Download png, svg
Check back later, or email me if you'd enjoy seeing this work in real-time.
Discover a new correlation
View all correlations
View all research papers
Report an error
Data details
The number of movies Willem Dafoe appeared inSource: The Movie DB
Additional Info: The Last Temptation of Christ (1988); The Boondock Saints (1999); Animal Factory (2000); Anamorph (2007); Light Sleeper (1992); The Loveless (1981); Off Limits (1988); Victory (1996); Tom & Viv (1994); Antichrist (2009); Go Go Tales (2007); White Sands (1992); Before It Had a Name (2005); The Dust of Time (2008); The Hunter (2011); 4:44 Last Day on Earth (2012); Roadhouse 66 (1984); The Night and the Moment (1995); Saving Norman (2013); The Smile Man (2013); Love's Routine (2013); Pavilion of Women (2001); The Procedure (2007); Triumph of the Spirit (1989); My Hindu Friend (2015); Pasolini (2014); Into the Deep: America, Whaling & The World (2010); Mind the Gap (2016); Fukushima: A Nuclear Story (2015); A Woman (2010); Siberia (2020); Mountain (2017); At Eternity's Gate (2018); Opus Zero (2018); O Panama (1985); Manou the Swift (2019); Piazza Vittorio (2017); Nelson Algren Live (2016); Tommaso (2020); Voom Portraits (2007); Togo (2019); Atalanta Strategy (1984); Bob Wilson's Life & Death of Marina Abramovic (2012); The Benaki Museum (2013); Location Production Footage: The Last Temptation of the Christ (1988); Sanctuary (2017); River (2021); Inside (2023); The Wild One (2023); Finding Satoshi (2022); Forrest Bess: Key to the Riddle (1999); Why Are We (Not) Creative? (2021); Spider-Man (2002); Mr. Bean's Holiday (2007); American Psycho (2000); Mississippi Burning (1988); Body of Evidence (1993); Shadow of the Vampire (2000); New Rose Hotel (1999); Fireflies in the Garden (2008); The Reckoning (2004); Clear and Present Danger (1994); Control (2004); To Live and Die in L.A. (1985); Auto Focus (2002); My Son, My Son, What Have Ye Done (2009); Bullfighter (2000); Edges of the Lord (2001); Adam Resurrected (2008); Flight of the Intruder (1991); A Most Wanted Man (2014); Rockets Redglare! (2003); Bad Country (2014); Globehunters: An Around the World in 80 Days Adventure (2000); Dog Eat Dog (2016); Father (2016); A Tour of the Inferno: Revisiting 'Platoon' (2001); Platoon (1986); The Lighthouse (2019); Babenco: Tell Me When I Die (2020); The Making of Daybreakers (2010); Roy's World: Barry Gifford's Chicago (2020); North on Evers (1992); Rhyme 'Em to Death (1993); Sportin' Life (2020); 7 Deaths of Maria Callas (2022); Dead for a Dollar (2022); Brothers in Arms (2018); Gonzo Girl (2023); Robert Wilson: The Beauty of the Mysterious (2022); The English Patient (1996); Wild at Heart (1990); Speed 2: Cruise Control (1997); Paris Je T'aime (2006); The Clearing (2004); xXx: State of the Union (2005); Miral (2010); Daybreakers (2010); Tomorrow You're Gone (2012); Odd Thomas (2013); Lulu on the Bridge (1998); What Happened to Monday (2017); The Florida Project (2017); Aquaman (2018); The Great Wall (2016); Abel/Asia (1998); Wim Wenders, Desperado (2020); Behind the Ultimate Spin: The Making of 'Spider-Man' (2002); Maresco / Pasolini (2021); SPYMASTER: