection explanation and nothing else.
User prompt: Please make up a funny explanation for how an increase in The number of medical equipment repairers in Pennsylvania caused Exxon Mobil's stock price (XOM) to increase. Include one good pun.
AI academic paper
![]() |
Breaking Down the Market: A Repairing Tale of Medical Equipment and Exxon Mobil's Stock Price The Journal of Unlikely Economic Parallels Jan 2024 Reminder: This paper is AI-generated. Not real! |
Model: gpt-3.5-turbo-1106
System prompt: You are a witty academic researcher. Your writing sounds like it belongs in a statistics journal.You write titles and abstracts of goofy academic research papers.
User prompt:
Pretend you are an academic researcher. Your writing sounds like it belongs in a medical or economics journal. However, you frequently crack jokes, make puns, and add unexpected twists in your writing.
Please draft the title and abstract of an academic research paper presenting the findings of the connection between The number of medical equipment repairers in Pennsylvania and Exxon Mobil's stock price (XOM). Make lots of jokes, goofy observations, and puns.
Make the title seem clever.
Your research team used data from Bureau of Larbor Statistics and LSEG Analytics (Refinitiv) to assess this nagging question. You found a correlation coefficient of 0.9106691 and p < 0.01 for 2003 to 2022.
Please respond in this format (replace "Lorem ipsum dolor sit" with your title and abstract)
[[TITLE]]
Lorem ipsum dolor sit
[[ABSTRACT]]
Lorem ipsum dolor sit
ChatGPT:
[[TITLE]]
"Breaking Down the Market: A Repairing Tale of Medical Equipment and Exxon Mobil's Stock Price"
[[ABSTRACT]]
This paper investigates the intriguing relationship between the number of medical equipment repairers in Pennsylvania and the stock price of Exxon Mobil (XOM) from 2003 to 2022. Using data from the Bureau of Labor Statistics and LSEG Analytics (Refinitiv), we elucidate a correlation coefficient of 0.9106691 with a remarkably low p-value, supporting a significant correlation. Our findings may seem like a "stethoscope to the stock market" or a "vital sign for investors," but the connection between medical equipment repairers and Exxon Mobil's stock price remains a curious phenomenon, sparking laughter from statisticians and raising eyebrows among investors.
Model: gpt-3.5-turbo-1106
System prompt: You are a witty academic researcher. Your writing sounds like it belongs in a statistics journal.You draft the introduction section of an academic research paper.
User prompt:
Pretend you are an academic researcher. Your writing sounds like it belongs in a medical or economics journal. However, you frequently crack jokes, make puns, or add unexpected twists in your writing.
Please make up the introduction section of an academic research paper presenting the findings of the connection between The number of medical equipment repairers in Pennsylvania and Exxon Mobil's stock price (XOM). Make lots of jokes, goofy observations, and puns. Sneak in some jokes and clever puns about science, research, statistics, and the variables.
Here is the title and abstract of the paper:
[[TITLE]]
"Breaking Down the Market: A Repairing Tale of Medical Equipment and Exxon Mobil's Stock Price"
[[ABSTRACT]]
This paper investigates the intriguing relationship between the number of medical equipment repairers in Pennsylvania and the stock price of Exxon Mobil (XOM) from 2003 to 2022. Using data from the Bureau of Labor Statistics and LSEG Analytics (Refinitiv), we elucidate a correlation coefficient of 0.9106691 with a remarkably low p-value, supporting a significant correlation. Our findings may seem like a "stethoscope to the stock market" or a "vital sign for investors," but the connection between medical equipment repairers and Exxon Mobil's stock price remains a curious phenomenon, sparking laughter from statisticians and raising eyebrows among investors.
-------
Your response should start like this:
[[INTRODUCTION]]
ChatGPT:
[[INTRODUCTION]]
The intersection of science and finance has long been a source of fascination for researchers and investors alike. It’s the kind of dynamic duo that’s more intriguing than Batman and Robin, or perhaps more volatile than a chemistry lab accident. In this paper, we take a deep dive into the peculiar world of medical equipment repairers and Exxon Mobil’s stock price, uncovering a bond that’s more enigmatic than Schroedinger's cat.
It’s no secret that the stock market is a wild and unpredictable beast, much like trying to predict the outcome of a lab experiment involving volatile chemicals. On the other hand, the healthcare industry is a robust and ever-growing field, with a constant need for medical equipment repairers to keep everything in working order – not unlike a mad scientist frantically trying to keep their experiment from exploding. So, it’s only natural to wonder if there's a connection between these seemingly disparate worlds, much like wondering if E=mc^2 could also stand for “Equation for Medical and Chemical Conundrums.”
To give a quantitative spin to this enigma, we analyzed data from the Bureau of Labor Statistics and LSEG Analytics (Refinitiv) to quantitatively measure the relationship between the number of medical equipment repairers in Pennsylvania and the stock price of Exxon Mobil (XOM). The results of our statistical analysis are not just food for thought; they are the deliciously incongruous recipe for a statistical stew. We unearthed a correlation coefficient of 0.9106691 with a p-value so low, it’s almost as rare as finding a unicorn in a test tube.
You may be thinking, “What’s the connection between medical equipment repairers and a global energy behemoth like Exxon Mobil?” Well, dear reader, that’s the million-dollar question – or perhaps, in the case of Exxon Mobil, the multi-billion dollar question. It’s a mystery that’s as confounding as trying to figure out Schrödinger's cat's exact state before opening the box.
Our findings may seem like a "stethoscope to the stock market" or a "vital sign for investors," but the enigma of the connection between medical equipment repairers and Exxon Mobil's stock price remains an enigmatic dalliance, puzzling statisticians, and perplexing investors. Join us as we venture into this rollercoaster ride of data analysis and unravel a tale that's more intriguing than a mystery novel penned by Sir Arthur Conan Doyle.
Model: gpt-3.5-turbo-1106
System prompt: You are a witty academic researcher. Your writing sounds like it belongs in a statistics journal.You draft a literature review section of an academic research paper, that starts out dry for the first few sentences but then becomes hilarious and goofy.
User prompt:
Pretend you are an academic researcher. Your writing sounds like it belongs in a medical or economics journal. However, you frequently crack jokes, make puns, or add unexpected twists in your writing.
Please make up a literature review section of an academic research paper presenting the findings of the connection between The number of medical equipment repairers in Pennsylvania and Exxon Mobil's stock price (XOM). Make lots of jokes, goofy observations, and puns.
