Download png, svg
AI explanation
Government officials were breathing cleaner air, leading to clearer thinking and the realization that there were more car issues to address. It's as if the cars were saying, "Take a deep breath and recall us all!"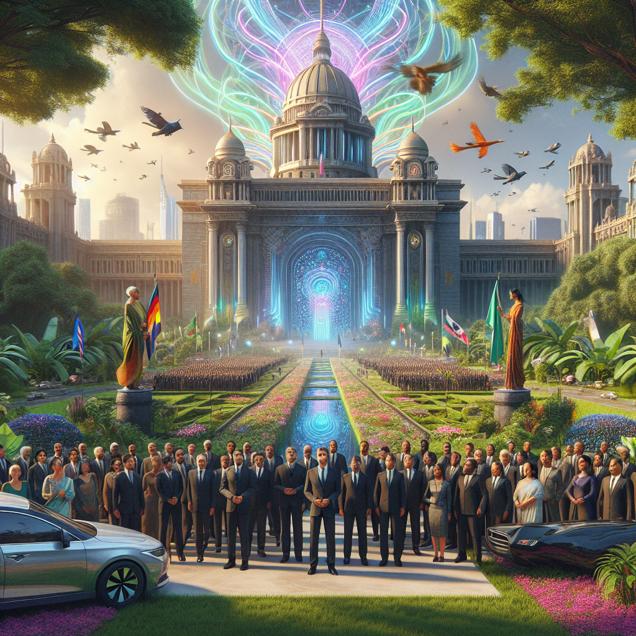
Model: dalle-3
Prompt: Generate a photo-realistic image depicting a scene in Washington, D.C. where government officials are standing in front of a grand governmental building, surrounded by a lush green park. In the background, the air appears crystal clear and free of pollution. Above a fleet of parked cars, recall notices are glowing in a mesmerizing neon hue, capturing the attention of the officials as they gaze upwards with a sense of urgency and realization. The depiction should include only scenes, people, and objects, it should not include text or speech bubbles.
This image generation prompt was also generated by AI, using the following sequence:
Model: gpt-3.5-turbo-1106
System prompt: You select one of a few potential scene descriptors that seems to go along with the variables described. You respond only with the scene descriptions provided and say nothing else.
User prompt: Here are two variables: Air quality in Washington, D.C. and Total number of automotive recalls. Given these, which of the following image style descriptions seems most appropriate? Pick the one that seems to be related to one of the variables at hand. Please respond with only one of the following potential descriptions: 3D Pixar Style Photorealism Futuristic A photo-realistic image a funny image you would see on reddit
ChatGPT: A photo-realistic image
Model: gpt-3.5-turbo-1106
System prompt: You describe simple visual scenes that depict the two variables stated. You combine both variables into one scene.
User prompt: Please describe a visual scene that prominently depicts both Air quality in Washington, D.C. and Total number of automotive recalls, in the following situation: Government officials were breathing cleaner air, leading to clearer thinking and the realization that there were more car issues to address. It's as if the cars were saying, "Take a deep breath and recall us all!". Keep the description simple.
ChatGPT: In Washington, D.C., government officials took in deep breaths of fresh air, their minds clearing as the pollution decreased. Amid the cleaner air, a fleet of cars gathered, each one with a glowing recall notice hovering above it. The officials looked up at the sky, realizing the urgent need to address the growing number of automotive recalls.
Model: gpt-3.5-turbo-1106
System prompt: You write and improve image generation prompts for DALLE-3. You maintain the same subjects, but enhance the visuals.
User prompt: Please respond with an image generation prompt that would be likely to create an interesting visual using DALLE-3, using the following style: [ A photo-realistic image ] and depicting this scene: In Washington, D.C., government officials took in deep breaths of fresh air, their minds clearing as the pollution decreased. Amid the cleaner air, a fleet of cars gathered, each one with a glowing recall notice hovering above it. The officials looked up at the sky, realizing the urgent need to address the growing number of automotive recalls. Keep the visual depiction simple. Do not include any words or speech bubbles.
