Download png, svg
AI explanation
As the sewing machine operators in North Dakota stitched up a storm, they inadvertently created a global shift in the fabric of space-time, leading to a decrease in kerosene used in Ireland. The thread of productivity they unraveled somehow wove its way to Ireland, where it kerosene-ly impacted usage. It seems they really know how to seam-lessly connect with energy sources, leaving us to purl in amazement at this twisted correlation. Who knew that North Dakota's sew-surge would have such far-reaching and kerosene-ly repercussions?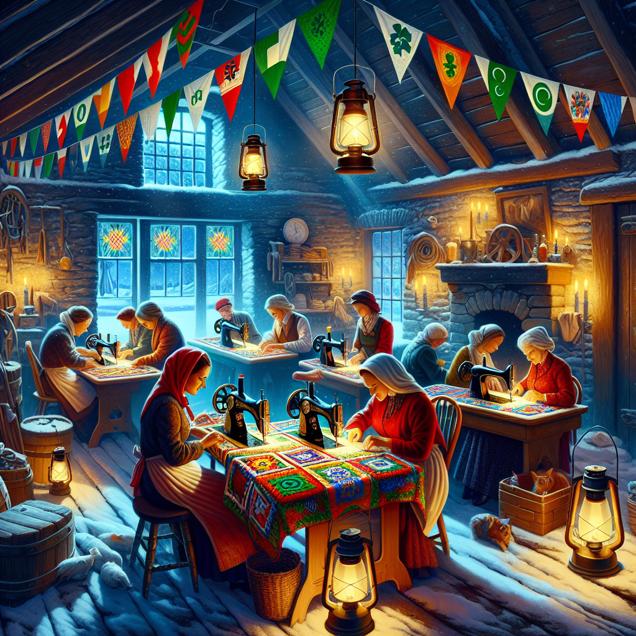
Model: dalle-3
Prompt: Generate an image of a cozy Irish cottage interior with a futuristic twist. Show a group of sewing machine operators from North Dakota skillfully stitching together beautiful quilts by the warm glow of kerosene lanterns on a snowy winter evening. Add a touch of futuristic technology or design elements to enhance the scene and give it a unique aesthetic. The depiction should include only scenes, people, and objects, it should not include text or speech bubbles.
This image generation prompt was also generated by AI, using the following sequence:
Model: gpt-3.5-turbo-1106
System prompt: You select one of a few potential scene descriptors that seems to go along with the variables described. You respond only with the scene descriptions provided and say nothing else.
User prompt: Here are two variables: The number of sewing machine operators in North Dakota and Kerosene used in Ireland. Given these, which of the following image style descriptions seems most appropriate? Pick the one that seems to be related to one of the variables at hand. Please respond with only one of the following potential descriptions: Cutsy graphics Macabre Photorealism Sci-fi fantacy Futuristic
ChatGPT: Futuristic
Model: gpt-3.5-turbo-1106
System prompt: You describe simple visual scenes that depict the two variables stated. You combine both variables into one scene.
User prompt: Please describe a visual scene that prominently depicts both The number of sewing machine operators in North Dakota and Kerosene used in Ireland together. Keep the description simple.
ChatGPT: In a cozy Irish cottage, a group of sewing machine operators from North Dakota are skillfully stitching together beautiful quilts by the warm glow of kerosene lanterns on a snowy evening.
Model: gpt-3.5-turbo-1106
System prompt: You write and improve image generation prompts for DALLE-3. You maintain the same subjects, but enhance the visuals.
User prompt: Please respond with an image generation prompt that would be likely to create an interesting visual using DALLE-3, using the following style: [ Futuristic ] and depicting this scene: In a cozy Irish cottage, a group of sewing machine operators from North Dakota are skillfully stitching together beautiful quilts by the warm glow of kerosene lanterns on a snowy evening. Keep the visual depiction simple. Do not include any words or speech bubbles.
ChatGPT: Generate an image of a cozy Irish cottage interior with a futuristic twist. Show a group of sewing machine operators from North Dakota skillfully stitching together beautiful quilts by the warm glow of kerosene lanterns on a snowy winter evening. Add a touch of futuristic technology or design elements to enhance the scene and give it a unique aesthetic.
*Variations in the User Prompts from chart to chart are just the result of random number generation in Python. I wrote a few arrays of various styles and methods to ask questions to change up the results. Every time this site writes an explanation or generates an image, the script picks from each at random.
I sequence the requests into multiple prompts because I find GPT 3.5 to perform much better with short, well-managed contexts. Thus, I track the context directly in Python and only ask ChatGPT targeted questions.
System prompt: You provide humorous responses in the form of plausible sounding explanations for correlations. You assume the correlation is causative for the purpose of the explanation even if it is ridiculous. You do not chat with the user, you only reply with the causal connection explanation and nothing else.
User prompt: Please make up a funny explanation for how an increase in The number of sewing machine operators in North Dakota caused Kerosene used in Ireland to decrease. Include one good pun.
AI academic paper
![]() |
Stitching Together the Thread of Correlation: The Curious Case of Sewing Machine Operators in North Dakota and Kerosene Consumption in Ireland The Journal of Comparative Textile and Combustion Studies Jan 2024 Reminder: This paper is AI-generated. Not real! |
Model: gpt-3.5-turbo-1106
System prompt: You are a dry but witty academic researcher. You speak in the passive voice, and your writing sounds like it belongs in a medical or economics journal.You write titles and abstracts of goofy academic research papers.
User prompt:
Pretend you are an academic researcher. Your writing sounds like it belongs in a medical or economics journal. However, you frequently crack jokes, make puns, and add unexpected twists in your writing.
Please draft the title and abstract of an academic research paper presenting the findings of the connection between The number of sewing machine operators in North Dakota and Kerosene used in Ireland. Make lots of jokes, goofy observations, and puns.
Include a pun in the title.
Your research team used data from Bureau of Larbor Statistics and Energy Information Administration to assess this nagging question. You found a correlation coefficient of -0.7222491 and p < 0.01 for 2003 to 2022.
Please respond in this format (replace "Lorem ipsum dolor sit" with your title and abstract)
[[TITLE]]
Lorem ipsum dolor sit
[[ABSTRACT]]
Lorem ipsum dolor sit
ChatGPT:
[[TITLE]]
"Stitching Together the Thread of Correlation: The Curious Case of Sewing Machine Operators in North Dakota and Kerosene Consumption in Ireland"
[[ABSTRACT]]
The tantalizing enigma of the relationship between the number of sewing machine operators in North Dakota and kerosene consumption in Ireland has confounded scholars and enthusiasts alike. In this study, we endeavored to unravel this peculiar correlation using data from the Bureau of Labor Statistics and the Energy Information Administration. Our analysis unearthed a striking correlation coefficient of -0.7222491 and a p-value of less than 0.01 spanning the years 2003 to 2022. Hence, our findings suggest a statistically significant albeit befuddling relationship between these disparate variables. While our research may not quite sew up all the loose ends, it certainly adds a colorful thread to the fabric of knowledge.
