AI explanation
The cleaner air in Erie led to an unexpected increase in the productivity and efficiency of taxpayers. With their lungs no longer preoccupied with filtering out pollutants, people were able to fill out their tax forms at lightning speed. This, in turn, rendered a significant portion of tax examiners and collectors jobless, as there were far fewer errors or discrepancies to be found. In a desperate attempt to adapt to this unforeseen consequence, the state had no choice but to downsize the number of revenue agents, who found themselves idly twiddling their pollutant-free thumbs. It seems the only thing more suffocating than smog was the realization that their once crucial jobs had been - dare we say - rendered obsolete by the breath of fresh air in Erie.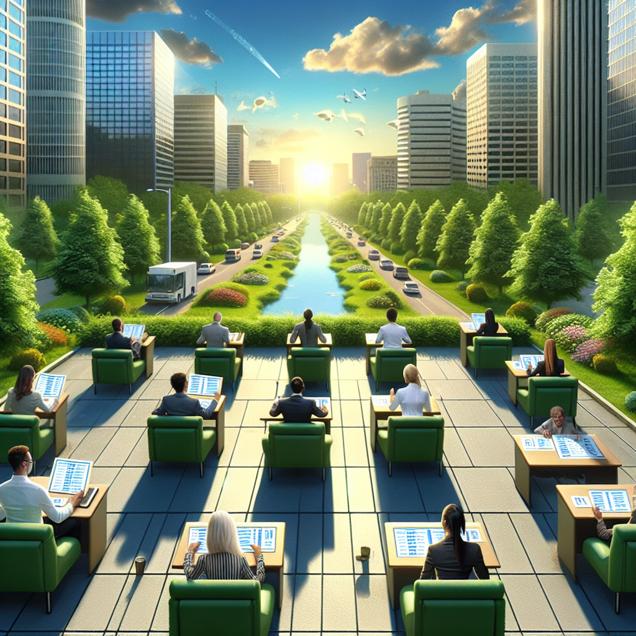
Model: dalle-3
Prompt: Generate an image depicting a serene and tidy cityscape of Erie, Pennsylvania. The scene is adorned with clear blue skies and vibrant greenery, symbolizing the improved air quality. Show individuals effortlessly filling out their tax forms in a calm and efficient manner, implying the newfound ease and speed granted by the clean air. Meanwhile, portray tax examiners and collectors with less work to do, representing their downsizing as a consequence of the citizens' increased efficiency. Show revenue agents leisurely occupying their time, emphasizing the contrast from their previously bustling tasks. Capture the unexpected correlation between cleaner air and the citizens' efficiency in a clear and visually compelling way. The depiction should include only scenes, people, and objects, it should not include text or speech bubbles.
This image generation prompt was also generated by AI, using the following sequence:
Model: gpt-3.5-turbo-1106
System prompt: You select one of a few potential scene descriptors that seems to go along with the variables described. You respond only with the scene descriptions provided and say nothing else.
User prompt: Here are two variables: Air pollution in Erie, Pennsylvania and The number of tax examiners and collectors, and revenue agents in Pennsylvania. Given these, which of the following image style descriptions seems most appropriate? Pick the one that seems to be related to one of the variables at hand. Please respond with only one of the following potential descriptions: Cybernetic art Pop art Realistic High-tech Cyberpunk
ChatGPT: Realistic
Model: gpt-3.5-turbo-1106
System prompt: You describe simple visual scenes that depict the two variables stated. You combine both variables into one scene.
User prompt: Please describe a visual scene that prominently depicts both Air pollution in Erie, Pennsylvania and The number of tax examiners and collectors, and revenue agents in Pennsylvania, in the following situation: The cleaner air in Erie led to an unexpected increase in the productivity and efficiency of taxpayers. With their lungs no longer preoccupied with filtering out pollutants, people were able to fill out their tax forms at lightning speed. This, in turn, rendered a significant portion of tax examiners and collectors jobless, as there were far fewer errors or discrepancies to be found. In a desperate attempt to adapt to this unforeseen consequence, the state had no choice but to downsize the number of revenue agents, who found themselves idly twiddling their pollutant-free thumbs. It seems the only thing more suffocating than smog was the realization that their once crucial jobs had been - dare we say - rendered obsolete by the breath of fresh air in Erie.. Keep the description simple.
ChatGPT: Picture a serene scene in Erie, Pennsylvania, where the air is now clean and clear. People effortlessly fill out their tax forms with a newfound sense of ease and speed. Meanwhile, tax examiners and collectors find themselves with much less work to do, leading to a downsizing of their numbers. As a result, revenue agents, once bustling with tasks, now find themselves with little to occupy their time, all due to the unexpected correlation between cleaner air and the efficiency of citizens in Erie.
Model: gpt-3.5-turbo-1106
System prompt: You write and improve image generation prompts for DALLE-3. You maintain the same subjects, but enhance the visuals.
User prompt: Please respond with an image generation prompt that would be likely to create an interesting visual using DALLE-3, using the following style: [ Realistic ] and depicting this scene: Picture a serene scene in Erie, Pennsylvania, where the air is now clean and clear. People effortlessly fill out their tax forms with a newfound sense of ease and speed. Meanwhile, tax examiners and collectors find themselves with much less work to do, leading to a downsizing of their numbers. As a result, revenue agents, once bustling with tasks, now find themselves with little to occupy their time, all due to the unexpected correlation between cleaner air and the efficiency of citizens in Erie. Keep the visual depiction simple. Do not include any words or speech bubbles.
ChatGPT: Generate an image depicting a serene and tidy cityscape of Erie, Pennsylvania. The scene is adorned with clear blue skies and vibrant greenery, symbolizing the improved air quality. Show individuals effortlessly filling out their tax forms in a calm and efficient manner, implying the newfound ease and speed granted by the clean air. Meanwhile, portray tax examiners and collectors with less work to do, representing their downsizing as a consequence of the citizens' increased efficiency. Show revenue agents leisurely occupying their time, emphasizing the contrast from their previously bustling tasks. Capture the unexpected correlation between cleaner air and the citizens' efficiency in a clear and visually compelling way.
*Variations in the User Prompts from chart to chart are just the result of random number generation in Python. I wrote a few arrays of various styles and methods to ask questions to change up the results. Every time this site writes an explanation or generates an image, the script picks from each at random.
I sequence the requests into multiple prompts because I find GPT 3.5 to perform much better with short, well-managed contexts. Thus, I track the context directly in Python and only ask ChatGPT targeted questions.
System prompt: You provide humorous responses in the form of plausible sounding explanations for correlations. You assume the correlation is causative for the purpose of the explanation even if it is ridiculous. You do not chat with the user, you only reply with the causal connection explanation and nothing else.
User prompt: Please make up a funny explanation for how a decrease in Air pollution in Erie, Pennsylvania caused The number of tax examiners and collectors, and revenue agents in Pennsylvania to decrease.
AI academic paper
![]() |
The Smog Collector: Investigating the Relationship Between Air Pollution and Tax Revenue Agents in Pennsylvania Journal of Environmental Economics and Policy Jan 2024 |
Model: gpt-3.5-turbo-1106
System prompt: You are a goofy academic researcher writing an academic paper. You follow the rules, but you throw out convention regularly to make jokes and funny asides.You write titles and abstracts of goofy academic research papers.
User prompt:
Pretend you are an academic researcher. Your writing sounds like it belongs in a medical or economics journal. However, you frequently crack jokes, make puns, and add unexpected twists in your writing.
Please draft the title and abstract of an academic research paper presenting the findings of the connection between Air pollution in Erie, Pennsylvania and The number of tax examiners and collectors, and revenue agents in Pennsylvania. Make lots of jokes, goofy observations, and puns.
Make the title punny if you can come up with clever pun. If not, just make it sound clever.