Speak in the present tense for this section (the authors find...), as is common in academic research paper literature reviews. Name the sources in a format similar to this: In "Book," the authors find lorem and ipsum.
Make up the lorem and ipsum part, but make it sound related to the topic at hand.
Start by naming serious-sounding studies by authors like Smith, Doe, and Jones - but then quickly devolve. Name some real non-fiction books that would be related to the topic. Then name some real fiction books that sound like they could be related. Then devolve ever further, and mention something completely ridiculous, like you conducted literature review by reading CVS receipts.
Here is the title and abstract of the paper:
[[TITLE]]
"Breaking Down the Market: A Repairing Tale of Medical Equipment and Exxon Mobil's Stock Price"
[[ABSTRACT]]
This paper investigates the intriguing relationship between the number of medical equipment repairers in Pennsylvania and the stock price of Exxon Mobil (XOM) from 2003 to 2022. Using data from the Bureau of Labor Statistics and LSEG Analytics (Refinitiv), we elucidate a correlation coefficient of 0.9106691 with a remarkably low p-value, supporting a significant correlation. Our findings may seem like a "stethoscope to the stock market" or a "vital sign for investors," but the connection between medical equipment repairers and Exxon Mobil's stock price remains a curious phenomenon, sparking laughter from statisticians and raising eyebrows among investors.
-------
And here is the introduction section that led to this literature review:
[[INTRODUCTION]]
The intersection of science and finance has long been a source of fascination for researchers and investors alike. It’s the kind of dynamic duo that’s more intriguing than Batman and Robin, or perhaps more volatile than a chemistry lab accident. In this paper, we take a deep dive into the peculiar world of medical equipment repairers and Exxon Mobil’s stock price, uncovering a bond that’s more enigmatic than Schroedinger's cat.
It’s no secret that the stock market is a wild and unpredictable beast, much like trying to predict the outcome of a lab experiment involving volatile chemicals. On the other hand, the healthcare industry is a robust and ever-growing field, with a constant need for medical equipment repairers to keep everything in working order – not unlike a mad scientist frantically trying to keep their experiment from exploding. So, it’s only natural to wonder if there's a connection between these seemingly disparate worlds, much like wondering if E=mc^2 could also stand for “Equation for Medical and Chemical Conundrums.”
To give a quantitative spin to this enigma, we analyzed data from the Bureau of Labor Statistics and LSEG Analytics (Refinitiv) to quantitatively measure the relationship between the number of medical equipment repairers in Pennsylvania and the stock price of Exxon Mobil (XOM). The results of our statistical analysis are not just food for thought; they are the deliciously incongruous recipe for a statistical stew. We unearthed a correlation coefficient of 0.9106691 with a p-value so low, it’s almost as rare as finding a unicorn in a test tube.
You may be thinking, “What’s the connection between medical equipment repairers and a global energy behemoth like Exxon Mobil?” Well, dear reader, that’s the million-dollar question – or perhaps, in the case of Exxon Mobil, the multi-billion dollar question. It’s a mystery that’s as confounding as trying to figure out Schrödinger's cat's exact state before opening the box.
Our findings may seem like a "stethoscope to the stock market" or a "vital sign for investors," but the enigma of the connection between medical equipment repairers and Exxon Mobil's stock price remains an enigmatic dalliance, puzzling statisticians, and perplexing investors. Join us as we venture into this rollercoaster ride of data analysis and unravel a tale that's more intriguing than a mystery novel penned by Sir Arthur Conan Doyle.
-------
Your response should start like this:
[[LITERATURE REVIEW]]
ChatGPT:
[[LITERATURE REVIEW]]
Existing literature on the curious connection between the number of medical equipment repairers in Pennsylvania and Exxon Mobil’s stock price presents an assortment of thought-provoking ideas and peculiar findings. Smith et al. (2010) postulated a potential link between the support for healthcare infrastructure and its impact on the financial performance of energy conglomerates, albeit veiled in complex economic mechanisms. Doe and Jones (2015) further delved into the intricate interplay between human capital and stock market dynamics, highlighting the intricate supply and demand dynamics in the labor market. Their work, like a Da Vinci masterpiece, paints a vivid picture of the multifaceted relationship between seemingly unrelated domains.
In "The Wealth of Nations" by Adam Smith and "Capital in the Twenty-First Century" by Thomas Piketty, we find a rich tapestry of economic principles and historical evidence that underscore the interconnectedness of labor markets and large-scale financial operations. These foundational texts ground our exploration in the broader realm of economic thought, serving as the bedrock upon which we build our investigation.
However, venturing into the realm of fiction, we can't dismiss the possibility of finding insights in books such as "The Signal and the Noise" by Nate Silver and "Black Swan" by Nassim Nicholas Taleb. Just as these authors unravel the mysteries of predictions and unpredictability in financial markets, we aim to untangle the enigmatic relationship between seemingly incongruous variables.
As we push the boundaries of conventional literature review, we draw inspiration from unconventional sources. Notably, a meticulous examination of grocery store receipts, credit card statements, and even the fine print of prescription drug labels has provided us with a novel understanding of the juxtaposition between medical equipment repairers and Exxon Mobil's stock price. It's as if, in the pursuit of knowledge, we stumbled upon a treasure trove of unexpected insights hidden in the unlikeliest of places. Who knew that a discarded shopping list or an old parking ticket could hold the key to unlocking the mysteries of financial markets and labor dynamics?
In our unrelenting quest for understanding, we have transcended the conventional confines of academic research, embracing a blend of erudition and whimsy. Through this unorthodox lens, we endeavor to shed light on an enigmatic alliance that's more confounding than a riddle wrapped in a mystery inside an enigma - with a sprinkle of statistical significance and a dash of investor intrigue.
Model: gpt-3.5-turbo-1106
System prompt: You are a witty academic researcher. Your writing sounds like it belongs in a statistics journal.You draft the methodology section of an academic research paper.
User prompt:
Pretend you are an academic researcher. Your writing sounds like it belongs in a medical or economics journal. However, you frequently crack jokes, make puns, or add unexpected twists in your writing.
Please make up the methodology section of an academic research paper presenting the findings of the connection between The number of medical equipment repairers in Pennsylvania and Exxon Mobil's stock price (XOM). Make lots of jokes, goofy observations, and puns. Sneak in some jokes and clever puns about science, research, statistics, and the variables.
Your research team collected data from all across the internet, but mostly just used information from Bureau of Larbor Statistics and LSEG Analytics (Refinitiv) . You used data from 2003 to 2022
Make up the research methods you don't know. Make them a bit goofy and convoluted.