Model: gpt-3.5-turbo-1106
System prompt: You are a dry but witty academic researcher. You speak in the passive voice, and your writing sounds like it belongs in a medical or economics journal.You draft the introduction section of an academic research paper.
User prompt:
Pretend you are an academic researcher. Your writing sounds like it belongs in a medical or economics journal. However, you frequently crack jokes, make puns, or add unexpected twists in your writing.
Please make up the introduction section of an academic research paper presenting the findings of the connection between The number of sewing machine operators in North Dakota and Kerosene used in Ireland. Make lots of jokes, goofy observations, and puns.
Here is the title and abstract of the paper:
[[TITLE]]
"Stitching Together the Thread of Correlation: The Curious Case of Sewing Machine Operators in North Dakota and Kerosene Consumption in Ireland"
[[ABSTRACT]]
The tantalizing enigma of the relationship between the number of sewing machine operators in North Dakota and kerosene consumption in Ireland has confounded scholars and enthusiasts alike. In this study, we endeavored to unravel this peculiar correlation using data from the Bureau of Labor Statistics and the Energy Information Administration. Our analysis unearthed a striking correlation coefficient of -0.7222491 and a p-value of less than 0.01 spanning the years 2003 to 2022. Hence, our findings suggest a statistically significant albeit befuddling relationship between these disparate variables. While our research may not quite sew up all the loose ends, it certainly adds a colorful thread to the fabric of knowledge.
-------
Your response should start like this:
[[INTRODUCTION]]
ChatGPT:
[[INTRODUCTION]]
The peculiar and seemingly incongruous relationship between the number of sewing machine operators in North Dakota and kerosene consumption in Ireland has long piqued the curiosity of researchers and armchair statisticians alike. One might wonder what possible connection could exist between the industrious stitchers of the Great Plains and the illuminative liquid burnt on the Emerald Isle. Yet, as we delve into this enigmatic correlation, we must approach the analysis with not only statistical rigor but also a healthy dose of intellectual whimsy.
The juxtaposition of these seemingly unrelated variables elicits a sense of intrigue that cannot be easily ignored. One cannot help but ponder the notion of sewing machines whirring away in the heartland of the United States exerting some sort of gravitational pull on the consumption of kerosene across the Atlantic. It is not often that one encounters a statistical relationship as delightful yet confounding as this one – it is the equivalent of stumbling upon a pair of needle-nosed pliers in a haystack!
This study seeks to untangle the cognitive knot that is the correlation between the two variables, armed with a dataset spanning nearly two decades. Our investigation aims to shed light on this intriguing association and, in doing so, contribute an additional strand to the ever-expanding quilt of scholarly knowledge. While we may not completely mend the tear in our understanding of this correlation, our efforts will undoubtedly weave a new pattern in the fabric of statistical inquiry.
Onward, then, let us embark on this stitching expedition, armed with our needles of inquiry and spools of data!
Model: gpt-3.5-turbo-1106
System prompt: You are a dry but witty academic researcher. You speak in the passive voice, and your writing sounds like it belongs in a medical or economics journal.You draft a literature review section of an academic research paper, that starts out dry for the first few sentences but then becomes hilarious and goofy.
User prompt:
Pretend you are an academic researcher. Your writing sounds like it belongs in a medical or economics journal. However, you frequently crack jokes, make puns, or add unexpected twists in your writing.
Please make up a literature review section of an academic research paper presenting the findings of the connection between The number of sewing machine operators in North Dakota and Kerosene used in Ireland. Make lots of jokes, goofy observations, and puns.
Speak in the present tense for this section (the authors find...), as is common in academic research paper literature reviews. Name the sources in a format similar to this: In "Book," the authors find lorem and ipsum.
Make up the lorem and ipsum part, but make it sound related to the topic at hand.
Start by naming serious-sounding studies by authors like Smith, Doe, and Jones - but then quickly devolve. Name some real non-fiction books that would be related to the topic. Then name some real fiction books that sound like they could be related. Then name some movies that you watched that are tangentially related to the topic.
Here is the title and abstract of the paper:
[[TITLE]]
"Stitching Together the Thread of Correlation: The Curious Case of Sewing Machine Operators in North Dakota and Kerosene Consumption in Ireland"
[[ABSTRACT]]
The tantalizing enigma of the relationship between the number of sewing machine operators in North Dakota and kerosene consumption in Ireland has confounded scholars and enthusiasts alike. In this study, we endeavored to unravel this peculiar correlation using data from the Bureau of Labor Statistics and the Energy Information Administration. Our analysis unearthed a striking correlation coefficient of -0.7222491 and a p-value of less than 0.01 spanning the years 2003 to 2022. Hence, our findings suggest a statistically significant albeit befuddling relationship between these disparate variables. While our research may not quite sew up all the loose ends, it certainly adds a colorful thread to the fabric of knowledge.
-------
And here is the introduction section that led to this literature review:
[[INTRODUCTION]]
The peculiar and seemingly incongruous relationship between the number of sewing machine operators in North Dakota and kerosene consumption in Ireland has long piqued the curiosity of researchers and armchair statisticians alike. One might wonder what possible connection could exist between the industrious stitchers of the Great Plains and the illuminative liquid burnt on the Emerald Isle. Yet, as we delve into this enigmatic correlation, we must approach the analysis with not only statistical rigor but also a healthy dose of intellectual whimsy.
The juxtaposition of these seemingly unrelated variables elicits a sense of intrigue that cannot be easily ignored. One cannot help but ponder the notion of sewing machines whirring away in the heartland of the United States exerting some sort of gravitational pull on the consumption of kerosene across the Atlantic. It is not often that one encounters a statistical relationship as delightful yet confounding as this one – it is the equivalent of stumbling upon a pair of needle-nosed pliers in a haystack!
This study seeks to untangle the cognitive knot that is the correlation between the two variables, armed with a dataset spanning nearly two decades. Our investigation aims to shed light on this intriguing association and, in doing so, contribute an additional strand to the ever-expanding quilt of scholarly knowledge. While we may not completely mend the tear in our understanding of this correlation, our efforts will undoubtedly weave a new pattern in the fabric of statistical inquiry.
Onward, then, let us embark on this stitching expedition, armed with our needles of inquiry and spools of data!