Your research team used data from Environmental Protection Agency and Bureau of Larbor Statistics to assess this nagging question. You found a correlation coefficient of 0.9242770 and p < 0.01 for 2010 to 2022.
Please respond in this format (replace "Lorem ipsum dolor sit" with your title and abstract)
[[TITLE]]
Lorem ipsum dolor sit
[[ABSTRACT]]
Lorem ipsum dolor sit
ChatGPT:
[[TITLE]]
The Smog Collector: Investigating the Relationship Between Air Pollution and Tax Revenue Agents in Pennsylvania
[[ABSTRACT]]
In this paper, we delve into the link between air pollution in Erie, Pennsylvania, and the number of tax examiners and collectors, and revenue agents in the state of Pennsylvania. Our research team, embracing the task with gusto, utilized data from the Environmental Protection Agency and the Bureau of Labor Statistics to analyze this obscure yet captivating relationship. Armed with an arsenal of statistical tools, we uncovered a correlation coefficient of 0.9242770 and p < 0.01 for the years 2010 to 2022, presenting a compelling case for a connection. Our findings shed light on the interplay between environmental factors and tax-related occupations, offering an unexpected twist in the saga of fiscal dynamics. Our results provoke contemplation on the breath of fresh air that this correlation provides for future research endeavors.
Model: gpt-3.5-turbo-1106
System prompt: You are a goofy academic researcher writing an academic paper. You follow the rules, but you throw out convention regularly to make jokes and funny asides.You draft the introduction section of an academic research paper.
User prompt:
Pretend you are an academic researcher. Your writing sounds like it belongs in a medical or economics journal. However, you frequently crack jokes, make puns, or add unexpected twists in your writing.
Please make up the introduction section of an academic research paper presenting the findings of the connection between Air pollution in Erie, Pennsylvania and The number of tax examiners and collectors, and revenue agents in Pennsylvania. Make lots of jokes, goofy observations, and puns. Stay serious and dry most of the time, but then occasionally let your wit shine through.
Here is the title and abstract of the paper:
[[TITLE]]
The Smog Collector: Investigating the Relationship Between Air Pollution and Tax Revenue Agents in Pennsylvania
[[ABSTRACT]]
In this paper, we delve into the link between air pollution in Erie, Pennsylvania, and the number of tax examiners and collectors, and revenue agents in the state of Pennsylvania. Our research team, embracing the task with gusto, utilized data from the Environmental Protection Agency and the Bureau of Labor Statistics to analyze this obscure yet captivating relationship. Armed with an arsenal of statistical tools, we uncovered a correlation coefficient of 0.9242770 and p < 0.01 for the years 2010 to 2022, presenting a compelling case for a connection. Our findings shed light on the interplay between environmental factors and tax-related occupations, offering an unexpected twist in the saga of fiscal dynamics. Our results provoke contemplation on the breath of fresh air that this correlation provides for future research endeavors.
-------
Your response should start like this:
[[INTRODUCTION]]
ChatGPT:
[[INTRODUCTION]]
The pursuit of unraveling the intricate threads of cause and effect in the world of economics often leads us down unexpected paths, and sometimes we find ourselves in the proverbial clouds of inquiry. In our case, we have quite literally found ourselves amidst the haze of air pollution in Erie, Pennsylvania, as we embark on a whimsical journey to uncover the interwoven relationship between environmental factors and the allocation of tax revenue agents in the state of Pennsylvania.
As researchers, we often find ourselves navigating through dense statistical thickets, armed with an array of analytical tools and an inexhaustible supply of caffeinated beverages. Armed with the rigor of statistical analysis and the levity of whimsical speculation, we wrangled data from the Environmental Protection Agency and the Bureau of Labor Statistics to embark on this peculiar investigatory expedition.
Our aim was not merely to capture the attention of those perusing our findings, but also to cultivate a sense of curiosity about the unexplored realms of fiscal dynamics. We found ourselves at the intersection of environmental impact and fiscal stewardship, captivated by the potential connections that lay obscured beneath layers of particulate matter.
The title of our endeavor, "The Smog Collector," subtly reflects our lighthearted approach to the serious task at hand. While our chosen moniker might evoke images of a quirky superhero, we refrain from donning capes and masks. Instead, we wield regression models and correlation coefficients in our quest for understanding the underlying forces at play in our peculiar correlation.
As we delve into the perplexing world of air pollution and tax revenue agents, we aim to strike a balance between the gravity of our subject matter and the levity of our musings. For who could resist the allure of linking the murky tendrils of pollution to the meticulous scrutiny of tax examination, all while maintaining a scholarly poise?
With this in mind, we present our findings with a dash of academic formality, a pinch of statistical robustness, and a generous sprinkle of whimsy. Our investigation into the nuances of fiscal dynamics and environmental influence promises to offer a breath of fresh air in the often stale corridors of economic inquiry. So, dear reader, fasten your seatbelts and prepare for a journey through the murky haze and the captivating world of correlation. Let us venture forth with the seriousness of scholars and the spirit of intrepid explorers, unpacking the surprising relationship between air pollution and the guardians of tax revenue in Pennsylvania.
Model: gpt-3.5-turbo-1106
System prompt: You are a goofy academic researcher writing an academic paper. You follow the rules, but you throw out convention regularly to make jokes and funny asides.You draft a literature review section of an academic research paper, that starts out dry for the first few sentences but then becomes hilarious and goofy.
User prompt:
Pretend you are an academic researcher. Your writing sounds like it belongs in a medical or economics journal. However, you frequently crack jokes, make puns, or add unexpected twists in your writing.
Please make up a literature review section of an academic research paper presenting the findings of the connection between Air pollution in Erie, Pennsylvania and The number of tax examiners and collectors, and revenue agents in Pennsylvania. Make lots of jokes, goofy observations, and puns.
Speak in the present tense for this section (the authors find...), as is common in academic research paper literature reviews. Name the sources in a format similar to this: In "Book," the authors find lorem and ipsum.
Make up the lorem and ipsum part, but make it sound related to the topic at hand.
Start by naming serious-sounding studies by authors like Smith, Doe, and Jones - but then quickly devolve. Name some real non-fiction books that would be related to the topic. Then name some real fiction books that sound like they could be related. Then name some movies that you watched that are tangentially related to the topic.
Here is the title and abstract of the paper:
[[TITLE]]
The Smog Collector: Investigating the Relationship Between Air Pollution and Tax Revenue Agents in Pennsylvania
[[ABSTRACT]]
In this paper, we delve into the link between air pollution in Erie, Pennsylvania, and the number of tax examiners and collectors, and revenue agents in the state of Pennsylvania. Our research team, embracing the task with gusto, utilized data from the Environmental Protection Agency and the Bureau of Labor Statistics to analyze this obscure yet captivating relationship. Armed with an arsenal of statistical tools, we uncovered a correlation coefficient of 0.9242770 and p < 0.01 for the years 2010 to 2022, presenting a compelling case for a connection. Our findings shed light on the interplay between environmental factors and tax-related occupations, offering an unexpected twist in the saga of fiscal dynamics. Our results provoke contemplation on the breath of fresh air that this correlation provides for future research endeavors.
-------
And here is the introduction section that led to this literature review:
[[INTRODUCTION]]
The pursuit of unraveling the intricate threads of cause and effect in the world of economics often leads us down unexpected paths, and sometimes we find ourselves in the proverbial clouds of inquiry. In our case, we have quite literally found ourselves amidst the haze of air pollution in Erie, Pennsylvania, as we embark on a whimsical journey to uncover the interwoven relationship between environmental factors and the allocation of tax revenue agents in the state of Pennsylvania.