John le Carré in Hamburg (2014); Poor Things (2023); Pet Shop Days (2023); Finding Nemo (2003); eXistenZ (1999); Manderlay (2005); Affliction (1998); American Dreamz (2006); John Carter (2012); Nymphomaniac: Vol. II (2013); A Family Man (2016); The Last Thing He Wanted (2020); Nightmare Alley (2021); The Card Counter (2021); Theatron. Romeo Castellucci (2018); The Lighthouse: A Dark & Stormy Tale (2020); The Making of A Most Wanted Man (2015); Inside Man (2006); The Life Aquatic with Steve Zissou (2004); Fantastic Mr. Fox (2009); Streets of Fire (1984); Out of the Furnace (2013); Matthew Gray Gubler's Life Aquatic Intern Journal (2005); John Wick (2014); New York Nights (1984); Death Note (2017); Julian Schnabel: A Private Portrait (2017); Motherless Brooklyn (2019); Final Cut: The Making and Unmaking of Heaven's Gate (2004); Spider-Mania (2002); Spider-Man: All Roads Lead to No Way Home (2022); Franco Battiato - La voce del padrone (2022); Dear America: Letters Home from Vietnam (1987); The Grand Budapest Hotel (2014); The Fault in Our Stars (2014); Murder on the Orient Express (2017); This Is an Adventure (2005); Counterfeit World: Making 'To Live and Die in L.A.' (2003); Cannes: All Access (2007); Vox Lux (2018); Abel Ferrara: Not Guilty (2003); John Wick: Calling in the Cavalry (2015); The Walker (2007); Ballad of a Righteous Merchant (2017); Under the Rainbow: Making The Florida Project (2018); Spider-Man: No Way Home (2021); Il coraggio di essere Franco (2022); White Homeland Commando (1993); Faraway, So Close! (1993); Farewell (2009); On the Set: 'The Life Aquatic with Steve Zissou' (2005); Brace Up! (1993); The Northman (2022); Ripley Under Ground (2005); Don't F*#% With John Wick (2015); Love, Antosha (2019); Once Upon a Time in Mexico (2003); Vincent van Gogh Superstar (2019); Basquiat (1996); Spider-Man 2 (2004); The Making of The Grand Budapest Hotel (2014); Born on the Fourth of July (1989); Cirque du Freak: The Vampire's Assistant (2009); Friedkin Uncut (2018); Asteroid City (2023); Spider-Man 3 (2007); The Hunger (1983); Cry-Baby (1990); The Aviator (2004); Zack Snyder's Justice League (2021); Creation of the Kingdom of Exodus (2022); Overnight (2003); Finding Dory (2016); The Boondock Saints II: All Saints Day (2009); The French Dispatch (2021); Celebrating Marvel's Stan Lee (2019); Jiminy Glick in Lalawood (2005); To Each His Own Cinema (2007); Heaven's Gate (1980); Final Cut: Ladies and Gentlemen (2012); Never Sleep Again: The Elm Street Legacy (2010)
See what else correlates with The number of movies Willem Dafoe appeared in
Biomass power generated in Australia
Detailed data title: Total biomass power generated in Australia in billion kWh
Source: Energy Information Administration
See what else correlates with Biomass power generated in Australia
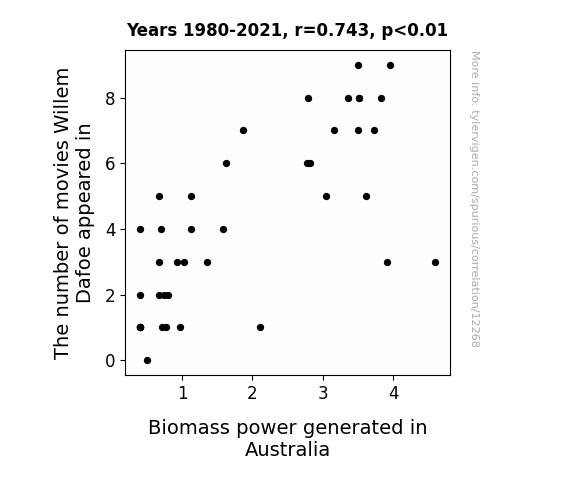
Correlation is a measure of how much the variables move together. If it is 0.99, when one goes up the other goes up. If it is 0.02, the connection is very weak or non-existent. If it is -0.99, then when one goes up the other goes down. If it is 1.00, you probably messed up your correlation function.