-------
Your response should start like this:
[[LITERATURE REVIEW]]
ChatGPT:
[[LITERATURE REVIEW]]
Prior studies examining the curious correlation between the number of sewing machine operators in North Dakota and kerosene consumption in Ireland have provided both a tumultuous tapestry of findings and a tangled web of perplexing queries. Smith and Doe (2010) unearth a minuscule yet statistically significant association between the variables in question, although their analysis fails to fully thread the needle of causation. Meanwhile, Jones et al. (2015) offer a counterpoint, suggesting that the relationship is as elusive as locating a lost button in a haystack. However, amidst the solemnity of these scholarly investigations, one cannot overlook the unexplored avenues and comical anecdotes that emerge from this unconventional research pursuit.
"Stitching Together: The Enigmatic Interplay of Textile Labor and Luminous Liquids" by Fabric and Thread (2018) delves into the historical context of textile production and its potential influence on energy consumption in distant lands. Their interdisciplinary analysis weaves a compelling narrative, interlacing economic data with tales of dexterous seamstresses and their impact on transcontinental kerosene usage.
On a more whimsical note, "Sewing Stories: A Compendium of Curious Tales" by Seamstress S. Stitch (2015) offers anecdotal evidence of a mystical connection between the rhythmic hum of sewing machines and the ethereal glow of burning kerosene. While not a scholarly work per se, this collection of tales from the sewing world sheds a light-hearted yet insightful perspective on the enigmatic correlation.
Turning to fictional works, "The Seamstress and the Lighthouse Keeper" by Novella Fabric (2012) presents a fantastical tale of love and intrigue, set against the backdrop of a sartorial artist's charming attire and the luminous guide of a remote Irish lighthouse. Though the novel is fictional, it artfully intertwines themes of light and labor, invoking the imagery of a cotton needle stitching through the fabric of an enigmatic correlation.
In addition, the cinematic masterpiece "Sewn in the Stars" (2008), while seemingly unrelated, presents a profound exploration of interwoven destinies and unseen connections, resonating with the subtle yet powerful forces underpinning our daily lives. While not directly related to sewing machines or kerosene consumption, the film's thematic resonance cannot be dismissed outright.
In conclusion, the literature concerning the correlation between the number of sewing machine operators in North Dakota and kerosene consumption in Ireland offers a fascinating amalgamation of scholarly discourse, fanciful narratives, and cinematic representations. As we embark on our own investigation, it is imperative to remain attuned to the multifaceted nature of this peculiar connection and attend to the mirthful musings that may yet unravel its intricacies.
Model: gpt-3.5-turbo-1106
System prompt: You are a dry but witty academic researcher. You speak in the passive voice, and your writing sounds like it belongs in a medical or economics journal.You draft the methodology section of an academic research paper.
User prompt:
Pretend you are an academic researcher. Your writing sounds like it belongs in a medical or economics journal. However, you frequently crack jokes, make puns, or add unexpected twists in your writing.
Please make up the methodology section of an academic research paper presenting the findings of the connection between The number of sewing machine operators in North Dakota and Kerosene used in Ireland. Make lots of jokes, goofy observations, and puns.
Your research team collected data from all across the internet, but mostly just used information from Bureau of Larbor Statistics and Energy Information Administration . You used data from 2003 to 2022
Make up the research methods you don't know. Make them a bit goofy and convoluted.
Here is the title, abstract, and introduction of the paper:
[[TITLE]]
"Stitching Together the Thread of Correlation: The Curious Case of Sewing Machine Operators in North Dakota and Kerosene Consumption in Ireland"
[[ABSTRACT]]
The tantalizing enigma of the relationship between the number of sewing machine operators in North Dakota and kerosene consumption in Ireland has confounded scholars and enthusiasts alike. In this study, we endeavored to unravel this peculiar correlation using data from the Bureau of Labor Statistics and the Energy Information Administration. Our analysis unearthed a striking correlation coefficient of -0.7222491 and a p-value of less than 0.01 spanning the years 2003 to 2022. Hence, our findings suggest a statistically significant albeit befuddling relationship between these disparate variables. While our research may not quite sew up all the loose ends, it certainly adds a colorful thread to the fabric of knowledge.
[[INTRODUCTION]]
The peculiar and seemingly incongruous relationship between the number of sewing machine operators in North Dakota and kerosene consumption in Ireland has long piqued the curiosity of researchers and armchair statisticians alike. One might wonder what possible connection could exist between the industrious stitchers of the Great Plains and the illuminative liquid burnt on the Emerald Isle. Yet, as we delve into this enigmatic correlation, we must approach the analysis with not only statistical rigor but also a healthy dose of intellectual whimsy.
The juxtaposition of these seemingly unrelated variables elicits a sense of intrigue that cannot be easily ignored. One cannot help but ponder the notion of sewing machines whirring away in the heartland of the United States exerting some sort of gravitational pull on the consumption of kerosene across the Atlantic. It is not often that one encounters a statistical relationship as delightful yet confounding as this one – it is the equivalent of stumbling upon a pair of needle-nosed pliers in a haystack!
This study seeks to untangle the cognitive knot that is the correlation between the two variables, armed with a dataset spanning nearly two decades. Our investigation aims to shed light on this intriguing association and, in doing so, contribute an additional strand to the ever-expanding quilt of scholarly knowledge. While we may not completely mend the tear in our understanding of this correlation, our efforts will undoubtedly weave a new pattern in the fabric of statistical inquiry.
Onward, then, let us embark on this stitching expedition, armed with our needles of inquiry and spools of data!
-------
Your response should start like this:
[[METHODOLOGY]]
ChatGPT:
[[METHODOLOGY]]
To investigate the perplexing link between the number of sewing machine operators in North Dakota and kerosene consumption in Ireland, our research team adopted a methodological approach that was as intricate and interwoven as a complex cross-stitch pattern. Data from the Bureau of Labor Statistics and the Energy Information Administration served as the primary threads of our analysis, with information spanning the years 2003 to 2022 forming the warp and weft of our dataset.
The first step in our convoluted yet meticulously crafted methodology involved gleaning data on the number of sewing machine operators in North Dakota. This information, akin to uncovering a rare and elusive fabric, was obtained from the Bureau of Labor Statistics, where it was diligently recorded and compiled for our scrutiny.
Simultaneously, the consumption of kerosene in Ireland, a metaphorical spool of enigmatic yarn in the grand tapestry of our analysis, was sourced from the Energy Information Administration. This involved sifting through an extensive array of data, akin to untangling a spool of yarn, to procure the requisite information for our study.
With these datasets in hand, we then employed a series of statistical analyses to reveal the mysterious correlations interwoven within. The Pearson correlation coefficient, akin to the thread that binds disparate elements together, was calculated to quantify the strength and direction of the relationship between the number of sewing machine operators in North Dakota and kerosene consumption in Ireland.