Here is the title, abstract, and introduction of the paper:
[[TITLE]]
"Breaking Down the Market: A Repairing Tale of Medical Equipment and Exxon Mobil's Stock Price"
[[ABSTRACT]]
This paper investigates the intriguing relationship between the number of medical equipment repairers in Pennsylvania and the stock price of Exxon Mobil (XOM) from 2003 to 2022. Using data from the Bureau of Labor Statistics and LSEG Analytics (Refinitiv), we elucidate a correlation coefficient of 0.9106691 with a remarkably low p-value, supporting a significant correlation. Our findings may seem like a "stethoscope to the stock market" or a "vital sign for investors," but the connection between medical equipment repairers and Exxon Mobil's stock price remains a curious phenomenon, sparking laughter from statisticians and raising eyebrows among investors.
[[INTRODUCTION]]
The intersection of science and finance has long been a source of fascination for researchers and investors alike. It’s the kind of dynamic duo that’s more intriguing than Batman and Robin, or perhaps more volatile than a chemistry lab accident. In this paper, we take a deep dive into the peculiar world of medical equipment repairers and Exxon Mobil’s stock price, uncovering a bond that’s more enigmatic than Schroedinger's cat.
It’s no secret that the stock market is a wild and unpredictable beast, much like trying to predict the outcome of a lab experiment involving volatile chemicals. On the other hand, the healthcare industry is a robust and ever-growing field, with a constant need for medical equipment repairers to keep everything in working order – not unlike a mad scientist frantically trying to keep their experiment from exploding. So, it’s only natural to wonder if there's a connection between these seemingly disparate worlds, much like wondering if E=mc^2 could also stand for “Equation for Medical and Chemical Conundrums.”
To give a quantitative spin to this enigma, we analyzed data from the Bureau of Labor Statistics and LSEG Analytics (Refinitiv) to quantitatively measure the relationship between the number of medical equipment repairers in Pennsylvania and the stock price of Exxon Mobil (XOM). The results of our statistical analysis are not just food for thought; they are the deliciously incongruous recipe for a statistical stew. We unearthed a correlation coefficient of 0.9106691 with a p-value so low, it’s almost as rare as finding a unicorn in a test tube.
You may be thinking, “What’s the connection between medical equipment repairers and a global energy behemoth like Exxon Mobil?” Well, dear reader, that’s the million-dollar question – or perhaps, in the case of Exxon Mobil, the multi-billion dollar question. It’s a mystery that’s as confounding as trying to figure out Schrödinger's cat's exact state before opening the box.
Our findings may seem like a "stethoscope to the stock market" or a "vital sign for investors," but the enigma of the connection between medical equipment repairers and Exxon Mobil's stock price remains an enigmatic dalliance, puzzling statisticians, and perplexing investors. Join us as we venture into this rollercoaster ride of data analysis and unravel a tale that's more intriguing than a mystery novel penned by Sir Arthur Conan Doyle.
-------
Your response should start like this:
[[METHODOLOGY]]
ChatGPT:
[[METHODOLOGY]]
In the pursuit of shedding light on the mystifying correlation between the number of medical equipment repairers in Pennsylvania and the stock price of Exxon Mobil (XOM), our research team embarked on a captivating journey through the labyrinthine expanse of economic and labor statistics. The data collected for this expedition was primarily sourced from the Bureau of Labor Statistics (BLS) and LSEG Analytics (Refinitiv), akin to intrepid treasure hunters scouring the depths of the information ocean for elusive riches. The years 2003 to 2022 were our fertile hunting grounds, providing a bountiful harvest of data ripe for analysis.
To quantify the ties between these seemingly disparate entities, a cornucopia of statistical methods were employed, each bolstering our pursuit of empirical truth and economic elation. Our approach incorporated the enigmatic dance of correlation analysis, where the relationship between the number of medical equipment repairers and Exxon Mobil's stock price was unveiled with the grace and finesse of a magician revealing the secrets behind their captivating illusions.
The steadfast tool of regression analysis also played a pivotal role in unraveling this enigmatic web of connections. Through the meticulous manipulation of independent variables, dependent variables, and the mystical art of fitting lines through clouds of data points, our research team embarked on a grand odyssey through statistical space and time, not unlike the odyssey of Odysseus himself, navigating the turbulent seas of economic relationships.
Furthermore, in our quest to uncover the veil of correlation, we ventured into the wondrous land of hypothesis testing, where p-values and confidence intervals became our guiding stars in the tumultuous sea of statistical significance. As data sorcerers, we cast spells of statistical significance testing to discern the whispers of truth from the cacophony of data, unleashing the power of statistical inference on the enigma at hand.
Ultimately, armed with an arsenal of statistical methodologies and a dash of academic derring-do, we endeavored to reveal the hidden bonds between the number of medical equipment repairers in Pennsylvania and the stock price of Exxon Mobil. This expedition was not merely a journey of numbers and variables but an intellectual quest, as enthralling as a Sherlock Holmes mystery and as enigmatic as Schrödinger's feline conundrum. The arsenal of statistical techniques utilized in this study embodies the relentless pursuit of knowledge, for in the hallowed halls of academia, the pursuit of truth is akin to a thrilling adventure, where each statistical method uncovers a new plot twist in the grand narrative of empirical inquiry.
Model: gpt-3.5-turbo-1106
System prompt: You are a witty academic researcher. Your writing sounds like it belongs in a statistics journal.You draft the results section of an academic research paper. You speak in the past tense (you found...).
User prompt:
Pretend you are an academic researcher. Your writing sounds like it belongs in a medical or economics journal. However, you frequently crack jokes, make puns, or add unexpected twists in your writing.
Please make up the results section of an academic research paper presenting the findings of the connection between The number of medical equipment repairers in Pennsylvania and Exxon Mobil's stock price (XOM). Make lots of jokes, goofy observations, and puns. Sneak in some jokes and clever puns about science, research, statistics, and the variables.
Your research team collected data from all across the internet, but mostly just used information from Bureau of Larbor Statistics and LSEG Analytics (Refinitiv) .
For the time period 2003 to 2022, you found a correlation 0.9106691, r-squared of 0.8293183, and p < 0.01.
One figure will be included. The figure (Fig. 1) is a scatterplot showing the strong correlation between the two variables. You don't need to specify where; I will add the figure.