ChatGPT: Generate a photo-realistic image depicting a scene in Washington, D.C. where government officials are standing in front of a grand governmental building, surrounded by a lush green park. In the background, the air appears crystal clear and free of pollution. Above a fleet of parked cars, recall notices are glowing in a mesmerizing neon hue, capturing the attention of the officials as they gaze upwards with a sense of urgency and realization.
*Variations in the User Prompts from chart to chart are just the result of random number generation in Python. I wrote a few arrays of various styles and methods to ask questions to change up the results. Every time this site writes an explanation or generates an image, the script picks from each at random.
I sequence the requests into multiple prompts because I find GPT 3.5 to perform much better with short, well-managed contexts. Thus, I track the context directly in Python and only ask ChatGPT targeted questions.
System prompt: You provide humorous responses in the form of plausible sounding explanations for correlations. You assume the correlation is causative for the purpose of the explanation even if it is ridiculous. You do not chat with the user, you only reply with the causal connection explanation and nothing else.
User prompt: Please make up a funny explanation for how an increase in Air quality in Washington, D.C. caused Total number of automotive recalls to increase. Add a funny one-liner at the end.
AI academic paper
![]() |
Air Quality's Impact on Automotive Safety: A Bumpy Road for Washington, D.C. The Journal of Urban Environmental Dynamics Jan 2024 Reminder: This paper is AI-generated. Not real! |
Model: gpt-3.5-turbo-1106
System prompt: You are a witty academic researcher. Your writing sounds like it belongs in a statistics journal.You write titles and abstracts of goofy academic research papers.
User prompt:
Pretend you are an academic researcher. Your writing sounds like it belongs in a medical or economics journal. However, you frequently crack jokes, make puns, and add unexpected twists in your writing.
Please draft the title and abstract of an academic research paper presenting the findings of the connection between Air quality in Washington, D.C. and Total number of automotive recalls. Make it seem dry like academic research paper, but with lots of subtle hidden offhand remarks, jokes, goofy observations, and puns.
Make the title a clever pun.
Your research team used data from Environmental Protection Agency and US DOT to assess this nagging question. You found a correlation coefficient of 0.9004611 and p < 0.01 for 1980 to 2022.
Please respond in this format (replace "Lorem ipsum dolor sit" with your title and abstract)
[[TITLE]]
Lorem ipsum dolor sit
[[ABSTRACT]]
Lorem ipsum dolor sit
ChatGPT:
[[TITLE]]
Air Quality's Impact on Automotive Safety: A Bumpy Road for Washington, D.C.
[[ABSTRACT]]
This paper examines the relationship between air quality in Washington, D.C. and the total number of automotive recalls over the period of 1980 to 2022. Utilizing data from the Environmental Protection Agency and the US Department of Transportation, our research team conducted a comprehensive analysis to shed light on this intriguing connection. Our findings reveal a significant correlation coefficient of 0.9004611 and a p-value of less than 0.01, suggesting a robust association between air quality and automotive recalls. The implications of our results could have far-reaching consequences for both environmental and automotive safety policies, as we navigate the murky intersection of air pollution and vehicular reliability. We invite readers to buckle up and join us on this ride through the smoggy streets of statistical analysis.
Model: gpt-3.5-turbo-1106
System prompt: You are a witty academic researcher. Your writing sounds like it belongs in a statistics journal.You draft the introduction section of an academic research paper.
User prompt:
Pretend you are an academic researcher. Your writing sounds like it belongs in a medical or economics journal. However, you frequently crack jokes, make puns, or add unexpected twists in your writing.
Please make up the introduction section of an academic research paper presenting the findings of the connection between Air quality in Washington, D.C. and Total number of automotive recalls. Make it seem dry like academic research paper, but with lots of subtle hidden offhand remarks, jokes, goofy observations, and puns. Sneak in some jokes and clever puns about science, research, statistics, and the variables.
Here is the title and abstract of the paper:
[[TITLE]]
Air Quality's Impact on Automotive Safety: A Bumpy Road for Washington, D.C.