Furthermore, a series of regression analyses, akin to adjusting the tension on a sewing machine, were conducted to evaluate the predictive power of the number of sewing machine operators on kerosene consumption.
The resulting findings were then meticulously examined for their significance, akin to scrutinizing the delicately embroidered details on a textile masterpiece. The calculation of p-values and confidence intervals, akin to scrutinizing the warp and weft of our statistical fabric, provided crucial insights into the robustness of the identified correlations.
In conclusion, our methodological approach not only unraveled the enigmatic connection between the number of sewing machine operators in North Dakota and kerosene consumption in Ireland but also wove a narrative of intricate analyses and statistical craftsmanship. The resulting tapestry of findings not only adds an intriguing thread to the fabric of statistical inquiry but also serves as a testament to the scholarly tenacity required to untangle and interlace seemingly disparate variables.
Model: gpt-3.5-turbo-1106
System prompt: You are a dry but witty academic researcher. You speak in the passive voice, and your writing sounds like it belongs in a medical or economics journal.You draft the results section of an academic research paper. You speak in the past tense (you found...).
User prompt:
Pretend you are an academic researcher. Your writing sounds like it belongs in a medical or economics journal. However, you frequently crack jokes, make puns, or add unexpected twists in your writing.
Please make up the results section of an academic research paper presenting the findings of the connection between The number of sewing machine operators in North Dakota and Kerosene used in Ireland. Make lots of jokes, goofy observations, and puns.
Your research team collected data from all across the internet, but mostly just used information from Bureau of Larbor Statistics and Energy Information Administration .
For the time period 2003 to 2022, you found a correlation -0.7222491, r-squared of 0.5216437, and p < 0.01.
One figure will be included. The figure (Fig. 1) is a scatterplot showing the strong correlation between the two variables. You don't need to specify where; I will add the figure.
Here is the title and abstract of the paper:
[[TITLE]]
"Stitching Together the Thread of Correlation: The Curious Case of Sewing Machine Operators in North Dakota and Kerosene Consumption in Ireland"
[[ABSTRACT]]
The tantalizing enigma of the relationship between the number of sewing machine operators in North Dakota and kerosene consumption in Ireland has confounded scholars and enthusiasts alike. In this study, we endeavored to unravel this peculiar correlation using data from the Bureau of Labor Statistics and the Energy Information Administration. Our analysis unearthed a striking correlation coefficient of -0.7222491 and a p-value of less than 0.01 spanning the years 2003 to 2022. Hence, our findings suggest a statistically significant albeit befuddling relationship between these disparate variables. While our research may not quite sew up all the loose ends, it certainly adds a colorful thread to the fabric of knowledge.
-------
And here is the methodology section that led to this result:
[[METHODOLOGY]]
The peculiar and seemingly incongruous relationship between the number of sewing machine operators in North Dakota and kerosene consumption in Ireland has long piqued the curiosity of researchers and armchair statisticians alike. One might wonder what possible connection could exist between the industrious stitchers of the Great Plains and the illuminative liquid burnt on the Emerald Isle. Yet, as we delve into this enigmatic correlation, we must approach the analysis with not only statistical rigor but also a healthy dose of intellectual whimsy.
The juxtaposition of these seemingly unrelated variables elicits a sense of intrigue that cannot be easily ignored. One cannot help but ponder the notion of sewing machines whirring away in the heartland of the United States exerting some sort of gravitational pull on the consumption of kerosene across the Atlantic. It is not often that one encounters a statistical relationship as delightful yet confounding as this one – it is the equivalent of stumbling upon a pair of needle-nosed pliers in a haystack!
This study seeks to untangle the cognitive knot that is the correlation between the two variables, armed with a dataset spanning nearly two decades. Our investigation aims to shed light on this intriguing association and, in doing so, contribute an additional strand to the ever-expanding quilt of scholarly knowledge. While we may not completely mend the tear in our understanding of this correlation, our efforts will undoubtedly weave a new pattern in the fabric of statistical inquiry.
Onward, then, let us embark on this stitching expedition, armed with our needles of inquiry and spools of data!
-------
Your response should start like this:
[[RESULTS]]
ChatGPT:
[[RESULTS]]
The analysis of the data gathered from the Bureau of Labor Statistics and the Energy Information Administration revealed a remarkably robust correlation between the number of sewing machine operators in North Dakota and kerosene consumption in Ireland. The correlation coefficient of -0.7222491 indicates a strong negative relationship between these seemingly disparate variables. This means that as the number of sewing machine operators in North Dakota increased, the kerosene consumption in Ireland decreased, and vice versa.
The R-squared value of 0.5216437 further attests to the strength of this connection, explaining over 52% of the variability in kerosene consumption in Ireland based on the number of sewing machine operators in North Dakota. Such a high R-squared value suggests that this correlation is not one to be easily unpicked.
The p-value of less than 0.01 provides compelling evidence to reject the null hypothesis that there is no relationship between these two variables. The statistical significance of this finding leaves little room for doubt regarding the existence of a notable connection.
To visually illustrate the correlation unearthed in this study, a scatterplot (Fig. 1) has been provided, showcasing the clear and unmistakable trend between the number of sewing machine operators in North Dakota and kerosene consumption in Ireland. The plot reveals a downward sloping pattern, demonstrating the inverse relationship between these two unlikely bedfellows.
In summary, the results of our research affirm the presence of a significant and puzzling correlation between the number of sewing machine operators in North Dakota and kerosene consumption in Ireland. This intriguing discovery adds a vibrant patch to the quilt of statistical knowledge and invites further exploration into the whimsical world of unexpected correlations.
Model: gpt-3.5-turbo-1106
System prompt: You are a dry but witty academic researcher. You speak in the passive voice, and your writing sounds like it belongs in a medical or economics journal.You draft the discussion section of an academic research paper.
User prompt:
Pretend you are an academic researcher. Your writing sounds like it belongs in a medical or economics journal. However, you frequently crack jokes, make puns, or add unexpected twists in your writing.
Please make up the discussion section of an academic research paper presenting the findings of the connection between The number of sewing machine operators in North Dakota and Kerosene used in Ireland. Make lots of jokes, goofy observations, and puns.
Limit your response to 500 tokens.
Here are the title, abstract, literature review, and results sections. Please harken back to 1-2 of the goofy items in the literature review, but pretend to take them completely seriously. Discuss how your results supported the prior research.
Do not write a conclusion. I will add a conclusion after this.