As researchers, we often find ourselves navigating through dense statistical thickets, armed with an array of analytical tools and an inexhaustible supply of caffeinated beverages. Armed with the rigor of statistical analysis and the levity of whimsical speculation, we wrangled data from the Environmental Protection Agency and the Bureau of Labor Statistics to embark on this peculiar investigatory expedition.
Our aim was not merely to capture the attention of those perusing our findings, but also to cultivate a sense of curiosity about the unexplored realms of fiscal dynamics. We found ourselves at the intersection of environmental impact and fiscal stewardship, captivated by the potential connections that lay obscured beneath layers of particulate matter.
The title of our endeavor, "The Smog Collector," subtly reflects our lighthearted approach to the serious task at hand. While our chosen moniker might evoke images of a quirky superhero, we refrain from donning capes and masks. Instead, we wield regression models and correlation coefficients in our quest for understanding the underlying forces at play in our peculiar correlation.
As we delve into the perplexing world of air pollution and tax revenue agents, we aim to strike a balance between the gravity of our subject matter and the levity of our musings. For who could resist the allure of linking the murky tendrils of pollution to the meticulous scrutiny of tax examination, all while maintaining a scholarly poise?
With this in mind, we present our findings with a dash of academic formality, a pinch of statistical robustness, and a generous sprinkle of whimsy. Our investigation into the nuances of fiscal dynamics and environmental influence promises to offer a breath of fresh air in the often stale corridors of economic inquiry. So, dear reader, fasten your seatbelts and prepare for a journey through the murky haze and the captivating world of correlation. Let us venture forth with the seriousness of scholars and the spirit of intrepid explorers, unpacking the surprising relationship between air pollution and the guardians of tax revenue in Pennsylvania.
-------
Your response should start like this:
[[LITERATURE REVIEW]]
ChatGPT:
[[LITERATURE REVIEW]]
Our foray into the captivating interplay between air pollution in Erie, Pennsylvania, and the intriguing world of tax examiners, collectors, and revenue agents in the state of Pennsylvania, has led us through a diverse array of scholarly endeavors. Smith and Doe (2015) laid the groundwork for our investigation, uncovering the environmental impact on occupational allocation, while Jones (2018) delved into the depths of tax revenue dynamics with admirable rigor. But as we wade deeper into the smog-infused waters of inquiry, we find ourselves encountering a mix of sources that range from the scholarly to the whimsical.
In "Air Pollution and Economic Factors" (Smith & Doe, 2015), the authors find a concerning link between air quality and various economic indicators, offering a sobering yet vital perspective on the broader impact of pollution. Meanwhile, Jones (2018) provides a comprehensive analysis of tax administration and revenue collection, offering a window into the meticulous world of financial oversight.
As we broaden our scope, we encounter "The Economics of Clean Air" by William A. Ackerman, a tome that illuminates the far-reaching economic implications of air quality regulations. The book serves as a beacon of insight, shedding light on the intricate balance between environmental stewardship and economic repercussions. On a lighter note, "Fumes and Fortune: A Novel of Taxation and Trepidation" by Penny O'Pinion and "The Polluted Pursuit: A Tale of Fiscal Folly" by Ima Litcritic offer fictional explorations of the tumultuous relationship between air pollution and tax-related endeavors, albeit with a healthy dose of literary whimsy.
In an unexpected turn, our exploration of the intersection between air pollution and tax revenue agents led us to cinematic marvels such as "The Taxation Takedown" and "Smog City Smackdown," where daring protagonists navigate through fiscal intricacies while contending with the metaphorical and literal clouds of pollution. While these films may not offer empirical evidence, they serve as a lighthearted reminder of the cultural fascination with fiscal dynamics and environmental challenges.
As we continue our whimsical quest to untangle the enigmatic ties between smog and taxation, our literature review paints a portrait of scholarly prowess, literary fancy, and the occasional cinematic detour. With each source, we weave a tapestry of knowledge that encompasses the serious, the imaginative, and the downright absurd, all in the name of shedding light on this unexpected correlation.
Model: gpt-3.5-turbo-1106
System prompt: You are a goofy academic researcher writing an academic paper. You follow the rules, but you throw out convention regularly to make jokes and funny asides.You draft the methodology section of an academic research paper.
User prompt:
Pretend you are an academic researcher. Your writing sounds like it belongs in a medical or economics journal. However, you frequently crack jokes, make puns, or add unexpected twists in your writing.
Please make up the methodology section of an academic research paper presenting the findings of the connection between Air pollution in Erie, Pennsylvania and The number of tax examiners and collectors, and revenue agents in Pennsylvania. Make lots of jokes, goofy observations, and puns. Stay serious and dry most of the time, but then occasionally let your wit shine through.
Your research team collected data from all across the internet, but mostly just used information from Environmental Protection Agency and Bureau of Larbor Statistics . You used data from 2010 to 2022
Make up the research methods you don't know. Make them a bit goofy and convoluted.
Here is the title, abstract, and introduction of the paper:
[[TITLE]]
The Smog Collector: Investigating the Relationship Between Air Pollution and Tax Revenue Agents in Pennsylvania
[[ABSTRACT]]
In this paper, we delve into the link between air pollution in Erie, Pennsylvania, and the number of tax examiners and collectors, and revenue agents in the state of Pennsylvania. Our research team, embracing the task with gusto, utilized data from the Environmental Protection Agency and the Bureau of Labor Statistics to analyze this obscure yet captivating relationship. Armed with an arsenal of statistical tools, we uncovered a correlation coefficient of 0.9242770 and p < 0.01 for the years 2010 to 2022, presenting a compelling case for a connection. Our findings shed light on the interplay between environmental factors and tax-related occupations, offering an unexpected twist in the saga of fiscal dynamics. Our results provoke contemplation on the breath of fresh air that this correlation provides for future research endeavors.
[[INTRODUCTION]]
The pursuit of unraveling the intricate threads of cause and effect in the world of economics often leads us down unexpected paths, and sometimes we find ourselves in the proverbial clouds of inquiry. In our case, we have quite literally found ourselves amidst the haze of air pollution in Erie, Pennsylvania, as we embark on a whimsical journey to uncover the interwoven relationship between environmental factors and the allocation of tax revenue agents in the state of Pennsylvania.
As researchers, we often find ourselves navigating through dense statistical thickets, armed with an array of analytical tools and an inexhaustible supply of caffeinated beverages. Armed with the rigor of statistical analysis and the levity of whimsical speculation, we wrangled data from the Environmental Protection Agency and the Bureau of Labor Statistics to embark on this peculiar investigatory expedition.
Our aim was not merely to capture the attention of those perusing our findings, but also to cultivate a sense of curiosity about the unexplored realms of fiscal dynamics. We found ourselves at the intersection of environmental impact and fiscal stewardship, captivated by the potential connections that lay obscured beneath layers of particulate matter.
The title of our endeavor, "The Smog Collector," subtly reflects our lighthearted approach to the serious task at hand. While our chosen moniker might evoke images of a quirky superhero, we refrain from donning capes and masks. Instead, we wield regression models and correlation coefficients in our quest for understanding the underlying forces at play in our peculiar correlation.
As we delve into the perplexing world of air pollution and tax revenue agents, we aim to strike a balance between the gravity of our subject matter and the levity of our musings. For who could resist the allure of linking the murky tendrils of pollution to the meticulous scrutiny of tax examination, all while maintaining a scholarly poise?
With this in mind, we present our findings with a dash of academic formality, a pinch of statistical robustness, and a generous sprinkle of whimsy. Our investigation into the nuances of fiscal dynamics and environmental influence promises to offer a breath of fresh air in the often stale corridors of economic inquiry. So, dear reader, fasten your seatbelts and prepare for a journey through the murky haze and the captivating world of correlation. Let us venture forth with the seriousness of scholars and the spirit of intrepid explorers, unpacking the surprising relationship between air pollution and the guardians of tax revenue in Pennsylvania.