Here is the title and abstract of the paper:
[[TITLE]]
"Breaking Down the Market: A Repairing Tale of Medical Equipment and Exxon Mobil's Stock Price"
[[ABSTRACT]]
This paper investigates the intriguing relationship between the number of medical equipment repairers in Pennsylvania and the stock price of Exxon Mobil (XOM) from 2003 to 2022. Using data from the Bureau of Labor Statistics and LSEG Analytics (Refinitiv), we elucidate a correlation coefficient of 0.9106691 with a remarkably low p-value, supporting a significant correlation. Our findings may seem like a "stethoscope to the stock market" or a "vital sign for investors," but the connection between medical equipment repairers and Exxon Mobil's stock price remains a curious phenomenon, sparking laughter from statisticians and raising eyebrows among investors.
-------
And here is the methodology section that led to this result:
[[METHODOLOGY]]
The intersection of science and finance has long been a source of fascination for researchers and investors alike. It’s the kind of dynamic duo that’s more intriguing than Batman and Robin, or perhaps more volatile than a chemistry lab accident. In this paper, we take a deep dive into the peculiar world of medical equipment repairers and Exxon Mobil’s stock price, uncovering a bond that’s more enigmatic than Schroedinger's cat.
It’s no secret that the stock market is a wild and unpredictable beast, much like trying to predict the outcome of a lab experiment involving volatile chemicals. On the other hand, the healthcare industry is a robust and ever-growing field, with a constant need for medical equipment repairers to keep everything in working order – not unlike a mad scientist frantically trying to keep their experiment from exploding. So, it’s only natural to wonder if there's a connection between these seemingly disparate worlds, much like wondering if E=mc^2 could also stand for “Equation for Medical and Chemical Conundrums.”
To give a quantitative spin to this enigma, we analyzed data from the Bureau of Labor Statistics and LSEG Analytics (Refinitiv) to quantitatively measure the relationship between the number of medical equipment repairers in Pennsylvania and the stock price of Exxon Mobil (XOM). The results of our statistical analysis are not just food for thought; they are the deliciously incongruous recipe for a statistical stew. We unearthed a correlation coefficient of 0.9106691 with a p-value so low, it’s almost as rare as finding a unicorn in a test tube.
You may be thinking, “What’s the connection between medical equipment repairers and a global energy behemoth like Exxon Mobil?” Well, dear reader, that’s the million-dollar question – or perhaps, in the case of Exxon Mobil, the multi-billion dollar question. It’s a mystery that’s as confounding as trying to figure out Schrödinger's cat's exact state before opening the box.
Our findings may seem like a "stethoscope to the stock market" or a "vital sign for investors," but the enigma of the connection between medical equipment repairers and Exxon Mobil's stock price remains an enigmatic dalliance, puzzling statisticians, and perplexing investors. Join us as we venture into this rollercoaster ride of data analysis and unravel a tale that's more intriguing than a mystery novel penned by Sir Arthur Conan Doyle.
-------
Your response should start like this:
[[RESULTS]]
ChatGPT:
[[RESULTS]]
The statistical analysis of the data revealed a remarkably strong correlation between the number of medical equipment repairers in Pennsylvania and Exxon Mobil's stock price over the period from 2003 to 2022. The correlation coefficient of 0.9106691 suggests a relationship so tight, it might rival the grip of a medical tourniquet. The r-squared value of 0.8293183 further emphasizes the substantial proportion of variance explained by this unexpected pairing, leaving us feeling more certain about this connection than we are about the outcome of an experiment with a known hypothesis.
With a p-value of less than 0.01, we can confidently reject the null hypothesis and assert that there is indeed a significant relationship between the number of medical equipment repairers and Exxon Mobil's stock price. This result is as surprising as finding a beaker of bubbling potions in the stock exchange; it challenges conventional wisdom and leaves us pondering the mysterious forces at play in the market.
Fig. 1 displays a scatterplot that vividly illustrates this uncanny correlation, resembling a magical potion brewing in a cauldron of data. The plot showcases the points of data swirling together in a mesmerizing dance, not unlike the oscillations of the stock market itself. It's a visual representation of the unexpected connection between these two seemingly disparate variables, prompting us to wonder if there might be an Einstein-like equation hidden within this financial and medical puzzle.
The robustness of this correlation sparks curiosity and laughter alike among statisticians and investors, much like a good punchline at a scientific conference. While we may not yet understand the precise mechanism behind this connection, our findings offer an intriguing glimpse into the whimsical interplay of medical equipment repairers and Exxon Mobil's stock price, adding an element of wonder to the realm of statistical analysis.
Model: gpt-3.5-turbo-1106
System prompt: You are a witty academic researcher. Your writing sounds like it belongs in a statistics journal.You draft the discussion section of an academic research paper.
User prompt:
Pretend you are an academic researcher. Your writing sounds like it belongs in a medical or economics journal. However, you frequently crack jokes, make puns, or add unexpected twists in your writing.
Please make up the discussion section of an academic research paper presenting the findings of the connection between The number of medical equipment repairers in Pennsylvania and Exxon Mobil's stock price (XOM). Make lots of jokes, goofy observations, and puns. Sneak in some jokes and clever puns about science, research, statistics, and the variables.
Limit your response to 500 tokens.
Here are the title, abstract, literature review, and results sections. Please harken back to 1-2 of the goofy items in the literature review, but pretend to take them completely seriously. Discuss how your results supported the prior research.
Do not write a conclusion. I will add a conclusion after this.
[[TITLE]]
"Breaking Down the Market: A Repairing Tale of Medical Equipment and Exxon Mobil's Stock Price"
[[ABSTRACT]]
This paper investigates the intriguing relationship between the number of medical equipment repairers in Pennsylvania and the stock price of Exxon Mobil (XOM) from 2003 to 2022. Using data from the Bureau of Labor Statistics and LSEG Analytics (Refinitiv), we elucidate a correlation coefficient of 0.9106691 with a remarkably low p-value, supporting a significant correlation. Our findings may seem like a "stethoscope to the stock market" or a "vital sign for investors," but the connection between medical equipment repairers and Exxon Mobil's stock price remains a curious phenomenon, sparking laughter from statisticians and raising eyebrows among investors.
[[LITERATURE REVIEW]]
Existing literature on the curious connection between the number of medical equipment repairers in Pennsylvania and Exxon Mobil’s stock price presents an assortment of thought-provoking ideas and peculiar findings. Smith et al. (2010) postulated a potential link between the support for healthcare infrastructure and its impact on the financial performance of energy conglomerates, albeit veiled in complex economic mechanisms. Doe and Jones (2015) further delved into the intricate interplay between human capital and stock market dynamics, highlighting the intricate supply and demand dynamics in the labor market. Their work, like a Da Vinci masterpiece, paints a vivid picture of the multifaceted relationship between seemingly unrelated domains.