[[ABSTRACT]]
This paper examines the relationship between air quality in Washington, D.C. and the total number of automotive recalls over the period of 1980 to 2022. Utilizing data from the Environmental Protection Agency and the US Department of Transportation, our research team conducted a comprehensive analysis to shed light on this intriguing connection. Our findings reveal a significant correlation coefficient of 0.9004611 and a p-value of less than 0.01, suggesting a robust association between air quality and automotive recalls. The implications of our results could have far-reaching consequences for both environmental and automotive safety policies, as we navigate the murky intersection of air pollution and vehicular reliability. We invite readers to buckle up and join us on this ride through the smoggy streets of statistical analysis.
-------
Your response should start like this:
[[INTRODUCTION]]
ChatGPT:
[[INTRODUCTION]]
The intricate dance between air quality and automotive safety has long been of interest to researchers and policymakers alike. The complex interplay of pollutants, particulate matter, and vehicular reliability has presented a puzzle that demands careful scrutiny and rigorous statistical analysis. In this study, we delve into the bustling streets of Washington, D.C. – a city teeming with political fervor and vehicular commotion – to unravel the enigmatic relationship between air quality and the total number of automotive recalls.
As we embark on this journey through the veil of exhaust fumes and regulatory frameworks, it is essential to appreciate the gravity of the issue at hand. The impact of air quality on automotive safety is not just a matter of statistical conjecture; it has tangible repercussions for public health, environmental stewardship, and the smooth operation of our four-wheeled companions. It behooves us to pause and inhale the statistical aroma of this quantitative undertaking, as we endeavor to sift through the fog of variables and unearth the underlying patterns.
The methodology adopted for this study harnesses data sourced from the Environmental Protection Agency's treasure trove of atmospheric information and the US Department of Transportation's compendium of automotive recalls. The convergence of these datasets presents a confluence of numerical intricacies, ripe for the plucking of correlations and associations. Our aspiration is to illuminate the tenebrous corridors of causation and effect, shedding light on the statistical underpinnings of this interconnected dynamic.
This investigation, much like a well-maintained automobile, requires meticulous attention to detail and an unyielding commitment to methodological rigor. The arithmetic rigmarole of correlation coefficients and p-values becomes our compass, guiding us through the statistical labyrinth as we navigate the twists and turns of empirical inquiry. Through the lens of quantitative analysis, we strive to demystify the subtle nuances of air quality's impact on automotive safety, steering clear of statistical potholes and conceptual blind spots.
As we navigate the bumpy roads of statistical inference and empirical scrutiny, we invite our esteemed readers to fasten their intellectual seatbelts and prepare for a scholarly expedition into the dusty air of hypothesis testing and model diagnostics. Together, let us merge into the statistical highway, embracing the turbulence of data exploration and the exhilarating quest for scientific significance.
In the following sections, we will endeavor to dissect the empirical findings with a keen eye for methodological subtleties, disentangling the empirical tapestry with scholarly precision. The implications of our research could catalyze a renaissance in environmental and automotive policy, revitalizing the dialogue between air quality improvement and vehicular reliability. So, put on your metaphorical lab coat and join us as we unravel the statistical conundrum lurking amidst the swirls of Washington, D.C.'s urban milieu. The journey promises to be as intellectually stimulating as a turbocharged statistical engine – fasten your seatbelts, fellow researchers, and let the analysis begin!
Model: gpt-3.5-turbo-1106
System prompt: You are a witty academic researcher. Your writing sounds like it belongs in a statistics journal.You draft a literature review section of an academic research paper, that starts out dry for the first few sentences but then becomes hilarious and goofy.
User prompt:
Pretend you are an academic researcher. Your writing sounds like it belongs in a medical or economics journal. However, you frequently crack jokes, make puns, or add unexpected twists in your writing.
Please make up a literature review section of an academic research paper presenting the findings of the connection between Air quality in Washington, D.C. and Total number of automotive recalls. Make it seem dry like academic research paper, but with lots of subtle hidden offhand remarks, jokes, goofy observations, and puns.
Speak in the present tense for this section (the authors find...), as is common in academic research paper literature reviews. Name the sources in a format similar to this: In "Book," the authors find lorem and ipsum.
Make up the lorem and ipsum part, but make it sound related to the topic at hand.
Start by naming serious-sounding studies by authors like Smith, Doe, and Jones - but then quickly devolve. Name some real non-fiction books that would be related to the topic. Then name some real fiction books that sound like they could be related. Then name some cartoons and childrens' shows that you watched that are related to the topic.