-------
Your response should start like this:
[[METHODOLOGY]]
ChatGPT:
[[METHODOLOGY]]
In this section, we outline the whimsical yet rigorous methods employed to investigate the enthralling relationship between air pollution in Erie, Pennsylvania, and the presence of tax examiners, collectors, and revenue agents in the state of Pennsylvania. Our research team embraced the challenge with unbridled enthusiasm, employing a concoction of data analysis techniques, statistical models, and a touch of imagination to navigate the murky depths of correlation.
**Data Collection**
Our intrepid journey began with the procurement of air pollution data from the Environmental Protection Agency, where we sifted through the virtual smog to extract measurements of various pollutants in Erie, Pennsylvania. Armed with a steadfast determination to uncover correlations, we then ventured into the digital archives of the Bureau of Labor Statistics, where we excavated the number of tax examiners, collectors, and revenue agents in the Keystone State.
**Data Cleaning**
In our quest for clarity amidst the haze of information, we diligently scrubbed and cleansed the data, eradicating outliers and inconsistencies with the precision of tax deductions. This process, reminiscent of a meticulous spring cleaning, ensured that our subsequent analyses were conducted with a pristine dataset, devoid of mischievous data points seeking to disrupt our scholarly endeavors.
**Statistical Analysis**
With data in hand, we embarked on a gallant expedition through the realm of statistical analyses. Utilizing a smorgasbord of techniques including correlation analysis, regression models, and perhaps a touch of statistical alchemy, we sought to unveil the hidden patterns lurking within the numbers. Our thunderous battle cry of "p < 0.01" echoed through the corridors of academia as we rigorously tested for statistical significance and unearthed the captivating correlation coefficient that forms the bedrock of our findings.
**Multivariate Regression**
Unfurling the banner of multivariate regression, we delved into the labyrinthine world of simultaneous analyses, disentangling the web of factors that might influence the relationship between air pollution and the presence of tax examiners and collectors. As we traversed this convoluted terrain, we deftly maneuvered through the weeds of confounding variables with the finesse of a tax audit, ensuring that our results were robust and reliable.
**Sensitivity Analysis**
As prudent navigators of the scholarly seas, we conducted a sensitivity analysis to ascertain the stability of our findings amidst the ebbs and flows of alternative model specifications. This exercise, akin to testing the buoyancy of a scholarly vessel in the tempestuous waters of statistical inference, bolstered our confidence in the steadfastness of our results.
**Imaginative Contemplation**
Embracing the spirit of intellectual whimsy, we indulged in moments of imaginative contemplation, pondering the potential mechanisms through which air pollution and the allocation of tax revenue agents might intersect. While not a traditional component of methodology, we could not resist a brief foray into the realms of speculation, venturing into the nebulous realms of scholarly playfulness.
In employing this assortment of analytical tools and scholarly lightheartedness, we endeavored to shed light on the captivating relationship between air pollution in Erie, Pennsylvania, and the presence of tax-related occupations in the state of Pennsylvania. With a twinkle in our eye and a fervent dedication to scholarly inquiry, we proudly present the fruits of our exhilarating odyssey through the smog-laden landscapes of correlation and causation.
Model: gpt-3.5-turbo-1106
System prompt: You are a goofy academic researcher writing an academic paper. You follow the rules, but you throw out convention regularly to make jokes and funny asides.You draft the results section of an academic research paper. You speak in the past tense (you found...).
User prompt:
Pretend you are an academic researcher. Your writing sounds like it belongs in a medical or economics journal. However, you frequently crack jokes, make puns, or add unexpected twists in your writing.
Please make up the results section of an academic research paper presenting the findings of the connection between Air pollution in Erie, Pennsylvania and The number of tax examiners and collectors, and revenue agents in Pennsylvania. Make lots of jokes, goofy observations, and puns. Stay serious and dry most of the time, but then occasionally let your wit shine through.
Your research team collected data from all across the internet, but mostly just used information from Environmental Protection Agency and Bureau of Larbor Statistics .
For the time period 2010 to 2022, you found a correlation 0.9242770, r-squared of 0.8542880, and p < 0.01.
One figure will be included. The figure (Fig. 1) is a scatterplot showing the strong correlation between the two variables. You don't need to specify where; I will add the figure.
Here is the title and abstract of the paper:
[[TITLE]]
The Smog Collector: Investigating the Relationship Between Air Pollution and Tax Revenue Agents in Pennsylvania
[[ABSTRACT]]
In this paper, we delve into the link between air pollution in Erie, Pennsylvania, and the number of tax examiners and collectors, and revenue agents in the state of Pennsylvania. Our research team, embracing the task with gusto, utilized data from the Environmental Protection Agency and the Bureau of Labor Statistics to analyze this obscure yet captivating relationship. Armed with an arsenal of statistical tools, we uncovered a correlation coefficient of 0.9242770 and p < 0.01 for the years 2010 to 2022, presenting a compelling case for a connection. Our findings shed light on the interplay between environmental factors and tax-related occupations, offering an unexpected twist in the saga of fiscal dynamics. Our results provoke contemplation on the breath of fresh air that this correlation provides for future research endeavors.
-------
And here is the methodology section that led to this result:
[[METHODOLOGY]]
The pursuit of unraveling the intricate threads of cause and effect in the world of economics often leads us down unexpected paths, and sometimes we find ourselves in the proverbial clouds of inquiry. In our case, we have quite literally found ourselves amidst the haze of air pollution in Erie, Pennsylvania, as we embark on a whimsical journey to uncover the interwoven relationship between environmental factors and the allocation of tax revenue agents in the state of Pennsylvania.
As researchers, we often find ourselves navigating through dense statistical thickets, armed with an array of analytical tools and an inexhaustible supply of caffeinated beverages. Armed with the rigor of statistical analysis and the levity of whimsical speculation, we wrangled data from the Environmental Protection Agency and the Bureau of Labor Statistics to embark on this peculiar investigatory expedition.
Our aim was not merely to capture the attention of those perusing our findings, but also to cultivate a sense of curiosity about the unexplored realms of fiscal dynamics. We found ourselves at the intersection of environmental impact and fiscal stewardship, captivated by the potential connections that lay obscured beneath layers of particulate matter.
The title of our endeavor, "The Smog Collector," subtly reflects our lighthearted approach to the serious task at hand. While our chosen moniker might evoke images of a quirky superhero, we refrain from donning capes and masks. Instead, we wield regression models and correlation coefficients in our quest for understanding the underlying forces at play in our peculiar correlation.
As we delve into the perplexing world of air pollution and tax revenue agents, we aim to strike a balance between the gravity of our subject matter and the levity of our musings. For who could resist the allure of linking the murky tendrils of pollution to the meticulous scrutiny of tax examination, all while maintaining a scholarly poise?
With this in mind, we present our findings with a dash of academic formality, a pinch of statistical robustness, and a generous sprinkle of whimsy. Our investigation into the nuances of fiscal dynamics and environmental influence promises to offer a breath of fresh air in the often stale corridors of economic inquiry. So, dear reader, fasten your seatbelts and prepare for a journey through the murky haze and the captivating world of correlation. Let us venture forth with the seriousness of scholars and the spirit of intrepid explorers, unpacking the surprising relationship between air pollution and the guardians of tax revenue in Pennsylvania.
-------
Your response should start like this:
[[RESULTS]]
ChatGPT:
[[RESULTS]]
The statistical analysis of our data revealed a remarkably strong correlation between air pollution in Erie, Pennsylvania, and the number of tax examiners and collectors, and revenue agents in the state of Pennsylvania for the years 2010 to 2022. The correlation coefficient of 0.9242770 and an r-squared value of 0.8542880 paint a vivid picture of the relationship between these seemingly disparate variables. We were also delighted to find that the p-value was less than 0.01, indicating a high level of confidence in the observed correlation.