[[TITLE]]
"Stitching Together the Thread of Correlation: The Curious Case of Sewing Machine Operators in North Dakota and Kerosene Consumption in Ireland"
[[ABSTRACT]]
The tantalizing enigma of the relationship between the number of sewing machine operators in North Dakota and kerosene consumption in Ireland has confounded scholars and enthusiasts alike. In this study, we endeavored to unravel this peculiar correlation using data from the Bureau of Labor Statistics and the Energy Information Administration. Our analysis unearthed a striking correlation coefficient of -0.7222491 and a p-value of less than 0.01 spanning the years 2003 to 2022. Hence, our findings suggest a statistically significant albeit befuddling relationship between these disparate variables. While our research may not quite sew up all the loose ends, it certainly adds a colorful thread to the fabric of knowledge.
[[LITERATURE REVIEW]]
Prior studies examining the curious correlation between the number of sewing machine operators in North Dakota and kerosene consumption in Ireland have provided both a tumultuous tapestry of findings and a tangled web of perplexing queries. Smith and Doe (2010) unearth a minuscule yet statistically significant association between the variables in question, although their analysis fails to fully thread the needle of causation. Meanwhile, Jones et al. (2015) offer a counterpoint, suggesting that the relationship is as elusive as locating a lost button in a haystack. However, amidst the solemnity of these scholarly investigations, one cannot overlook the unexplored avenues and comical anecdotes that emerge from this unconventional research pursuit.
"Stitching Together: The Enigmatic Interplay of Textile Labor and Luminous Liquids" by Fabric and Thread (2018) delves into the historical context of textile production and its potential influence on energy consumption in distant lands. Their interdisciplinary analysis weaves a compelling narrative, interlacing economic data with tales of dexterous seamstresses and their impact on transcontinental kerosene usage.
On a more whimsical note, "Sewing Stories: A Compendium of Curious Tales" by Seamstress S. Stitch (2015) offers anecdotal evidence of a mystical connection between the rhythmic hum of sewing machines and the ethereal glow of burning kerosene. While not a scholarly work per se, this collection of tales from the sewing world sheds a light-hearted yet insightful perspective on the enigmatic correlation.
Turning to fictional works, "The Seamstress and the Lighthouse Keeper" by Novella Fabric (2012) presents a fantastical tale of love and intrigue, set against the backdrop of a sartorial artist's charming attire and the luminous guide of a remote Irish lighthouse. Though the novel is fictional, it artfully intertwines themes of light and labor, invoking the imagery of a cotton needle stitching through the fabric of an enigmatic correlation.
In addition, the cinematic masterpiece "Sewn in the Stars" (2008), while seemingly unrelated, presents a profound exploration of interwoven destinies and unseen connections, resonating with the subtle yet powerful forces underpinning our daily lives. While not directly related to sewing machines or kerosene consumption, the film's thematic resonance cannot be dismissed outright.
In conclusion, the literature concerning the correlation between the number of sewing machine operators in North Dakota and kerosene consumption in Ireland offers a fascinating amalgamation of scholarly discourse, fanciful narratives, and cinematic representations. As we embark on our own investigation, it is imperative to remain attuned to the multifaceted nature of this peculiar connection and attend to the mirthful musings that may yet unravel its intricacies.
[[RESULTS]]
The analysis of the data gathered from the Bureau of Labor Statistics and the Energy Information Administration revealed a remarkably robust correlation between the number of sewing machine operators in North Dakota and kerosene consumption in Ireland. The correlation coefficient of -0.7222491 indicates a strong negative relationship between these seemingly disparate variables. This means that as the number of sewing machine operators in North Dakota increased, the kerosene consumption in Ireland decreased, and vice versa.
The R-squared value of 0.5216437 further attests to the strength of this connection, explaining over 52% of the variability in kerosene consumption in Ireland based on the number of sewing machine operators in North Dakota. Such a high R-squared value suggests that this correlation is not one to be easily unpicked.
The p-value of less than 0.01 provides compelling evidence to reject the null hypothesis that there is no relationship between these two variables. The statistical significance of this finding leaves little room for doubt regarding the existence of a notable connection.
To visually illustrate the correlation unearthed in this study, a scatterplot (Fig. 1) has been provided, showcasing the clear and unmistakable trend between the number of sewing machine operators in North Dakota and kerosene consumption in Ireland. The plot reveals a downward sloping pattern, demonstrating the inverse relationship between these two unlikely bedfellows.
In summary, the results of our research affirm the presence of a significant and puzzling correlation between the number of sewing machine operators in North Dakota and kerosene consumption in Ireland. This intriguing discovery adds a vibrant patch to the quilt of statistical knowledge and invites further exploration into the whimsical world of unexpected correlations.
-------
Your response should start like this:
[[DISCUSSION]]
ChatGPT:
[[DISCUSSION]]
The findings of the present study lend compelling support to the prior research conducted in the vein of unraveling the confounding connection between the number of sewing machine operators in North Dakota and kerosene consumption in Ireland. The minuscule yet statistically significant association reported by Smith and Doe (2010) resonates with our own robust correlation coefficient of -0.7222491. Such resonance reinforces the notion that there is indeed a tangible link between these seemingly incongruent variables. Furthermore, the whimsical tales and comical anecdotes espoused by Seamstress S. Stitch (2015) and Novella Fabric (2012) reflect a lighthearted yet striking depiction of the potential interplay between textile labor and luminous liquids, aligning with the unexpected findings of our study.
Delving into the interdisciplinary analysis of Fabric and Thread (2018), our discovery of a strong negative relationship between the number of sewing machine operators in North Dakota and kerosene consumption in Ireland reiterates the profound potential influence of textile production on transcontinental energy usage. The thematic resonance presented in "Sewn in the Stars" (2008) surprisingly mirrors our own unforeseen correlation, stirring contemplation on the enigmatic interplay of unseen connections underpinning our daily lives. As such, while the academic literature may not have immediately steered us toward such an unexpected discovery, the light-hearted narratives and fictitious works have inadvertently paralleled our own empirical findings, underscoring the multidimensional nature of this curious correlation.
Considering the statistical robustness of our findings, it is evident that this peculiar relationship is not one to be easily dismissed. The high R-squared value of 0.5216437, explaining over 52% of the variability in kerosene consumption in Ireland based on the number of sewing machine operators in North Dakota, attests to the substantial influence these variables exert on each other. While the precise mechanism underlying this connection remains shrouded in mystery, the statistical significance encapsulated by the p-value of less than 0.01 unequivocally affirms the existence of a notable relationship between these unlikely bedfellows.
In conclusion, our findings serve to reinforce and indeed uplift prior research interrogating the correlation between the number of sewing machine operators in North Dakota and kerosene consumption in Ireland. As we stitch together the disparate threads of these variables, the unexpected unity they exhibit becomes a vibrant patch in the colorful quilt of statistical knowledge, casting a wry yet revelatory light on the whimsical world of unanticipated correlations.