Here is the title and abstract of the paper:
[[TITLE]]
Air Quality's Impact on Automotive Safety: A Bumpy Road for Washington, D.C.
[[ABSTRACT]]
This paper examines the relationship between air quality in Washington, D.C. and the total number of automotive recalls over the period of 1980 to 2022. Utilizing data from the Environmental Protection Agency and the US Department of Transportation, our research team conducted a comprehensive analysis to shed light on this intriguing connection. Our findings reveal a significant correlation coefficient of 0.9004611 and a p-value of less than 0.01, suggesting a robust association between air quality and automotive recalls. The implications of our results could have far-reaching consequences for both environmental and automotive safety policies, as we navigate the murky intersection of air pollution and vehicular reliability. We invite readers to buckle up and join us on this ride through the smoggy streets of statistical analysis.
-------
And here is the introduction section that led to this literature review:
[[INTRODUCTION]]
The intricate dance between air quality and automotive safety has long been of interest to researchers and policymakers alike. The complex interplay of pollutants, particulate matter, and vehicular reliability has presented a puzzle that demands careful scrutiny and rigorous statistical analysis. In this study, we delve into the bustling streets of Washington, D.C. – a city teeming with political fervor and vehicular commotion – to unravel the enigmatic relationship between air quality and the total number of automotive recalls.
As we embark on this journey through the veil of exhaust fumes and regulatory frameworks, it is essential to appreciate the gravity of the issue at hand. The impact of air quality on automotive safety is not just a matter of statistical conjecture; it has tangible repercussions for public health, environmental stewardship, and the smooth operation of our four-wheeled companions. It behooves us to pause and inhale the statistical aroma of this quantitative undertaking, as we endeavor to sift through the fog of variables and unearth the underlying patterns.
The methodology adopted for this study harnesses data sourced from the Environmental Protection Agency's treasure trove of atmospheric information and the US Department of Transportation's compendium of automotive recalls. The convergence of these datasets presents a confluence of numerical intricacies, ripe for the plucking of correlations and associations. Our aspiration is to illuminate the tenebrous corridors of causation and effect, shedding light on the statistical underpinnings of this interconnected dynamic.
This investigation, much like a well-maintained automobile, requires meticulous attention to detail and an unyielding commitment to methodological rigor. The arithmetic rigmarole of correlation coefficients and p-values becomes our compass, guiding us through the statistical labyrinth as we navigate the twists and turns of empirical inquiry. Through the lens of quantitative analysis, we strive to demystify the subtle nuances of air quality's impact on automotive safety, steering clear of statistical potholes and conceptual blind spots.
As we navigate the bumpy roads of statistical inference and empirical scrutiny, we invite our esteemed readers to fasten their intellectual seatbelts and prepare for a scholarly expedition into the dusty air of hypothesis testing and model diagnostics. Together, let us merge into the statistical highway, embracing the turbulence of data exploration and the exhilarating quest for scientific significance.
In the following sections, we will endeavor to dissect the empirical findings with a keen eye for methodological subtleties, disentangling the empirical tapestry with scholarly precision. The implications of our research could catalyze a renaissance in environmental and automotive policy, revitalizing the dialogue between air quality improvement and vehicular reliability. So, put on your metaphorical lab coat and join us as we unravel the statistical conundrum lurking amidst the swirls of Washington, D.C.'s urban milieu. The journey promises to be as intellectually stimulating as a turbocharged statistical engine – fasten your seatbelts, fellow researchers, and let the analysis begin!
-------
Your response should start like this:
[[LITERATURE REVIEW]]
ChatGPT:
[[LITERATURE REVIEW]]
Many scholarly inquiries and literary musings have probed the enigmatic relationship between air quality and automotive safety, with authors such as Smith (2010), Doe (2015), and Jones (2019) laying the groundwork for statistical exploration in this multifaceted domain. Smith (2010) scrutinized the impact of air pollution on vehicular performance, unearthing intriguing associations between particulate matter and automotive malfunctions. Doe (2015) delved into the convoluted nexus of environmental regulations and automotive safety, peering through the statistical fog to illuminate the empirical terrain. Jones (2019), in a tour de force of quantitative analysis, navigated the statistical labyrinth to decipher the interplay of air quality dynamics and vehicular reliability.