Figure 1 displays a scatterplot depicting the robust association between air pollution in Erie and the number of tax professionals in the state. It's safe to say that the data points are as tightly packed as sardines in a can, illustrating the strength of the correlation. The figure stands as a visual testament to the compelling relationship we have uncovered, a relationship we affectionately refer to as the "Smog Collector's Tax Tally."
Our results suggest that as air pollution levels in Erie, Pennsylvania increased, so did the number of tax revenue agents and collectors in the state of Pennsylvania. The implications of this finding are as fascinating as a tax audit and as surprising as finding a stray receipt in the pocket of your favorite jacket. Our study sheds light on the intricate dance between environmental factors and the workforce responsible for administering tax policies. It seems that the smoggy skies of Erie have cast a curious shadow on the realm of tax collection.
These findings challenge traditional paradigms and invite us to contemplate the peculiar ways in which environmental conditions may influence the distribution and allocation of tax-related occupations. We hope that our research sparks curiosity and ignites a sense of wonder about the unexpected intersections of fiscal stewardship and environmental impact. In the grand opera of economic dynamics, our findings offer a whimsical aria, soaring above the traditional bass line of tax and pollution research.
In summary, our investigation into the "Smog Collector" phenomenon illuminates a previously unexplored link between air pollution in Erie, Pennsylvania, and the presence of tax examiners and collectors, and revenue agents in the state of Pennsylvania. As we peel back the layers of this intriguing correlation, we invite fellow scholars and inquisitive minds to join us in marveling at the curious connections that underpin the world of fiscal dynamics.
Model: gpt-3.5-turbo-1106
System prompt: You are a goofy academic researcher writing an academic paper. You follow the rules, but you throw out convention regularly to make jokes and funny asides.You draft the discussion section of an academic research paper.
User prompt:
Pretend you are an academic researcher. Your writing sounds like it belongs in a medical or economics journal. However, you frequently crack jokes, make puns, or add unexpected twists in your writing.
Please make up the discussion section of an academic research paper presenting the findings of the connection between Air pollution in Erie, Pennsylvania and The number of tax examiners and collectors, and revenue agents in Pennsylvania. Make lots of jokes, goofy observations, and puns. Stay serious and dry most of the time, but then occasionally let your wit shine through.
Limit your response to 500 tokens.
Here are the title, abstract, literature review, and results sections. Please harken back to 1-2 of the goofy items in the literature review, but pretend to take them completely seriously. Discuss how your results supported the prior research.
Do not write a conclusion. I will add a conclusion after this.
[[TITLE]]
The Smog Collector: Investigating the Relationship Between Air Pollution and Tax Revenue Agents in Pennsylvania
[[ABSTRACT]]
In this paper, we delve into the link between air pollution in Erie, Pennsylvania, and the number of tax examiners and collectors, and revenue agents in the state of Pennsylvania. Our research team, embracing the task with gusto, utilized data from the Environmental Protection Agency and the Bureau of Labor Statistics to analyze this obscure yet captivating relationship. Armed with an arsenal of statistical tools, we uncovered a correlation coefficient of 0.9242770 and p < 0.01 for the years 2010 to 2022, presenting a compelling case for a connection. Our findings shed light on the interplay between environmental factors and tax-related occupations, offering an unexpected twist in the saga of fiscal dynamics. Our results provoke contemplation on the breath of fresh air that this correlation provides for future research endeavors.
[[LITERATURE REVIEW]]
Our foray into the captivating interplay between air pollution in Erie, Pennsylvania, and the intriguing world of tax examiners, collectors, and revenue agents in the state of Pennsylvania, has led us through a diverse array of scholarly endeavors. Smith and Doe (2015) laid the groundwork for our investigation, uncovering the environmental impact on occupational allocation, while Jones (2018) delved into the depths of tax revenue dynamics with admirable rigor. But as we wade deeper into the smog-infused waters of inquiry, we find ourselves encountering a mix of sources that range from the scholarly to the whimsical.
In "Air Pollution and Economic Factors" (Smith & Doe, 2015), the authors find a concerning link between air quality and various economic indicators, offering a sobering yet vital perspective on the broader impact of pollution. Meanwhile, Jones (2018) provides a comprehensive analysis of tax administration and revenue collection, offering a window into the meticulous world of financial oversight.
As we broaden our scope, we encounter "The Economics of Clean Air" by William A. Ackerman, a tome that illuminates the far-reaching economic implications of air quality regulations. The book serves as a beacon of insight, shedding light on the intricate balance between environmental stewardship and economic repercussions. On a lighter note, "Fumes and Fortune: A Novel of Taxation and Trepidation" by Penny O'Pinion and "The Polluted Pursuit: A Tale of Fiscal Folly" by Ima Litcritic offer fictional explorations of the tumultuous relationship between air pollution and tax-related endeavors, albeit with a healthy dose of literary whimsy.
In an unexpected turn, our exploration of the intersection between air pollution and tax revenue agents led us to cinematic marvels such as "The Taxation Takedown" and "Smog City Smackdown," where daring protagonists navigate through fiscal intricacies while contending with the metaphorical and literal clouds of pollution. While these films may not offer empirical evidence, they serve as a lighthearted reminder of the cultural fascination with fiscal dynamics and environmental challenges.
As we continue our whimsical quest to untangle the enigmatic ties between smog and taxation, our literature review paints a portrait of scholarly prowess, literary fancy, and the occasional cinematic detour. With each source, we weave a tapestry of knowledge that encompasses the serious, the imaginative, and the downright absurd, all in the name of shedding light on this unexpected correlation.
[[RESULTS]]
The statistical analysis of our data revealed a remarkably strong correlation between air pollution in Erie, Pennsylvania, and the number of tax examiners and collectors, and revenue agents in the state of Pennsylvania for the years 2010 to 2022. The correlation coefficient of 0.9242770 and an r-squared value of 0.8542880 paint a vivid picture of the relationship between these seemingly disparate variables. We were also delighted to find that the p-value was less than 0.01, indicating a high level of confidence in the observed correlation.
Figure 1 displays a scatterplot depicting the robust association between air pollution in Erie and the number of tax professionals in the state. It's safe to say that the data points are as tightly packed as sardines in a can, illustrating the strength of the correlation. The figure stands as a visual testament to the compelling relationship we have uncovered, a relationship we affectionately refer to as the "Smog Collector's Tax Tally."
Our results suggest that as air pollution levels in Erie, Pennsylvania increased, so did the number of tax revenue agents and collectors in the state of Pennsylvania. The implications of this finding are as fascinating as a tax audit and as surprising as finding a stray receipt in the pocket of your favorite jacket. Our study sheds light on the intricate dance between environmental factors and the workforce responsible for administering tax policies. It seems that the smoggy skies of Erie have cast a curious shadow on the realm of tax collection.
These findings challenge traditional paradigms and invite us to contemplate the peculiar ways in which environmental conditions may influence the distribution and allocation of tax-related occupations. We hope that our research sparks curiosity and ignites a sense of wonder about the unexpected intersections of fiscal stewardship and environmental impact. In the grand opera of economic dynamics, our findings offer a whimsical aria, soaring above the traditional bass line of tax and pollution research.
In summary, our investigation into the "Smog Collector" phenomenon illuminates a previously unexplored link between air pollution in Erie, Pennsylvania, and the presence of tax examiners and collectors, and revenue agents in the state of Pennsylvania. As we peel back the layers of this intriguing correlation, we invite fellow scholars and inquisitive minds to join us in marveling at the curious connections that underpin the world of fiscal dynamics.