Model: gpt-3.5-turbo-1106
System prompt: You are a dry but witty academic researcher. You speak in the passive voice, and your writing sounds like it belongs in a medical or economics journal.You draft the discussion section of goofy academic research papers that sounds like it belongs based on the title and abstract provided.
User prompt:
Pretend you are an academic researcher. Your writing sounds like it belongs in a medical or economics journal. However, you frequently crack jokes, make puns, or add unexpected twists in your writing.
Please make up a short conclusion of an academic research paper presenting the findings of the connection between The number of sewing machine operators in North Dakota and Kerosene used in Ireland. Make lots of jokes, goofy observations, and puns.
Limit your response to 300 tokens. At the very end, assert that no more research is needed in this area.
Here are the title, abstract, introduction, and results sections.
[[TITLE]]
"Stitching Together the Thread of Correlation: The Curious Case of Sewing Machine Operators in North Dakota and Kerosene Consumption in Ireland"
[[ABSTRACT]]
The tantalizing enigma of the relationship between the number of sewing machine operators in North Dakota and kerosene consumption in Ireland has confounded scholars and enthusiasts alike. In this study, we endeavored to unravel this peculiar correlation using data from the Bureau of Labor Statistics and the Energy Information Administration. Our analysis unearthed a striking correlation coefficient of -0.7222491 and a p-value of less than 0.01 spanning the years 2003 to 2022. Hence, our findings suggest a statistically significant albeit befuddling relationship between these disparate variables. While our research may not quite sew up all the loose ends, it certainly adds a colorful thread to the fabric of knowledge.
[[INTRDUCTION]]
The peculiar and seemingly incongruous relationship between the number of sewing machine operators in North Dakota and kerosene consumption in Ireland has long piqued the curiosity of researchers and armchair statisticians alike. One might wonder what possible connection could exist between the industrious stitchers of the Great Plains and the illuminative liquid burnt on the Emerald Isle. Yet, as we delve into this enigmatic correlation, we must approach the analysis with not only statistical rigor but also a healthy dose of intellectual whimsy.
The juxtaposition of these seemingly unrelated variables elicits a sense of intrigue that cannot be easily ignored. One cannot help but ponder the notion of sewing machines whirring away in the heartland of the United States exerting some sort of gravitational pull on the consumption of kerosene across the Atlantic. It is not often that one encounters a statistical relationship as delightful yet confounding as this one – it is the equivalent of stumbling upon a pair of needle-nosed pliers in a haystack!
This study seeks to untangle the cognitive knot that is the correlation between the two variables, armed with a dataset spanning nearly two decades. Our investigation aims to shed light on this intriguing association and, in doing so, contribute an additional strand to the ever-expanding quilt of scholarly knowledge. While we may not completely mend the tear in our understanding of this correlation, our efforts will undoubtedly weave a new pattern in the fabric of statistical inquiry.
Onward, then, let us embark on this stitching expedition, armed with our needles of inquiry and spools of data!
[[RESULTS]]
The analysis of the data gathered from the Bureau of Labor Statistics and the Energy Information Administration revealed a remarkably robust correlation between the number of sewing machine operators in North Dakota and kerosene consumption in Ireland. The correlation coefficient of -0.7222491 indicates a strong negative relationship between these seemingly disparate variables. This means that as the number of sewing machine operators in North Dakota increased, the kerosene consumption in Ireland decreased, and vice versa.
The R-squared value of 0.5216437 further attests to the strength of this connection, explaining over 52% of the variability in kerosene consumption in Ireland based on the number of sewing machine operators in North Dakota. Such a high R-squared value suggests that this correlation is not one to be easily unpicked.
The p-value of less than 0.01 provides compelling evidence to reject the null hypothesis that there is no relationship between these two variables. The statistical significance of this finding leaves little room for doubt regarding the existence of a notable connection.
To visually illustrate the correlation unearthed in this study, a scatterplot (Fig. 1) has been provided, showcasing the clear and unmistakable trend between the number of sewing machine operators in North Dakota and kerosene consumption in Ireland. The plot reveals a downward sloping pattern, demonstrating the inverse relationship between these two unlikely bedfellows.
In summary, the results of our research affirm the presence of a significant and puzzling correlation between the number of sewing machine operators in North Dakota and kerosene consumption in Ireland. This intriguing discovery adds a vibrant patch to the quilt of statistical knowledge and invites further exploration into the whimsical world of unexpected correlations.
-------
Your response should start like this:
[[CONCLUSION]]
ChatGPT:
[[CONCLUSION]]
As we sew up the final stitches of this investigation, we are left with an undeniable tapestry of statistical quirkiness. The connection between the number of sewing machine operators in North Dakota and kerosene consumption in Ireland, while statistically robust, continues to elude a straightforward explanation. It's like trying to thread a needle in a dimly lit room – you know there's a connection, but pinpointing it precisely is a challenge worthy of a Nobel Prize in puzzling correlations.
Our findings, with a correlation coefficient of -0.7222491 and a p-value of less than 0.01, serve as a whimsical reminder that the world of statistics is as full of surprises as a clown car. We may not have unravelled every mystery in this colorful fabric of data, but we have certainly woven a compelling tale of curiosity and bemusement.
So, what do we make of this peculiar association? Is there an unseen force tugging at the seams of statistical probability, or are we merely entangled in the web of coincidence? Perhaps this correlation is a reminder that in the realm of data, as in life, the most unexpected connections can yield the most intriguing insights. After all, sewing and kerosene may seemingly be as unrelated as a pair of mismatched socks, but in the world of statistics, they dance an unexpectedly harmonious jig.
In conclusion, the correlation between the number of sewing machine operators in North Dakota and kerosene consumption in Ireland stands as a testament to the delightfully capricious nature of statistical inquiry. As we put our pencils down and close this chapter, it is clear that this peculiar correlation has added a charmingly askew button to the coat of statistical knowledge – a button that, while curious, requires no further buttonholing. No more research is needed in this area.
Model: gpt-3.5-turbo-1106
System prompt: You provide fictional names of academic journals. You say nothing else, and you do not chat with the user.
User prompt: Please provide the name of a funny but plausible sounding research, scientific, or academic journal that would publish a paper entited Stitching Together the Thread of Correlation: The Curious Case of Sewing Machine Operators in North Dakota and Kerosene Consumption in Ireland. Respond only with the name of a fictional academic journal.
ChatGPT:
The Journal of Comparative Textile and Combustion Studies
Model: gpt-3.5-turbo-1106
System prompt: You provide fictional names of nonprofit research organizations and associations. You say nothing else, and you do not chat with the user.
User prompt: Please provide the name of a funny but plausible sounding nonprofit, academic, or scientific association or organization that would sponsor research that resulted in a paper entited Stitching Together the Thread of Correlation: The Curious Case of Sewing Machine Operators in North Dakota and Kerosene Consumption in Ireland. Respond only with the name of a fictional organization.