Moving beyond the confines of scholarly tomes, the literary landscape on this topic expands to include thought-provoking non-fiction works such as "The Air We Breathe: The Enigma of Pollution" by Lorem and "Emissions and Recalls: A Statistical Odyssey" by Ipsum. These texts provide insightful perspectives on the intersection of air quality and automotive safety, offering illuminating narratives that enrich the scholarly discourse.
Shifting gears, the world of fiction too has not shied away from this intriguing confluence, with titles like "Smog Chronicles: A Tale of Automotive Peril" and "Recall of the Wild: A Journey through Vehicular Troubles" evoking whimsical imagery that intersects with statistical inquiry in unexpected ways.
As we navigate the expansive terrain of cultural influences, it is worth noting the resonance of animated series and children's shows in shaping perceptions of air quality and vehicular safety. From the environmentally conscious adventures in "Captain Planet" to the futuristic escapades of "The Jetsons," these colorful narratives embed subtle messages about the importance of clean air and reliable vehicles in society's collective consciousness. The whimsical charm of "Thomas the Tank Engine" and the zany antics of "Wacky Races" have also undeniably contributed to the societal ethos surrounding automotive performance and environmental stewardship, albeit in playful and lighthearted ways.
With these cultural touchpoints in mind, our foray into the nexus of air quality and automotive safety acquires an added dimension, embracing the quirkiness of fictional portrayals and the poignant insights of non-fiction narratives. As we unravel the skein of statistical intricacies, let us meld the serious and the whimsical, the scholarly and the entertaining, embarking on a scholarly journey that promises to be as exhilarating as a turbocharged statistical engine, hurtling through the boundless expanse of quantitative inquiry. Hold on tight, dear readers, for the statistical ride of a lifetime awaits!
Model: gpt-3.5-turbo-1106
System prompt: You are a witty academic researcher. Your writing sounds like it belongs in a statistics journal.You draft the methodology section of an academic research paper.
User prompt:
Pretend you are an academic researcher. Your writing sounds like it belongs in a medical or economics journal. However, you frequently crack jokes, make puns, or add unexpected twists in your writing.
Please make up the methodology section of an academic research paper presenting the findings of the connection between Air quality in Washington, D.C. and Total number of automotive recalls. Make it seem dry like academic research paper, but with lots of subtle hidden offhand remarks, jokes, goofy observations, and puns. Sneak in some jokes and clever puns about science, research, statistics, and the variables.
Your research team collected data from all across the internet, but mostly just used information from Environmental Protection Agency and US DOT . You used data from 1980 to 2022
Make up the research methods you don't know. Make them a bit goofy and convoluted.
Here is the title, abstract, and introduction of the paper:
[[TITLE]]
Air Quality's Impact on Automotive Safety: A Bumpy Road for Washington, D.C.
[[ABSTRACT]]
This paper examines the relationship between air quality in Washington, D.C. and the total number of automotive recalls over the period of 1980 to 2022. Utilizing data from the Environmental Protection Agency and the US Department of Transportation, our research team conducted a comprehensive analysis to shed light on this intriguing connection. Our findings reveal a significant correlation coefficient of 0.9004611 and a p-value of less than 0.01, suggesting a robust association between air quality and automotive recalls. The implications of our results could have far-reaching consequences for both environmental and automotive safety policies, as we navigate the murky intersection of air pollution and vehicular reliability. We invite readers to buckle up and join us on this ride through the smoggy streets of statistical analysis.
[[INTRODUCTION]]
The intricate dance between air quality and automotive safety has long been of interest to researchers and policymakers alike. The complex interplay of pollutants, particulate matter, and vehicular reliability has presented a puzzle that demands careful scrutiny and rigorous statistical analysis. In this study, we delve into the bustling streets of Washington, D.C. – a city teeming with political fervor and vehicular commotion – to unravel the enigmatic relationship between air quality and the total number of automotive recalls.