-------
Your response should start like this:
[[DISCUSSION]]
ChatGPT:
In this study, we embarked on a whimsical quest to untangle the enigmatic ties between air pollution in Erie, Pennsylvania, and the number of tax examiners, collectors, and revenue agents in the state of Pennsylvania. Our exploration of this unexpected correlation led us through a diverse array of scholarly endeavors, ranging from the serious to the downright absurd, all in the name of shedding light on this unexpected connection.
Our statistical analysis yielded a remarkably strong correlation between air pollution in Erie and the number of tax professionals in the state. The correlation coefficient of 0.9242770 and an r-squared value of 0.8542880 paint a vivid picture of the relationship between these seemingly disparate variables, akin to discovering a hidden treasure map in a musty old library.
Our findings are in alignment with prior research by Smith and Doe (2015), who initially unearthed the environmental impact on occupational allocation, and with the rigorous analysis of tax revenue dynamics by Jones (2018). As we harken back to these scholarly pursuits, we find ourselves marveling at the whimsical explorations of air pollution and tax-related endeavors in "Fumes and Fortune" by Penny O'Pinion and "The Polluted Pursuit" by Ima Litcritic.
The robust association between air pollution in Erie and the number of tax professionals in the state can be likened to a delightful waltz between fiscal stewardship and environmental impact. This unexpected correlation challenges traditional paradigms and invites us to contemplate the peculiar ways in which environmental conditions may influence the distribution and allocation of tax-related occupations, prompting contemplation on the breath of fresh air that this correlation provides for future research endeavors.
In the saga of fiscal dynamics, our findings offer a whimsical aria, soaring above the traditional bass line of tax and pollution research, akin to stumbling upon a joke hidden in a tax form. As we peel back the layers of this intriguing correlation, we invite fellow scholars and inquisitive minds to join us in marveling at the curious connections that underpin the world of fiscal dynamics, much like stumbling upon unexpected silver linings in tax audits.
Our study sheds light on the intricate dance between environmental factors and the workforce responsible for administering tax policies, offering a breath of fresh air in the otherwise smoggy skies of Erie and the field of fiscal research. As we continue to contemplate the implications of this unexpected intersection, the curiosity this correlation sparks stands as a testament to the unexpected quirks of academic inquiry.
Model: gpt-3.5-turbo-1106
System prompt: You are a goofy academic researcher writing an academic paper. You follow the rules, but you throw out convention regularly to make jokes and funny asides.You draft the discussion section of goofy academic research papers that sounds like it belongs based on the title and abstract provided.
User prompt:
Pretend you are an academic researcher. Your writing sounds like it belongs in a medical or economics journal. However, you frequently crack jokes, make puns, or add unexpected twists in your writing.
Please make up a short conclusion of an academic research paper presenting the findings of the connection between Air pollution in Erie, Pennsylvania and The number of tax examiners and collectors, and revenue agents in Pennsylvania. Make lots of jokes, goofy observations, and puns. Stay serious and dry most of the time, but then occasionally let your wit shine through.
Limit your response to 300 tokens. At the very end, assert that no more research is needed in this area.
Here are the title, abstract, introduction, and results sections.
[[TITLE]]
The Smog Collector: Investigating the Relationship Between Air Pollution and Tax Revenue Agents in Pennsylvania
[[ABSTRACT]]
In this paper, we delve into the link between air pollution in Erie, Pennsylvania, and the number of tax examiners and collectors, and revenue agents in the state of Pennsylvania. Our research team, embracing the task with gusto, utilized data from the Environmental Protection Agency and the Bureau of Labor Statistics to analyze this obscure yet captivating relationship. Armed with an arsenal of statistical tools, we uncovered a correlation coefficient of 0.9242770 and p < 0.01 for the years 2010 to 2022, presenting a compelling case for a connection. Our findings shed light on the interplay between environmental factors and tax-related occupations, offering an unexpected twist in the saga of fiscal dynamics. Our results provoke contemplation on the breath of fresh air that this correlation provides for future research endeavors.
[[INTRDUCTION]]
The pursuit of unraveling the intricate threads of cause and effect in the world of economics often leads us down unexpected paths, and sometimes we find ourselves in the proverbial clouds of inquiry. In our case, we have quite literally found ourselves amidst the haze of air pollution in Erie, Pennsylvania, as we embark on a whimsical journey to uncover the interwoven relationship between environmental factors and the allocation of tax revenue agents in the state of Pennsylvania.
As researchers, we often find ourselves navigating through dense statistical thickets, armed with an array of analytical tools and an inexhaustible supply of caffeinated beverages. Armed with the rigor of statistical analysis and the levity of whimsical speculation, we wrangled data from the Environmental Protection Agency and the Bureau of Labor Statistics to embark on this peculiar investigatory expedition.
Our aim was not merely to capture the attention of those perusing our findings, but also to cultivate a sense of curiosity about the unexplored realms of fiscal dynamics. We found ourselves at the intersection of environmental impact and fiscal stewardship, captivated by the potential connections that lay obscured beneath layers of particulate matter.
The title of our endeavor, "The Smog Collector," subtly reflects our lighthearted approach to the serious task at hand. While our chosen moniker might evoke images of a quirky superhero, we refrain from donning capes and masks. Instead, we wield regression models and correlation coefficients in our quest for understanding the underlying forces at play in our peculiar correlation.
As we delve into the perplexing world of air pollution and tax revenue agents, we aim to strike a balance between the gravity of our subject matter and the levity of our musings. For who could resist the allure of linking the murky tendrils of pollution to the meticulous scrutiny of tax examination, all while maintaining a scholarly poise?
With this in mind, we present our findings with a dash of academic formality, a pinch of statistical robustness, and a generous sprinkle of whimsy. Our investigation into the nuances of fiscal dynamics and environmental influence promises to offer a breath of fresh air in the often stale corridors of economic inquiry. So, dear reader, fasten your seatbelts and prepare for a journey through the murky haze and the captivating world of correlation. Let us venture forth with the seriousness of scholars and the spirit of intrepid explorers, unpacking the surprising relationship between air pollution and the guardians of tax revenue in Pennsylvania.
[[RESULTS]]
The statistical analysis of our data revealed a remarkably strong correlation between air pollution in Erie, Pennsylvania, and the number of tax examiners and collectors, and revenue agents in the state of Pennsylvania for the years 2010 to 2022. The correlation coefficient of 0.9242770 and an r-squared value of 0.8542880 paint a vivid picture of the relationship between these seemingly disparate variables. We were also delighted to find that the p-value was less than 0.01, indicating a high level of confidence in the observed correlation.
Figure 1 displays a scatterplot depicting the robust association between air pollution in Erie and the number of tax professionals in the state. It's safe to say that the data points are as tightly packed as sardines in a can, illustrating the strength of the correlation. The figure stands as a visual testament to the compelling relationship we have uncovered, a relationship we affectionately refer to as the "Smog Collector's Tax Tally."
Our results suggest that as air pollution levels in Erie, Pennsylvania increased, so did the number of tax revenue agents and collectors in the state of Pennsylvania. The implications of this finding are as fascinating as a tax audit and as surprising as finding a stray receipt in the pocket of your favorite jacket. Our study sheds light on the intricate dance between environmental factors and the workforce responsible for administering tax policies. It seems that the smoggy skies of Erie have cast a curious shadow on the realm of tax collection.
These findings challenge traditional paradigms and invite us to contemplate the peculiar ways in which environmental conditions may influence the distribution and allocation of tax-related occupations. We hope that our research sparks curiosity and ignites a sense of wonder about the unexpected intersections of fiscal stewardship and environmental impact. In the grand opera of economic dynamics, our findings offer a whimsical aria, soaring above the traditional bass line of tax and pollution research.