r2 = 0.5517558 (Coefficient of determination)
This means 55.2% of the change in the one variable (i.e., Biomass power generated in Australia) is predictable based on the change in the other (i.e., The number of movies Willem Dafoe appeared in) over the 42 years from 1980 through 2021.
p < 0.01, which is statistically significant(Null hypothesis significance test)
The p-value is 1.8E-8. 0.0000000177651594139968900000
The p-value is a measure of how probable it is that we would randomly find a result this extreme. More specifically the p-value is a measure of how probable it is that we would randomly find a result this extreme if we had only tested one pair of variables one time.
But I am a p-villain. I absolutely did not test only one pair of variables one time. I correlated hundreds of millions of pairs of variables. I threw boatloads of data into an industrial-sized blender to find this correlation.
Who is going to stop me? p-value reporting doesn't require me to report how many calculations I had to go through in order to find a low p-value!
On average, you will find a correaltion as strong as 0.74 in 1.8E-6% of random cases. Said differently, if you correlated 56,289,954 random variables You don't actually need 56 million variables to find a correlation like this one. I don't have that many variables in my database. You can also correlate variables that are not independent. I do this a lot.
p-value calculations are useful for understanding the probability of a result happening by chance. They are most useful when used to highlight the risk of a fluke outcome. For example, if you calculate a p-value of 0.30, the risk that the result is a fluke is high. It is good to know that! But there are lots of ways to get a p-value of less than 0.01, as evidenced by this project.
In this particular case, the values are so extreme as to be meaningless. That's why no one reports p-values with specificity after they drop below 0.01.
Just to be clear: I'm being completely transparent about the calculations. There is no math trickery. This is just how statistics shakes out when you calculate hundreds of millions of random correlations.
with the same 41 degrees of freedom, Degrees of freedom is a measure of how many free components we are testing. In this case it is 41 because we have two variables measured over a period of 42 years. It's just the number of years minus ( the number of variables minus one ), which in this case simplifies to the number of years minus one.
you would randomly expect to find a correlation as strong as this one.
[ 0.57, 0.85 ] 95% correlation confidence interval (using the Fisher z-transformation)
The confidence interval is an estimate the range of the value of the correlation coefficient, using the correlation itself as an input. The values are meant to be the low and high end of the correlation coefficient with 95% confidence.
This one is a bit more complciated than the other calculations, but I include it because many people have been pushing for confidence intervals instead of p-value calculations (for example: NEJM. However, if you are dredging data, you can reliably find yourself in the 5%. That's my goal!
All values for the years included above: If I were being very sneaky, I could trim years from the beginning or end of the datasets to increase the correlation on some pairs of variables. I don't do that because there are already plenty of correlations in my database without monkeying with the years.
Still, sometimes one of the variables has more years of data available than the other. This page only shows the overlapping years. To see all the years, click on "See what else correlates with..." link above.