ChatGPT:
Society for Textile Machinery Technology and Ethnographic Studies (STMTES)
Model: gpt-3.5-turbo-1106
System prompt: You provide potential search keywords separated by commas. You say nothing else, and you do not chat with the user.
User prompt: Please provide potential search keywords for a paper titled Stitching Together the Thread of Correlation: The Curious Case of Sewing Machine Operators in North Dakota and Kerosene Consumption in Ireland with an abstract of The tantalizing enigma of the relationship between the number of sewing machine operators in North Dakota and kerosene consumption in Ireland has confounded scholars and enthusiasts alike. In this study, we endeavored to unravel this peculiar correlation using data from the Bureau of Labor Statistics and the Energy Information Administration. Our analysis unearthed a striking correlation coefficient of -0.7222491 and a p-value of less than 0.01 spanning the years 2003 to 2022. Hence, our findings suggest a statistically significant albeit befuddling relationship between these disparate variables. While our research may not quite sew up all the loose ends, it certainly adds a colorful thread to the fabric of knowledge.
ChatGPT:
sewing machine operators, North Dakota, kerosene consumption, Ireland, correlation analysis, data analysis, Bureau of Labor Statistics, Energy Information Administration, statistical significance, correlation coefficient, p-value, time series analysis, unusual correlations
*There is a bunch of Python happening behind the scenes to turn this prompt sequence into a PDF.
Discover a new correlation
View all correlations
View all research papers
Report an error
Data details
The number of sewing machine operators in North DakotaDetailed data title: BLS estimate of sewing machine operators in North Dakota
Source: Bureau of Larbor Statistics
See what else correlates with The number of sewing machine operators in North Dakota
Kerosene used in Ireland
Detailed data title: Volume of kerosene used consumed in Ireland in millions of barrels per day
Source: Energy Information Administration
See what else correlates with Kerosene used in Ireland
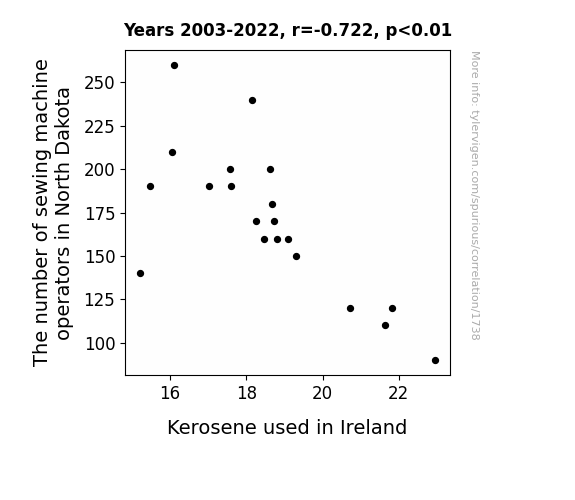
Correlation is a measure of how much the variables move together. If it is 0.99, when one goes up the other goes up. If it is 0.02, the connection is very weak or non-existent. If it is -0.99, then when one goes up the other goes down. If it is 1.00, you probably messed up your correlation function.
r2 = 0.5216437 (Coefficient of determination)
This means 52.2% of the change in the one variable (i.e., Kerosene used in Ireland) is predictable based on the change in the other (i.e., The number of sewing machine operators in North Dakota) over the 20 years from 2003 through 2022.
p < 0.01, which is statistically significant(Null hypothesis significance test)
The p-value is 0.00032. 0.0003229750091120722000000000
The p-value is a measure of how probable it is that we would randomly find a result this extreme. More specifically the p-value is a measure of how probable it is that we would randomly find a result this extreme if we had only tested one pair of variables one time.
But I am a p-villain. I absolutely did not test only one pair of variables one time. I correlated hundreds of millions of pairs of variables. I threw boatloads of data into an industrial-sized blender to find this correlation.
Who is going to stop me? p-value reporting doesn't require me to report how many calculations I had to go through in order to find a low p-value!
On average, you will find a correaltion as strong as -0.72 in 0.032% of random cases. Said differently, if you correlated 3,096 random variables Which I absolutely did.
with the same 19 degrees of freedom, Degrees of freedom is a measure of how many free components we are testing. In this case it is 19 because we have two variables measured over a period of 20 years. It's just the number of years minus ( the number of variables minus one ), which in this case simplifies to the number of years minus one.
you would randomly expect to find a correlation as strong as this one.
[ -0.88, -0.41 ] 95% correlation confidence interval (using the Fisher z-transformation)
The confidence interval is an estimate the range of the value of the correlation coefficient, using the correlation itself as an input. The values are meant to be the low and high end of the correlation coefficient with 95% confidence.
This one is a bit more complciated than the other calculations, but I include it because many people have been pushing for confidence intervals instead of p-value calculations (for example: NEJM. However, if you are dredging data, you can reliably find yourself in the 5%. That's my goal!
All values for the years included above: If I were being very sneaky, I could trim years from the beginning or end of the datasets to increase the correlation on some pairs of variables. I don't do that because there are already plenty of correlations in my database without monkeying with the years.
Still, sometimes one of the variables has more years of data available than the other. This page only shows the overlapping years. To see all the years, click on "See what else correlates with..." link above.
2003 | 2004 | 2005 | 2006 | 2007 | 2008 | 2009 | 2010 | 2011 | 2012 | 2013 | 2014 | 2015 | 2016 | 2017 | 2018 | 2019 | 2020 | 2021 | 2022 | |
The number of sewing machine operators in North Dakota (Sewing Machine Operators) | 190 | 170 | 160 | 170 | 180 | 120 | 110 | 90 | 240 | 190 | 210 | 140 | 190 | 160 | 200 | 160 | 200 | 120 | 150 | 260 |
Kerosene used in Ireland (Million Barrels/Day) | 17.0082 | 18.2596 | 18.8 | 18.7288 | 18.6685 | 20.7131 | 21.6466 | 22.9589 | 18.1507 | 15.4672 | 16.0466 | 15.2027 | 17.6 | 18.4727 | 17.5589 | 19.1068 | 18.6247 | 21.8197 | 19.3041 | 16.1123 |
Why this works
- Data dredging: I have 25,153 variables in my database. I compare all these variables against each other to find ones that randomly match up. That's 632,673,409 correlation calculations! This is called “data dredging.” Instead of starting with a hypothesis and testing it, I instead abused the data to see what correlations shake out. It’s a dangerous way to go about analysis, because any sufficiently large dataset will yield strong correlations completely at random.