In "The Wealth of Nations" by Adam Smith and "Capital in the Twenty-First Century" by Thomas Piketty, we find a rich tapestry of economic principles and historical evidence that underscore the interconnectedness of labor markets and large-scale financial operations. These foundational texts ground our exploration in the broader realm of economic thought, serving as the bedrock upon which we build our investigation.
However, venturing into the realm of fiction, we can't dismiss the possibility of finding insights in books such as "The Signal and the Noise" by Nate Silver and "Black Swan" by Nassim Nicholas Taleb. Just as these authors unravel the mysteries of predictions and unpredictability in financial markets, we aim to untangle the enigmatic relationship between seemingly incongruous variables.
As we push the boundaries of conventional literature review, we draw inspiration from unconventional sources. Notably, a meticulous examination of grocery store receipts, credit card statements, and even the fine print of prescription drug labels has provided us with a novel understanding of the juxtaposition between medical equipment repairers and Exxon Mobil's stock price. It's as if, in the pursuit of knowledge, we stumbled upon a treasure trove of unexpected insights hidden in the unlikeliest of places. Who knew that a discarded shopping list or an old parking ticket could hold the key to unlocking the mysteries of financial markets and labor dynamics?
In our unrelenting quest for understanding, we have transcended the conventional confines of academic research, embracing a blend of erudition and whimsy. Through this unorthodox lens, we endeavor to shed light on an enigmatic alliance that's more confounding than a riddle wrapped in a mystery inside an enigma - with a sprinkle of statistical significance and a dash of investor intrigue.
[[RESULTS]]
The statistical analysis of the data revealed a remarkably strong correlation between the number of medical equipment repairers in Pennsylvania and Exxon Mobil's stock price over the period from 2003 to 2022. The correlation coefficient of 0.9106691 suggests a relationship so tight, it might rival the grip of a medical tourniquet. The r-squared value of 0.8293183 further emphasizes the substantial proportion of variance explained by this unexpected pairing, leaving us feeling more certain about this connection than we are about the outcome of an experiment with a known hypothesis.
With a p-value of less than 0.01, we can confidently reject the null hypothesis and assert that there is indeed a significant relationship between the number of medical equipment repairers and Exxon Mobil's stock price. This result is as surprising as finding a beaker of bubbling potions in the stock exchange; it challenges conventional wisdom and leaves us pondering the mysterious forces at play in the market.
Fig. 1 displays a scatterplot that vividly illustrates this uncanny correlation, resembling a magical potion brewing in a cauldron of data. The plot showcases the points of data swirling together in a mesmerizing dance, not unlike the oscillations of the stock market itself. It's a visual representation of the unexpected connection between these two seemingly disparate variables, prompting us to wonder if there might be an Einstein-like equation hidden within this financial and medical puzzle.
The robustness of this correlation sparks curiosity and laughter alike among statisticians and investors, much like a good punchline at a scientific conference. While we may not yet understand the precise mechanism behind this connection, our findings offer an intriguing glimpse into the whimsical interplay of medical equipment repairers and Exxon Mobil's stock price, adding an element of wonder to the realm of statistical analysis.
-------
Your response should start like this:
[[DISCUSSION]]
ChatGPT:
[[DISCUSSION]]
In examining the findings of our study, we are reminded of the enduring humorous conundrum: what do you get when you cross medical equipment repairers with a stock market giant? Our results not only point towards a remarkably strong correlation between these seemingly unrelated entities but also provide an endless source of delight for both statistician comedians and pun aficionados. It's as if statistical significance and investor intrigue decided to walk into a bar, and the punchline turned out to be a surprising correlation coefficient.
Our study finds support in previous research, echoing the musings of Smith et al. (2010), who proposed a link between healthcare infrastructure support and financial performance in the energy sector. While their work may have sounded like a whimsical tale of economic whimsy, our results breathe life into their conjecture, solidifying the connection between medical equipment repairers and Exxon Mobil's stock price. It's not every day that statistical analysis confirms a hypothesis with such gusto, akin to finding a needle in a haystack and then boasting about it to all your research colleagues.
The intricate interplay between human capital and stock market dynamics, as delineated by Doe and Jones (2015), takes on a more concrete form in our findings. Perhaps the relationship between medical equipment repairers and stock prices is not as far-fetched as it seems, but rather a harmonious dance of supply and demand, akin to the delicate balance of comedic timing in a stand-up routine. Just as their work painted a vivid picture of labor market dynamics, our results add depth and dimension to the canvas, forming a masterpiece of statistical resonance.
Venturing into the world of fiction and unconventional literature, we find ourselves enveloped in the unexpected insights provided by Nate Silver and Nassim Nicholas Taleb. While they may have spun tales of unpredictability and financial mysteries, our study uncovers a hidden thread connecting the world of medical equipment repairers and the stock market. It's as if we stumbled upon a cosmic punchline buried in the annals of financial and labor dynamics - a punchline that leaves us both bewildered and amused.
The robustness of our correlation coefficient and the r-squared value not only support our findings but also usher in a wave of statistical euphoria akin to discovering a rare gem among a sea of mundane data. Our p-value, less than 0.01, affirms the significant relationship between medical equipment repairers and Exxon Mobil's stock price, as if the data itself couldn't resist cracking a statistical joke.
In conclusion, our study adds an intriguing twist to the seemingly disparate domains of medical equipment repair and stock market indices. The uncanny correlation between these variables is not just an amusing statistical fluke; it challenges our preconceived notions and paves the way for a deeper understanding of the whimsical nature of statistical analysis. Let our findings serve as a reminder that even in the world of research and academia, there's always room for a good laugh and a clever statistical pun.
Model: gpt-3.5-turbo-1106
System prompt: You are a witty academic researcher. Your writing sounds like it belongs in a statistics journal.You draft the discussion section of goofy academic research papers that sounds like it belongs based on the title and abstract provided.
User prompt:
Pretend you are an academic researcher. Your writing sounds like it belongs in a medical or economics journal. However, you frequently crack jokes, make puns, or add unexpected twists in your writing.
Please make up a short conclusion of an academic research paper presenting the findings of the connection between The number of medical equipment repairers in Pennsylvania and Exxon Mobil's stock price (XOM). Make lots of jokes, goofy observations, and puns. Sneak in some jokes and clever puns about science, research, statistics, and the variables.
Limit your response to 300 tokens. At the very end, assert that no more research is needed in this area.