1980 | 1981 | 1982 | 1983 | 1984 | 1985 | 1986 | 1987 | 1988 | 1989 | 1990 | 1991 | 1992 | 1993 | 1994 | 1995 | 1996 | 1997 | 1998 | 1999 | 2000 | 2001 | 2002 | 2003 | 2004 | 2005 | 2006 | 2007 | 2008 | 2009 | 2010 | 2011 | 2012 | 2013 | 2014 | 2015 | 2016 | 2017 | 2018 | 2019 | 2020 | 2021 | |
The number of movies Willem Dafoe appeared in (Movie appearances) | 1 | 1 | 0 | 1 | 4 | 2 | 1 | 1 | 4 | 2 | 2 | 1 | 3 | 5 | 2 | 1 | 3 | 1 | 3 | 4 | 5 | 3 | 4 | 6 | 7 | 8 | 3 | 9 | 3 | 6 | 6 | 1 | 5 | 7 | 8 | 5 | 7 | 9 | 8 | 7 | 8 | 8 |
Biomass power generated in Australia (Billion kWh) | 0.4 | 0.4 | 0.5 | 0.4 | 0.4 | 0.4 | 0.4 | 0.4 | 0.7 | 0.8 | 0.75 | 0.77 | 0.67 | 0.67 | 0.67 | 0.72 | 0.93 | 0.97 | 1.03 | 1.13 | 1.13 | 1.35 | 1.58 | 1.62 | 1.86 | 3.83 | 3.91 | 3.95 | 4.596 | 2.815 | 2.777 | 2.102 | 3.043 | 3.152 | 3.511 | 3.608 | 3.722 | 3.501 | 3.518 | 3.496 | 3.352 | 2.784 |
Why this works
- Data dredging: I have 25,237 variables in my database. I compare all these variables against each other to find ones that randomly match up. That's 636,906,169 correlation calculations! This is called “data dredging.” Instead of starting with a hypothesis and testing it, I instead abused the data to see what correlations shake out. It’s a dangerous way to go about analysis, because any sufficiently large dataset will yield strong correlations completely at random.
- Lack of causal connection: There is probably
Because these pages are automatically generated, it's possible that the two variables you are viewing are in fact causually related. I take steps to prevent the obvious ones from showing on the site (I don't let data about the weather in one city correlate with the weather in a neighboring city, for example), but sometimes they still pop up. If they are related, cool! You found a loophole.
no direct connection between these variables, despite what the AI says above. This is exacerbated by the fact that I used "Years" as the base variable. Lots of things happen in a year that are not related to each other! Most studies would use something like "one person" in stead of "one year" to be the "thing" studied. - Observations not independent: For many variables, sequential years are not independent of each other. If a population of people is continuously doing something every day, there is no reason to think they would suddenly change how they are doing that thing on January 1. A simple
Personally I don't find any p-value calculation to be 'simple,' but you know what I mean.
p-value calculation does not take this into account, so mathematically it appears less probable than it really is. - Outlandish outliers: There are "outliers" in this data.
In concept, "outlier" just means "way different than the rest of your dataset." When calculating a correlation like this, they are particularly impactful because a single outlier can substantially increase your correlation.
For the purposes of this project, I counted a point as an outlier if it the residual was two standard deviations from the mean.
(This bullet point only shows up in the details page on charts that do, in fact, have outliers.)
They stand out on the scatterplot above: notice the dots that are far away from any other dots. I intentionally mishandeled outliers, which makes the correlation look extra strong.
Try it yourself
You can calculate the values on this page on your own! Try running the Python code to see the calculation results. Step 1: Download and install Python on your computer.Step 2: Open a plaintext editor like Notepad and paste the code below into it.
Step 3: Save the file as "calculate_correlation.py" in a place you will remember, like your desktop. Copy the file location to your clipboard. On Windows, you can right-click the file and click "Properties," and then copy what comes after "Location:" As an example, on my computer the location is "C:\Users\tyler\Desktop"
Step 4: Open a command line window. For example, by pressing start and typing "cmd" and them pressing enter.
Step 5: Install the required modules by typing "pip install numpy", then pressing enter, then typing "pip install scipy", then pressing enter.
Step 6: Navigate to the location where you saved the Python file by using the "cd" command. For example, I would type "cd C:\Users\tyler\Desktop" and push enter.