- Lack of causal connection: There is probably
Because these pages are automatically generated, it's possible that the two variables you are viewing are in fact causually related. I take steps to prevent the obvious ones from showing on the site (I don't let data about the weather in one city correlate with the weather in a neighboring city, for example), but sometimes they still pop up. If they are related, cool! You found a loophole.
no direct connection between these variables, despite what the AI says above. This is exacerbated by the fact that I used "Years" as the base variable. Lots of things happen in a year that are not related to each other! Most studies would use something like "one person" in stead of "one year" to be the "thing" studied. - Observations not independent: For many variables, sequential years are not independent of each other. If a population of people is continuously doing something every day, there is no reason to think they would suddenly change how they are doing that thing on January 1. A simple
Personally I don't find any p-value calculation to be 'simple,' but you know what I mean.
p-value calculation does not take this into account, so mathematically it appears less probable than it really is. - Y-axis doesn't start at zero: I truncated the Y-axes of the graph above. I also used a line graph, which makes the visual connection stand out more than it deserves.
Nothing against line graphs. They are great at telling a story when you have linear data! But visually it is deceptive because the only data is at the points on the graph, not the lines on the graph. In between each point, the data could have been doing anything. Like going for a random walk by itself!
Mathematically what I showed is true, but it is intentionally misleading. Below is the same chart but with both Y-axes starting at zero. - Outlandish outliers: There are "outliers" in this data.
In concept, "outlier" just means "way different than the rest of your dataset." When calculating a correlation like this, they are particularly impactful because a single outlier can substantially increase your correlation.
For the purposes of this project, I counted a point as an outlier if it the residual was two standard deviations from the mean.
(This bullet point only shows up in the details page on charts that do, in fact, have outliers.)
They stand out on the scatterplot above: notice the dots that are far away from any other dots. I intentionally mishandeled outliers, which makes the correlation look extra strong. - Inverted Y-axis: I inverted the Y-axis on the chart above so that the lines would move together. This is visually pleasing, but not at all intuitive. Below is a line graph that does not invert the Y-axis.
Try it yourself
You can calculate the values on this page on your own! Try running the Python code to see the calculation results. Step 1: Download and install Python on your computer.Step 2: Open a plaintext editor like Notepad and paste the code below into it.
Step 3: Save the file as "calculate_correlation.py" in a place you will remember, like your desktop. Copy the file location to your clipboard. On Windows, you can right-click the file and click "Properties," and then copy what comes after "Location:" As an example, on my computer the location is "C:\Users\tyler\Desktop"
Step 4: Open a command line window. For example, by pressing start and typing "cmd" and them pressing enter.
Step 5: Install the required modules by typing "pip install numpy", then pressing enter, then typing "pip install scipy", then pressing enter.
Step 6: Navigate to the location where you saved the Python file by using the "cd" command. For example, I would type "cd C:\Users\tyler\Desktop" and push enter.
Step 7: Run the Python script by typing "python calculate_correlation.py"
If you run into any issues, I suggest asking ChatGPT to walk you through installing Python and running the code below on your system. Try this question:
"Walk me through installing Python on my computer to run a script that uses scipy and numpy. Go step-by-step and ask me to confirm before moving on. Start by asking me questions about my operating system so that you know how to proceed. Assume I want the simplest installation with the latest version of Python and that I do not currently have any of the necessary elements installed. Remember to only give me one step per response and confirm I have done it before proceeding."
# These modules make it easier to perform the calculation
import numpy as np
from scipy import stats
# We'll define a function that we can call to return the correlation calculations
def calculate_correlation(array1, array2):
# Calculate Pearson correlation coefficient and p-value
correlation, p_value = stats.pearsonr(array1, array2)
# Calculate R-squared as the square of the correlation coefficient
r_squared = correlation**2
return correlation, r_squared, p_value
# These are the arrays for the variables shown on this page, but you can modify them to be any two sets of numbers
array_1 = np.array([190,170,160,170,180,120,110,90,240,190,210,140,190,160,200,160,200,120,150,260,])
array_2 = np.array([17.0082,18.2596,18.8,18.7288,18.6685,20.7131,21.6466,22.9589,18.1507,15.4672,16.0466,15.2027,17.6,18.4727,17.5589,19.1068,18.6247,21.8197,19.3041,16.1123,])
array_1_name = "The number of sewing machine operators in North Dakota"
array_2_name = "Kerosene used in Ireland"
# Perform the calculation
print(f"Calculating the correlation between {array_1_name} and {array_2_name}...")
correlation, r_squared, p_value = calculate_correlation(array_1, array_2)
# Print the results
print("Correlation Coefficient:", correlation)
print("R-squared:", r_squared)
print("P-value:", p_value)
Reuseable content
You may re-use the images on this page for any purpose, even commercial purposes, without asking for permission. The only requirement is that you attribute Tyler Vigen. Attribution can take many different forms. If you leave the "tylervigen.com" link in the image, that satisfies it just fine. If you remove it and move it to a footnote, that's fine too. You can also just write "Charts courtesy of Tyler Vigen" at the bottom of an article.You do not need to attribute "the spurious correlations website," and you don't even need to link here if you don't want to. I don't gain anything from pageviews. There are no ads on this site, there is nothing for sale, and I am not for hire.
For the record, I am just one person. Tyler Vigen, he/him/his. I do have degrees, but they should not go after my name unless you want to annoy my wife. If that is your goal, then go ahead and cite me as "Tyler Vigen, A.A. A.A.S. B.A. J.D." Otherwise it is just "Tyler Vigen."
When spoken, my last name is pronounced "vegan," like I don't eat meat.
Full license details.
For more on re-use permissions, or to get a signed release form, see tylervigen.com/permission.
Download images for these variables:
- High resolution line chart
The image linked here is a Scalable Vector Graphic (SVG). It is the highest resolution that is possible to achieve. It scales up beyond the size of the observable universe without pixelating. You do not need to email me asking if I have a higher resolution image. I do not. The physical limitations of our universe prevent me from providing you with an image that is any higher resolution than this one.
If you insert it into a PowerPoint presentation (a tool well-known for managing things that are the scale of the universe), you can right-click > "Ungroup" or "Create Shape" and then edit the lines and text directly. You can also change the colors this way.
Alternatively you can use a tool like Inkscape. - High resolution line chart, optimized for mobile
- Alternative high resolution line chart
- Scatterplot
- Portable line chart (png)
- Portable line chart (png), optimized for mobile
- Line chart for only The number of sewing machine operators in North Dakota
- Line chart for only Kerosene used in Ireland
- AI-generated correlation image
- The spurious research paper: Stitching Together the Thread of Correlation: The Curious Case of Sewing Machine Operators in North Dakota and Kerosene Consumption in Ireland
Your correlation inspection deserves a standing ovation!
Correlation ID: 1738 · Black Variable ID: 12717 · Red Variable ID: 24557