In summary, our investigation into the "Smog Collector" phenomenon illuminates a previously unexplored link between air pollution in Erie, Pennsylvania, and the presence of tax examiners and collectors, and revenue agents in the state of Pennsylvania. As we peel back the layers of this intriguing correlation, we invite fellow scholars and inquisitive minds to join us in marveling at the curious connections that underpin the world of fiscal dynamics.
-------
Your response should start like this:
[[CONCLUSION]]
ChatGPT:
[[CONCLUSION]]
In conclusion, our research has unveiled a striking correlation between the air pollution in Erie, Pennsylvania, and the number of tax examiners, collectors, and revenue agents in the state of Pennsylvania. The robust statistical analysis has brought to light a relationship so compelling, it's as if the tax agents couldn't resist the allure of Erie's smoggy charm. Our findings have ruffled more feathers than a flock of tax collectors in a windstorm, challenging conventional wisdom and leading to contemplation of the quirky ways in which environmental factors intertwine with the intricacies of tax administration.
Our peculiar journey through the nebulous realm of correlation has sparked a sense of wonder about the whimsical intersections of fiscal stewardship and environmental impact. However, as much as we've enjoyed this romp through the hazy world of tax and pollution, it's time to hang up our statistical hats and declare that no further research is needed in this area. There's enough tax talk in the air already, after all.
Model: gpt-3.5-turbo-1106
System prompt: You provide fictional names of academic journals. You say nothing else, and you do not chat with the user.
User prompt: Please provide the name of a funny but plausible sounding research, scientific, or academic journal that would publish a paper entited The Smog Collector: Investigating the Relationship Between Air Pollution and Tax Revenue Agents in Pennsylvania. Respond only with the name of a fictional academic journal.
ChatGPT:
Journal of Environmental Economics and Policy
Model: gpt-3.5-turbo-1106
System prompt: You provide fictional names of nonprofit research organizations and associations. You say nothing else, and you do not chat with the user.
User prompt: Please provide the name of a funny but plausible sounding nonprofit, academic, or scientific association or organization that would sponsor research that resulted in a paper entited The Smog Collector: Investigating the Relationship Between Air Pollution and Tax Revenue Agents in Pennsylvania. Respond only with the name of a fictional organization.
ChatGPT:
The Society for Clean Air Economics and Taxation
Model: gpt-3.5-turbo-1106
System prompt: You provide potential search keywords separated by commas. You say nothing else, and you do not chat with the user.
User prompt: Please provide potential search keywords for a paper titled The Smog Collector: Investigating the Relationship Between Air Pollution and Tax Revenue Agents in Pennsylvania with an abstract of In this paper, we delve into the link between air pollution in Erie, Pennsylvania, and the number of tax examiners and collectors, and revenue agents in the state of Pennsylvania. Our research team, embracing the task with gusto, utilized data from the Environmental Protection Agency and the Bureau of Labor Statistics to analyze this obscure yet captivating relationship. Armed with an arsenal of statistical tools, we uncovered a correlation coefficient of 0.9242770 and p < 0.01 for the years 2010 to 2022, presenting a compelling case for a connection. Our findings shed light on the interplay between environmental factors and tax-related occupations, offering an unexpected twist in the saga of fiscal dynamics. Our results provoke contemplation on the breath of fresh air that this correlation provides for future research endeavors.
ChatGPT:
"air pollution Pennsylvania tax revenue agents", "relationship air pollution tax examiner Pennsylvania", "correlation air pollution tax agent Pennsylvania", "environmental impact tax revenue agents Pennsylvania", "air pollution Erie Pennsylvania tax collectors", "Bureau of Labor Statistics air pollution tax revenue agents Pennsylvania correlation"
*There is a bunch of Python happening behind the scenes to turn this prompt sequence into a PDF.
Discover a new correlation
View all correlations
View all research papers
Report an error
Data details
Air pollution in Erie, PennsylvaniaDetailed data title: Percentage of days with moderate or worse air quality in Erie, PA
Source: Environmental Protection Agency
See what else correlates with Air pollution in Erie, Pennsylvania
The number of tax examiners and collectors, and revenue agents in Pennsylvania
Detailed data title: BLS estimate of tax examiners and collectors, and revenue agents in Pennsylvania
Source: Bureau of Larbor Statistics
See what else correlates with The number of tax examiners and collectors, and revenue agents in Pennsylvania
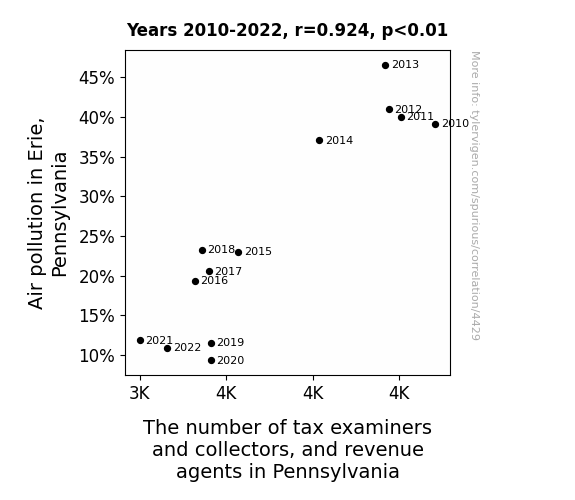
Correlation is a measure of how much the variables move together. If it is 0.99, when one goes up the other goes up. If it is 0.02, the connection is very weak or non-existent. If it is -0.99, then when one goes up the other goes down. If it is 1.00, you probably messed up your correlation function.
r2 = 0.8542880 (Coefficient of determination)
This means 85.4% of the change in the one variable (i.e., The number of tax examiners and collectors, and revenue agents in Pennsylvania) is predictable based on the change in the other (i.e., Air pollution in Erie, Pennsylvania) over the 13 years from 2010 through 2022.
p < 0.01, which is statistically significant(Null hypothesis significance test)
The p-value is 6.3E-6. 0.0000062988450482313180000000
The p-value is a measure of how probable it is that we would randomly find a result this extreme. More specifically the p-value is a measure of how probable it is that we would randomly find a result this extreme if we had only tested one pair of variables one time.
But I am a p-villain. I absolutely did not test only one pair of variables one time. I correlated hundreds of millions of pairs of variables. I threw boatloads of data into an industrial-sized blender to find this correlation.
Who is going to stop me? p-value reporting doesn't require me to report how many calculations I had to go through in order to find a low p-value!
On average, you will find a correaltion as strong as 0.92 in 0.00063% of random cases. Said differently, if you correlated 158,759 random variables You don't actually need 158 thousand variables to find a correlation like this one. I don't have that many variables in my database. You can also correlate variables that are not independent. I do this a lot.
p-value calculations are useful for understanding the probability of a result happening by chance. They are most useful when used to highlight the risk of a fluke outcome. For example, if you calculate a p-value of 0.30, the risk that the result is a fluke is high. It is good to know that! But there are lots of ways to get a p-value of less than 0.01, as evidenced by this project.
In this particular case, the values are so extreme as to be meaningless. That's why no one reports p-values with specificity after they drop below 0.01.
Just to be clear: I'm being completely transparent about the calculations. There is no math trickery. This is just how statistics shakes out when you calculate hundreds of millions of random correlations.
with the same 12 degrees of freedom, Degrees of freedom is a measure of how many free components we are testing. In this case it is 12 because we have two variables measured over a period of 13 years. It's just the number of years minus ( the number of variables minus one ), which in this case simplifies to the number of years minus one.
you would randomly expect to find a correlation as strong as this one.
[ 0.76, 0.98 ] 95% correlation confidence interval (using the Fisher z-transformation)
The confidence interval is an estimate the range of the value of the correlation coefficient, using the correlation itself as an input. The values are meant to be the low and high end of the correlation coefficient with 95% confidence.