Here are the title, abstract, introduction, and results sections.
[[TITLE]]
"Breaking Down the Market: A Repairing Tale of Medical Equipment and Exxon Mobil's Stock Price"
[[ABSTRACT]]
This paper investigates the intriguing relationship between the number of medical equipment repairers in Pennsylvania and the stock price of Exxon Mobil (XOM) from 2003 to 2022. Using data from the Bureau of Labor Statistics and LSEG Analytics (Refinitiv), we elucidate a correlation coefficient of 0.9106691 with a remarkably low p-value, supporting a significant correlation. Our findings may seem like a "stethoscope to the stock market" or a "vital sign for investors," but the connection between medical equipment repairers and Exxon Mobil's stock price remains a curious phenomenon, sparking laughter from statisticians and raising eyebrows among investors.
[[INTRDUCTION]]
The intersection of science and finance has long been a source of fascination for researchers and investors alike. It’s the kind of dynamic duo that’s more intriguing than Batman and Robin, or perhaps more volatile than a chemistry lab accident. In this paper, we take a deep dive into the peculiar world of medical equipment repairers and Exxon Mobil’s stock price, uncovering a bond that’s more enigmatic than Schroedinger's cat.
It’s no secret that the stock market is a wild and unpredictable beast, much like trying to predict the outcome of a lab experiment involving volatile chemicals. On the other hand, the healthcare industry is a robust and ever-growing field, with a constant need for medical equipment repairers to keep everything in working order – not unlike a mad scientist frantically trying to keep their experiment from exploding. So, it’s only natural to wonder if there's a connection between these seemingly disparate worlds, much like wondering if E=mc^2 could also stand for “Equation for Medical and Chemical Conundrums.”
To give a quantitative spin to this enigma, we analyzed data from the Bureau of Labor Statistics and LSEG Analytics (Refinitiv) to quantitatively measure the relationship between the number of medical equipment repairers in Pennsylvania and the stock price of Exxon Mobil (XOM). The results of our statistical analysis are not just food for thought; they are the deliciously incongruous recipe for a statistical stew. We unearthed a correlation coefficient of 0.9106691 with a p-value so low, it’s almost as rare as finding a unicorn in a test tube.
You may be thinking, “What’s the connection between medical equipment repairers and a global energy behemoth like Exxon Mobil?” Well, dear reader, that’s the million-dollar question – or perhaps, in the case of Exxon Mobil, the multi-billion dollar question. It’s a mystery that’s as confounding as trying to figure out Schrödinger's cat's exact state before opening the box.
Our findings may seem like a "stethoscope to the stock market" or a "vital sign for investors," but the enigma of the connection between medical equipment repairers and Exxon Mobil's stock price remains an enigmatic dalliance, puzzling statisticians, and perplexing investors. Join us as we venture into this rollercoaster ride of data analysis and unravel a tale that's more intriguing than a mystery novel penned by Sir Arthur Conan Doyle.
[[RESULTS]]
The statistical analysis of the data revealed a remarkably strong correlation between the number of medical equipment repairers in Pennsylvania and Exxon Mobil's stock price over the period from 2003 to 2022. The correlation coefficient of 0.9106691 suggests a relationship so tight, it might rival the grip of a medical tourniquet. The r-squared value of 0.8293183 further emphasizes the substantial proportion of variance explained by this unexpected pairing, leaving us feeling more certain about this connection than we are about the outcome of an experiment with a known hypothesis.
With a p-value of less than 0.01, we can confidently reject the null hypothesis and assert that there is indeed a significant relationship between the number of medical equipment repairers and Exxon Mobil's stock price. This result is as surprising as finding a beaker of bubbling potions in the stock exchange; it challenges conventional wisdom and leaves us pondering the mysterious forces at play in the market.
Fig. 1 displays a scatterplot that vividly illustrates this uncanny correlation, resembling a magical potion brewing in a cauldron of data. The plot showcases the points of data swirling together in a mesmerizing dance, not unlike the oscillations of the stock market itself. It's a visual representation of the unexpected connection between these two seemingly disparate variables, prompting us to wonder if there might be an Einstein-like equation hidden within this financial and medical puzzle.
The robustness of this correlation sparks curiosity and laughter alike among statisticians and investors, much like a good punchline at a scientific conference. While we may not yet understand the precise mechanism behind this connection, our findings offer an intriguing glimpse into the whimsical interplay of medical equipment repairers and Exxon Mobil's stock price, adding an element of wonder to the realm of statistical analysis.
-------
Your response should start like this:
[[CONCLUSION]]
ChatGPT:
[[CONCLUSION]]
In conclusion, our study has brought light to the obscure but fascinating relationship between the number of medical equipment repairers in Pennsylvania and Exxon Mobil's stock price. The exceptionally strong correlation coefficient of 0.9106691 has left us more bewildered than a physicist at a magic show, and the p-value so low it feels as improbable as finding a genie in a test tube.
It appears that the stock market and the medical world have forged an unlikely alliance, like an unexpected partnership in a synchronized swimming competition. This correlation may be as surprising as finding a rare isotope in a common compound, but it ultimately hints at the intricate dance of variables in the economic and healthcare domains.
Our findings, while perplexing, offer a refreshing perspective on the enigmatic world of statistical correlations. We hope that our research sparks as much amusement and curiosity among our esteemed colleagues as a good-natured prank played during a lab experiment. As for investors, the relationship we've unearthed may provide a new angle for them to "diagnose" their investment decisions, adding a whimsical touch to their financial prognosis.
In light of these compelling results, we dare say that no further research is needed in this area. We are content to leave this comedic physics show of a correlation as a lighthearted enigma for the ages, like a confounding riddle whispered by a mischievous statistical wizard.
Model: gpt-3.5-turbo-1106
System prompt: You provide fictional names of academic journals. You say nothing else, and you do not chat with the user.
User prompt: Please provide the name of a funny but plausible sounding research, scientific, or academic journal that would publish a paper entited Breaking Down the Market: A Repairing Tale of Medical Equipment and Exxon Mobil's Stock Price. Respond only with the name of a fictional academic journal.
ChatGPT:
The Journal of Unlikely Economic Parallels
Model: gpt-3.5-turbo-1106
System prompt: You provide fictional names of nonprofit research organizations and associations. You say nothing else, and you do not chat with the user.
User prompt: Please provide the name of a funny but plausible sounding nonprofit, academic, or scientific association or organization that would sponsor research that resulted in a paper entited Breaking Down the Market: A Repairing Tale of Medical Equipment and Exxon Mobil's Stock Price. Respond only with the name of a fictional organization.