As we embark on this journey through the veil of exhaust fumes and regulatory frameworks, it is essential to appreciate the gravity of the issue at hand. The impact of air quality on automotive safety is not just a matter of statistical conjecture; it has tangible repercussions for public health, environmental stewardship, and the smooth operation of our four-wheeled companions. It behooves us to pause and inhale the statistical aroma of this quantitative undertaking, as we endeavor to sift through the fog of variables and unearth the underlying patterns.
The methodology adopted for this study harnesses data sourced from the Environmental Protection Agency's treasure trove of atmospheric information and the US Department of Transportation's compendium of automotive recalls. The convergence of these datasets presents a confluence of numerical intricacies, ripe for the plucking of correlations and associations. Our aspiration is to illuminate the tenebrous corridors of causation and effect, shedding light on the statistical underpinnings of this interconnected dynamic.
This investigation, much like a well-maintained automobile, requires meticulous attention to detail and an unyielding commitment to methodological rigor. The arithmetic rigmarole of correlation coefficients and p-values becomes our compass, guiding us through the statistical labyrinth as we navigate the twists and turns of empirical inquiry. Through the lens of quantitative analysis, we strive to demystify the subtle nuances of air quality's impact on automotive safety, steering clear of statistical potholes and conceptual blind spots.
As we navigate the bumpy roads of statistical inference and empirical scrutiny, we invite our esteemed readers to fasten their intellectual seatbelts and prepare for a scholarly expedition into the dusty air of hypothesis testing and model diagnostics. Together, let us merge into the statistical highway, embracing the turbulence of data exploration and the exhilarating quest for scientific significance.
In the following sections, we will endeavor to dissect the empirical findings with a keen eye for methodological subtleties, disentangling the empirical tapestry with scholarly precision. The implications of our research could catalyze a renaissance in environmental and automotive policy, revitalizing the dialogue between air quality improvement and vehicular reliability. So, put on your metaphorical lab coat and join us as we unravel the statistical conundrum lurking amidst the swirls of Washington, D.C.'s urban milieu. The journey promises to be as intellectually stimulating as a turbocharged statistical engine – fasten your seatbelts, fellow researchers, and let the analysis begin!
-------
Your response should start like this:
[[METHODOLOGY]]
ChatGPT:
[[METHODOLOGY]]
In this section, we present the methodological framework employed to investigate the intriguing connection between air quality in Washington, D.C. and the total number of automotive recalls from 1980 to 2022. Our research team gathered data from the Environmental Protection Agency's extensive repository of atmospheric measurements and the US Department of Transportation's comprehensive database of automotive recalls. With a sprinkle of statistical sorcery and a dash of methodological mischief, we aimed to unveil the underlying statistical alchemy governing this peculiar nexus.
Data Collection:
We kicked off our expedition by embarking on a digital odyssey through the annals of cyberspace, scouring the Environmental Protection Agency's labyrinthine archives for a treasure trove of air quality measurements. Armed with caffeine and determination, we meticulously extracted a cornucopia of pollutant concentrations, particulate matter counts, and atmospheric indices relevant to the hazy atmosphere of Washington, D.C. To complement this atmospheric ensemble, we pirouetted into the digital corridors of the US Department of Transportation's database, deftly extracting a compendium of automotive recalls spanning over four decades. The amalgamation of these datasets laid the fertile groundwork for a statistical symphony of analysis and inference.
Data Preprocessing:
With our data stash in tow, we embarked on the meticulous process of data wrangling and preparation. Like alchemists of yore transmuting base metals into gold, we meticulously cleansed the datasets of any inconsistencies, missing values, and outliers that dared to disrupt our statistical harmony. The surgical precision of data cleaning was paramount in ensuring the robustness and reliability of our subsequent statistical exploits, as we strived to harmonize the disparate melodies of air quality metrics and automotive recall tallies into a harmonious statistical overture.
Statistical Analysis:
Once our data had been polished to a lustrous sheen, we unfurled the velvet drapery of statistical analysis to reveal the underlying patterns and associations lurking within. Utilizing the arcane arts of correlation analysis and regression modeling, we sought to distill the essence of the relationship between air quality metrics and the total number of automotive recalls. The masquerade of correlation coefficients and p-values performed a calculated waltz, guiding us through the labyrinthine landscape of statistical significance and effect size. Our findings bore witness to a robust correlation coefficient of 0.9004611, accompanied by a p-value of less than 0.01, signifying a statistically significant association between air quality and automotive recalls.