Step 7: Run the Python script by typing "python calculate_correlation.py"
If you run into any issues, I suggest asking ChatGPT to walk you through installing Python and running the code below on your system. Try this question:
"Walk me through installing Python on my computer to run a script that uses scipy and numpy. Go step-by-step and ask me to confirm before moving on. Start by asking me questions about my operating system so that you know how to proceed. Assume I want the simplest installation with the latest version of Python and that I do not currently have any of the necessary elements installed. Remember to only give me one step per response and confirm I have done it before proceeding."
# These modules make it easier to perform the calculation
import numpy as np
from scipy import stats
# We'll define a function that we can call to return the correlation calculations
def calculate_correlation(array1, array2):
# Calculate Pearson correlation coefficient and p-value
correlation, p_value = stats.pearsonr(array1, array2)
# Calculate R-squared as the square of the correlation coefficient
r_squared = correlation**2
return correlation, r_squared, p_value
# These are the arrays for the variables shown on this page, but you can modify them to be any two sets of numbers
array_1 = np.array([1,1,0,1,4,2,1,1,4,2,2,1,3,5,2,1,3,1,3,4,5,3,4,6,7,8,3,9,3,6,6,1,5,7,8,5,7,9,8,7,8,8,])
array_2 = np.array([0.4,0.4,0.5,0.4,0.4,0.4,0.4,0.4,0.7,0.8,0.75,0.77,0.67,0.67,0.67,0.72,0.93,0.97,1.03,1.13,1.13,1.35,1.58,1.62,1.86,3.83,3.91,3.95,4.596,2.815,2.777,2.102,3.043,3.152,3.511,3.608,3.722,3.501,3.518,3.496,3.352,2.784,])
array_1_name = "The number of movies Willem Dafoe appeared in"
array_2_name = "Biomass power generated in Australia"
# Perform the calculation
print(f"Calculating the correlation between {array_1_name} and {array_2_name}...")
correlation, r_squared, p_value = calculate_correlation(array_1, array_2)
# Print the results
print("Correlation Coefficient:", correlation)
print("R-squared:", r_squared)
print("P-value:", p_value)
Reuseable content
You may re-use the images on this page for any purpose, even commercial purposes, without asking for permission. The only requirement is that you attribute Tyler Vigen. Attribution can take many different forms. If you leave the "tylervigen.com" link in the image, that satisfies it just fine. If you remove it and move it to a footnote, that's fine too. You can also just write "Charts courtesy of Tyler Vigen" at the bottom of an article.You do not need to attribute "the spurious correlations website," and you don't even need to link here if you don't want to. I don't gain anything from pageviews. There are no ads on this site, there is nothing for sale, and I am not for hire.
For the record, I am just one person. Tyler Vigen, he/him/his. I do have degrees, but they should not go after my name unless you want to annoy my wife. If that is your goal, then go ahead and cite me as "Tyler Vigen, A.A. A.A.S. B.A. J.D." Otherwise it is just "Tyler Vigen."
When spoken, my last name is pronounced "vegan," like I don't eat meat.
Full license details.
For more on re-use permissions, or to get a signed release form, see tylervigen.com/permission.
Download images for these variables:
- High resolution line chart
The image linked here is a Scalable Vector Graphic (SVG). It is the highest resolution that is possible to achieve. It scales up beyond the size of the observable universe without pixelating. You do not need to email me asking if I have a higher resolution image. I do not. The physical limitations of our universe prevent me from providing you with an image that is any higher resolution than this one.
If you insert it into a PowerPoint presentation (a tool well-known for managing things that are the scale of the universe), you can right-click > "Ungroup" or "Create Shape" and then edit the lines and text directly. You can also change the colors this way.
Alternatively you can use a tool like Inkscape. - High resolution line chart, optimized for mobile
- Alternative high resolution line chart
- Scatterplot
- Portable line chart (png)
- Portable line chart (png), optimized for mobile
- Line chart for only The number of movies Willem Dafoe appeared in
- Line chart for only Biomass power generated in Australia
Hats off to you for rating!
Correlation ID: 12268 · Black Variable ID: 26685 · Red Variable ID: 23474