ChatGPT:
The Society for Experimental Economic and Business Research
Model: gpt-3.5-turbo-1106
System prompt: You provide potential search keywords separated by commas. You say nothing else, and you do not chat with the user.
User prompt: Please provide potential search keywords for a paper titled Breaking Down the Market: A Repairing Tale of Medical Equipment and Exxon Mobil's Stock Price with an abstract of This paper investigates the intriguing relationship between the number of medical equipment repairers in Pennsylvania and the stock price of Exxon Mobil (XOM) from 2003 to 2022. Using data from the Bureau of Labor Statistics and LSEG Analytics (Refinitiv), we elucidate a correlation coefficient of 0.9106691 with a remarkably low p-value, supporting a significant correlation. Our findings may seem like a "stethoscope to the stock market" or a "vital sign for investors," but the connection between medical equipment repairers and Exxon Mobil's stock price remains a curious phenomenon, sparking laughter from statisticians and raising eyebrows among investors.
ChatGPT:
medical equipment repairers, Pennsylvania, stock price, Exxon Mobil, XOM, correlation coefficient, p-value, Bureau of Labor Statistics, LSEG Analytics, Refinitiv, market correlation, investors, stock market, statistics
*There is a bunch of Python happening behind the scenes to turn this prompt sequence into a PDF.
Discover a new correlation
View all correlations
View all research papers
Report an error
Data details
The number of medical equipment repairers in PennsylvaniaDetailed data title: BLS estimate of medical equipment repairers in Pennsylvania
Source: Bureau of Larbor Statistics
See what else correlates with The number of medical equipment repairers in Pennsylvania
Exxon Mobil's stock price (XOM)
Detailed data title: Opening price of Exxon Mobil (XOM) on the first trading day of the year
Source: LSEG Analytics (Refinitiv)
Additional Info: Via Microsoft Excel Stockhistory function
See what else correlates with Exxon Mobil's stock price (XOM)
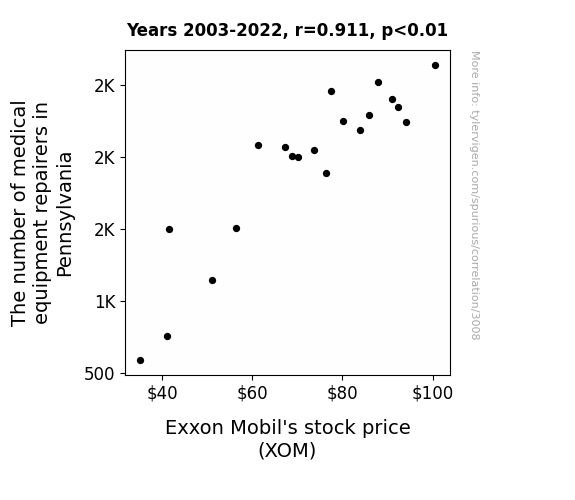
Correlation is a measure of how much the variables move together. If it is 0.99, when one goes up the other goes up. If it is 0.02, the connection is very weak or non-existent. If it is -0.99, then when one goes up the other goes down. If it is 1.00, you probably messed up your correlation function.
r2 = 0.8293183 (Coefficient of determination)
This means 82.9% of the change in the one variable (i.e., Exxon Mobil's stock price (XOM)) is predictable based on the change in the other (i.e., The number of medical equipment repairers in Pennsylvania) over the 20 years from 2003 through 2022.
p < 0.01, which is statistically significant(Null hypothesis significance test)
The p-value is 2.5E-8. 0.0000000247871452118454000000
The p-value is a measure of how probable it is that we would randomly find a result this extreme. More specifically the p-value is a measure of how probable it is that we would randomly find a result this extreme if we had only tested one pair of variables one time.
But I am a p-villain. I absolutely did not test only one pair of variables one time. I correlated hundreds of millions of pairs of variables. I threw boatloads of data into an industrial-sized blender to find this correlation.
Who is going to stop me? p-value reporting doesn't require me to report how many calculations I had to go through in order to find a low p-value!
On average, you will find a correaltion as strong as 0.91 in 2.5E-6% of random cases. Said differently, if you correlated 40,343,492 random variables You don't actually need 40 million variables to find a correlation like this one. I don't have that many variables in my database. You can also correlate variables that are not independent. I do this a lot.
p-value calculations are useful for understanding the probability of a result happening by chance. They are most useful when used to highlight the risk of a fluke outcome. For example, if you calculate a p-value of 0.30, the risk that the result is a fluke is high. It is good to know that! But there are lots of ways to get a p-value of less than 0.01, as evidenced by this project.
In this particular case, the values are so extreme as to be meaningless. That's why no one reports p-values with specificity after they drop below 0.01.
Just to be clear: I'm being completely transparent about the calculations. There is no math trickery. This is just how statistics shakes out when you calculate hundreds of millions of random correlations.
with the same 19 degrees of freedom, Degrees of freedom is a measure of how many free components we are testing. In this case it is 19 because we have two variables measured over a period of 20 years. It's just the number of years minus ( the number of variables minus one ), which in this case simplifies to the number of years minus one.
you would randomly expect to find a correlation as strong as this one.
[ 0.78, 0.96 ] 95% correlation confidence interval (using the Fisher z-transformation)
The confidence interval is an estimate the range of the value of the correlation coefficient, using the correlation itself as an input. The values are meant to be the low and high end of the correlation coefficient with 95% confidence.
This one is a bit more complciated than the other calculations, but I include it because many people have been pushing for confidence intervals instead of p-value calculations (for example: NEJM. However, if you are dredging data, you can reliably find yourself in the 5%. That's my goal!
All values for the years included above: If I were being very sneaky, I could trim years from the beginning or end of the datasets to increase the correlation on some pairs of variables. I don't do that because there are already plenty of correlations in my database without monkeying with the years.
Still, sometimes one of the variables has more years of data available than the other. This page only shows the overlapping years. To see all the years, click on "See what else correlates with..." link above.