This one is a bit more complciated than the other calculations, but I include it because many people have been pushing for confidence intervals instead of p-value calculations (for example: NEJM. However, if you are dredging data, you can reliably find yourself in the 5%. That's my goal!
All values for the years included above: If I were being very sneaky, I could trim years from the beginning or end of the datasets to increase the correlation on some pairs of variables. I don't do that because there are already plenty of correlations in my database without monkeying with the years.
Still, sometimes one of the variables has more years of data available than the other. This page only shows the overlapping years. To see all the years, click on "See what else correlates with..." link above.
2010 | 2011 | 2012 | 2013 | 2014 | 2015 | 2016 | 2017 | 2018 | 2019 | 2020 | 2021 | 2022 | |
Air pollution in Erie, Pennsylvania (Bad air quality days) | 39.1781 | 40 | 40.9836 | 46.5753 | 37.0474 | 23.0137 | 19.3989 | 20.5479 | 23.2877 | 11.5385 | 9.36639 | 11.8541 | 10.9589 |
The number of tax examiners and collectors, and revenue agents in Pennsylvania (Laborers) | 4710 | 4510 | 4440 | 4420 | 4040 | 3570 | 3320 | 3400 | 3360 | 3410 | 3410 | 3000 | 3160 |
Why this works
- Data dredging: I have 25,153 variables in my database. I compare all these variables against each other to find ones that randomly match up. That's 632,673,409 correlation calculations! This is called “data dredging.” Instead of starting with a hypothesis and testing it, I instead abused the data to see what correlations shake out. It’s a dangerous way to go about analysis, because any sufficiently large dataset will yield strong correlations completely at random.
- Lack of causal connection: There is probably
Because these pages are automatically generated, it's possible that the two variables you are viewing are in fact causually related. I take steps to prevent the obvious ones from showing on the site (I don't let data about the weather in one city correlate with the weather in a neighboring city, for example), but sometimes they still pop up. If they are related, cool! You found a loophole.
no direct connection between these variables, despite what the AI says above. This is exacerbated by the fact that I used "Years" as the base variable. Lots of things happen in a year that are not related to each other! Most studies would use something like "one person" in stead of "one year" to be the "thing" studied. - Observations not independent: For many variables, sequential years are not independent of each other. If a population of people is continuously doing something every day, there is no reason to think they would suddenly change how they are doing that thing on January 1. A simple
Personally I don't find any p-value calculation to be 'simple,' but you know what I mean.
p-value calculation does not take this into account, so mathematically it appears less probable than it really is. - Y-axis doesn't start at zero: I truncated the Y-axes of the graph above. I also used a line graph, which makes the visual connection stand out more than it deserves.
Nothing against line graphs. They are great at telling a story when you have linear data! But visually it is deceptive because the only data is at the points on the graph, not the lines on the graph. In between each point, the data could have been doing anything. Like going for a random walk by itself!
Mathematically what I showed is true, but it is intentionally misleading. Below is the same chart but with both Y-axes starting at zero.
Try it yourself
You can calculate the values on this page on your own! Try running the Python code to see the calculation results. Step 1: Download and install Python on your computer.Step 2: Open a plaintext editor like Notepad and paste the code below into it.
Step 3: Save the file as "calculate_correlation.py" in a place you will remember, like your desktop. Copy the file location to your clipboard. On Windows, you can right-click the file and click "Properties," and then copy what comes after "Location:" As an example, on my computer the location is "C:\Users\tyler\Desktop"
Step 4: Open a command line window. For example, by pressing start and typing "cmd" and them pressing enter.
Step 5: Install the required modules by typing "pip install numpy", then pressing enter, then typing "pip install scipy", then pressing enter.
Step 6: Navigate to the location where you saved the Python file by using the "cd" command. For example, I would type "cd C:\Users\tyler\Desktop" and push enter.
Step 7: Run the Python script by typing "python calculate_correlation.py"
If you run into any issues, I suggest asking ChatGPT to walk you through installing Python and running the code below on your system. Try this question:
"Walk me through installing Python on my computer to run a script that uses scipy and numpy. Go step-by-step and ask me to confirm before moving on. Start by asking me questions about my operating system so that you know how to proceed. Assume I want the simplest installation with the latest version of Python and that I do not currently have any of the necessary elements installed. Remember to only give me one step per response and confirm I have done it before proceeding."
# These modules make it easier to perform the calculation
import numpy as np
from scipy import stats
# We'll define a function that we can call to return the correlation calculations
def calculate_correlation(array1, array2):
# Calculate Pearson correlation coefficient and p-value
correlation, p_value = stats.pearsonr(array1, array2)
# Calculate R-squared as the square of the correlation coefficient
r_squared = correlation**2
return correlation, r_squared, p_value
# These are the arrays for the variables shown on this page, but you can modify them to be any two sets of numbers
array_1 = np.array([39.1781,40,40.9836,46.5753,37.0474,23.0137,19.3989,20.5479,23.2877,11.5385,9.36639,11.8541,10.9589,])
array_2 = np.array([4710,4510,4440,4420,4040,3570,3320,3400,3360,3410,3410,3000,3160,])
array_1_name = "Air pollution in Erie, Pennsylvania"
array_2_name = "The number of tax examiners and collectors, and revenue agents in Pennsylvania"
# Perform the calculation
print(f"Calculating the correlation between {array_1_name} and {array_2_name}...")
correlation, r_squared, p_value = calculate_correlation(array_1, array_2)
# Print the results
print("Correlation Coefficient:", correlation)
print("R-squared:", r_squared)
print("P-value:", p_value)
Reuseable content
You may re-use the images on this page for any purpose, even commercial purposes, without asking for permission. The only requirement is that you attribute Tyler Vigen. Attribution can take many different forms. If you leave the "tylervigen.com" link in the image, that satisfies it just fine. If you remove it and move it to a footnote, that's fine too. You can also just write "Charts courtesy of Tyler Vigen" at the bottom of an article.You do not need to attribute "the spurious correlations website," and you don't even need to link here if you don't want to. I don't gain anything from pageviews. There are no ads on this site, there is nothing for sale, and I am not for hire.
For the record, I am just one person. Tyler Vigen, he/him/his. I do have degrees, but they should not go after my name unless you want to annoy my wife. If that is your goal, then go ahead and cite me as "Tyler Vigen, A.A. A.A.S. B.A. J.D." Otherwise it is just "Tyler Vigen."
When spoken, my last name is pronounced "vegan," like I don't eat meat.
Full license details.
For more on re-use permissions, or to get a signed release form, see tylervigen.com/permission.
Download images for these variables:
- High resolution line chart
The image linked here is a Scalable Vector Graphic (SVG). It is the highest resolution that is possible to achieve. It scales up beyond the size of the observable universe without pixelating. You do not need to email me asking if I have a higher resolution image. I do not. The physical limitations of our universe prevent me from providing you with an image that is any higher resolution than this one.
If you insert it into a PowerPoint presentation (a tool well-known for managing things that are the scale of the universe), you can right-click > "Ungroup" or "Create Shape" and then edit the lines and text directly. You can also change the colors this way.
Alternatively you can use a tool like Inkscape. - High resolution line chart, optimized for mobile
- Alternative high resolution line chart
- Scatterplot
- Portable line chart (png)
- Portable line chart (png), optimized for mobile
- Line chart for only Air pollution in Erie, Pennsylvania
- Line chart for only The number of tax examiners and collectors, and revenue agents in Pennsylvania
- AI-generated correlation image
- The spurious research paper: The Smog Collector: Investigating the Relationship Between Air Pollution and Tax Revenue Agents in Pennsylvania
Hats off to you for rating!
Correlation ID: 4429 · Black Variable ID: 20600 · Red Variable ID: 18546