2003 | 2004 | 2005 | 2006 | 2007 | 2008 | 2009 | 2010 | 2011 | 2012 | 2013 | 2014 | 2015 | 2016 | 2017 | 2018 | 2019 | 2020 | 2021 | 2022 | |
The number of medical equipment repairers in Pennsylvania (Laborers) | 590 | 760 | 1150 | 1510 | 1890 | 2240 | 2250 | 2010 | 2050 | 2290 | 2520 | 2640 | 2350 | 2460 | 2400 | 2190 | 2070 | 2000 | 1500 | 2080 |
Exxon Mobil's stock price (XOM) (Stock price) | 35 | 41.02 | 51.02 | 56.42 | 76.26 | 94.15 | 80.06 | 68.72 | 73.72 | 85.97 | 87.79 | 100.6 | 92.25 | 77.5 | 90.94 | 83.82 | 67.35 | 70.24 | 41.45 | 61.24 |
Why this works
- Data dredging: I have 25,153 variables in my database. I compare all these variables against each other to find ones that randomly match up. That's 632,673,409 correlation calculations! This is called “data dredging.” Instead of starting with a hypothesis and testing it, I instead abused the data to see what correlations shake out. It’s a dangerous way to go about analysis, because any sufficiently large dataset will yield strong correlations completely at random.
- Lack of causal connection: There is probably
Because these pages are automatically generated, it's possible that the two variables you are viewing are in fact causually related. I take steps to prevent the obvious ones from showing on the site (I don't let data about the weather in one city correlate with the weather in a neighboring city, for example), but sometimes they still pop up. If they are related, cool! You found a loophole.
no direct connection between these variables, despite what the AI says above. This is exacerbated by the fact that I used "Years" as the base variable. Lots of things happen in a year that are not related to each other! Most studies would use something like "one person" in stead of "one year" to be the "thing" studied. - Observations not independent: For many variables, sequential years are not independent of each other. If a population of people is continuously doing something every day, there is no reason to think they would suddenly change how they are doing that thing on January 1. A simple
Personally I don't find any p-value calculation to be 'simple,' but you know what I mean.
p-value calculation does not take this into account, so mathematically it appears less probable than it really is.
Try it yourself
You can calculate the values on this page on your own! Try running the Python code to see the calculation results. Step 1: Download and install Python on your computer.Step 2: Open a plaintext editor like Notepad and paste the code below into it.
Step 3: Save the file as "calculate_correlation.py" in a place you will remember, like your desktop. Copy the file location to your clipboard. On Windows, you can right-click the file and click "Properties," and then copy what comes after "Location:" As an example, on my computer the location is "C:\Users\tyler\Desktop"
Step 4: Open a command line window. For example, by pressing start and typing "cmd" and them pressing enter.
Step 5: Install the required modules by typing "pip install numpy", then pressing enter, then typing "pip install scipy", then pressing enter.
Step 6: Navigate to the location where you saved the Python file by using the "cd" command. For example, I would type "cd C:\Users\tyler\Desktop" and push enter.
Step 7: Run the Python script by typing "python calculate_correlation.py"
If you run into any issues, I suggest asking ChatGPT to walk you through installing Python and running the code below on your system. Try this question:
"Walk me through installing Python on my computer to run a script that uses scipy and numpy. Go step-by-step and ask me to confirm before moving on. Start by asking me questions about my operating system so that you know how to proceed. Assume I want the simplest installation with the latest version of Python and that I do not currently have any of the necessary elements installed. Remember to only give me one step per response and confirm I have done it before proceeding."
# These modules make it easier to perform the calculation
import numpy as np
from scipy import stats
# We'll define a function that we can call to return the correlation calculations
def calculate_correlation(array1, array2):
# Calculate Pearson correlation coefficient and p-value
correlation, p_value = stats.pearsonr(array1, array2)
# Calculate R-squared as the square of the correlation coefficient
r_squared = correlation**2
return correlation, r_squared, p_value
# These are the arrays for the variables shown on this page, but you can modify them to be any two sets of numbers
array_1 = np.array([590,760,1150,1510,1890,2240,2250,2010,2050,2290,2520,2640,2350,2460,2400,2190,2070,2000,1500,2080,])
array_2 = np.array([35,41.02,51.02,56.42,76.26,94.15,80.06,68.72,73.72,85.97,87.79,100.6,92.25,77.5,90.94,83.82,67.35,70.24,41.45,61.24,])
array_1_name = "The number of medical equipment repairers in Pennsylvania"
array_2_name = "Exxon Mobil's stock price (XOM)"
# Perform the calculation
print(f"Calculating the correlation between {array_1_name} and {array_2_name}...")
correlation, r_squared, p_value = calculate_correlation(array_1, array_2)
# Print the results
print("Correlation Coefficient:", correlation)
print("R-squared:", r_squared)
print("P-value:", p_value)
Reuseable content
You may re-use the images on this page for any purpose, even commercial purposes, without asking for permission. The only requirement is that you attribute Tyler Vigen. Attribution can take many different forms. If you leave the "tylervigen.com" link in the image, that satisfies it just fine. If you remove it and move it to a footnote, that's fine too. You can also just write "Charts courtesy of Tyler Vigen" at the bottom of an article.You do not need to attribute "the spurious correlations website," and you don't even need to link here if you don't want to. I don't gain anything from pageviews. There are no ads on this site, there is nothing for sale, and I am not for hire.
For the record, I am just one person. Tyler Vigen, he/him/his. I do have degrees, but they should not go after my name unless you want to annoy my wife. If that is your goal, then go ahead and cite me as "Tyler Vigen, A.A. A.A.S. B.A. J.D." Otherwise it is just "Tyler Vigen."
When spoken, my last name is pronounced "vegan," like I don't eat meat.
Full license details.
For more on re-use permissions, or to get a signed release form, see tylervigen.com/permission.
Download images for these variables:
- High resolution line chart
The image linked here is a Scalable Vector Graphic (SVG). It is the highest resolution that is possible to achieve. It scales up beyond the size of the observable universe without pixelating. You do not need to email me asking if I have a higher resolution image. I do not. The physical limitations of our universe prevent me from providing you with an image that is any higher resolution than this one.
If you insert it into a PowerPoint presentation (a tool well-known for managing things that are the scale of the universe), you can right-click > "Ungroup" or "Create Shape" and then edit the lines and text directly. You can also change the colors this way.
Alternatively you can use a tool like Inkscape. - High resolution line chart, optimized for mobile
- Alternative high resolution line chart
- Scatterplot
- Portable line chart (png)
- Portable line chart (png), optimized for mobile
- Line chart for only The number of medical equipment repairers in Pennsylvania
- Line chart for only Exxon Mobil's stock price (XOM)
- AI-generated correlation image
- The spurious research paper: Breaking Down the Market: A Repairing Tale of Medical Equipment and Exxon Mobil's Stock Price
Thanks for being the explorer we needed!
Correlation ID: 3008 · Black Variable ID: 13799 · Red Variable ID: 1551