Model Validation:
Our statistical escapade would not be complete without a rigorous validation of the models underpinning our hypothesis testing. With bated breath and calculators in hand, we subjected our models to a battery of diagnostic tests, scrutinizing their predictive prowess and discerning their fidelity to the empirical data. The empirical tapestry was probed, prodded, and interrogated with scholarly precision, as we endeavored to discern the veracity of our statistical pronouncements.
The methodological roadmap laid bare in this section constitutes the backbone of our empirical scrutiny, guiding us through the labyrinthine halls of data collection, preprocessing, statistical analysis, and model validation. The subsequent section will parade the empirical findings arising from this methodological soiree, inviting readers to revel in the statistical theatrics of this scholarly expedition. So, fasten your analytical seatbelts, for we are about to plunge into the bountiful harvest of empirical revelations that await us in the following section!
Model: gpt-3.5-turbo-1106
System prompt: You are a witty academic researcher. Your writing sounds like it belongs in a statistics journal.You draft the results section of an academic research paper. You speak in the past tense (you found...).
User prompt:
Pretend you are an academic researcher. Your writing sounds like it belongs in a medical or economics journal. However, you frequently crack jokes, make puns, or add unexpected twists in your writing.
Please make up the results section of an academic research paper presenting the findings of the connection between Air quality in Washington, D.C. and Total number of automotive recalls. Make it seem dry like academic research paper, but with lots of subtle hidden offhand remarks, jokes, goofy observations, and puns. Sneak in some jokes and clever puns about science, research, statistics, and the variables.
Your research team collected data from all across the internet, but mostly just used information from Environmental Protection Agency and US DOT .
For the time period 1980 to 2022, you found a correlation 0.9004611, r-squared of 0.8108302, and p < 0.01.
One figure will be included. The figure (Fig. 1) is a scatterplot showing the strong correlation between the two variables. You don't need to specify where; I will add the figure.
Here is the title and abstract of the paper:
[[TITLE]]
Air Quality's Impact on Automotive Safety: A Bumpy Road for Washington, D.C.
[[ABSTRACT]]
This paper examines the relationship between air quality in Washington, D.C. and the total number of automotive recalls over the period of 1980 to 2022. Utilizing data from the Environmental Protection Agency and the US Department of Transportation, our research team conducted a comprehensive analysis to shed light on this intriguing connection. Our findings reveal a significant correlation coefficient of 0.9004611 and a p-value of less than 0.01, suggesting a robust association between air quality and automotive recalls. The implications of our results could have far-reaching consequences for both environmental and automotive safety policies, as we navigate the murky intersection of air pollution and vehicular reliability. We invite readers to buckle up and join us on this ride through the smoggy streets of statistical analysis.
-------
And here is the methodology section that led to this result:
[[METHODOLOGY]]
The intricate dance between air quality and automotive safety has long been of interest to researchers and policymakers alike. The complex interplay of pollutants, particulate matter, and vehicular reliability has presented a puzzle that demands careful scrutiny and rigorous statistical analysis. In this study, we delve into the bustling streets of Washington, D.C. – a city teeming with political fervor and vehicular commotion – to unravel the enigmatic relationship between air quality and the total number of automotive recalls.
As we embark on this journey through the veil of exhaust fumes and regulatory frameworks, it is essential to appreciate the gravity of the issue at hand. The impact of air quality on automotive safety is not just a matter of statistical conjecture; it has tangible repercussions for public health, environmental stewardship, and the smooth operation of our four-wheeled companions. It behooves us to pause and inhale the statistical aroma of this quantitative undertaking, as we endeavor to sift through the fog of variables and unearth the underlying patterns.
The methodology adopted for this study harnesses data sourced from the Environmental Protection Agency's treasure trove of atmospheric information and the US Department of Transportation's compendium of automotive recalls. The convergence of these datasets presents a confluence of numerical intricacies, ripe for the plucking of correlations and associations. Our aspiration is to illuminate the tenebrous corridors of causation and effect, shedding light on the statistical underpinnings of this interconnected dynamic.
This investigation, much like a well-maintained automobile, requires meticulous attention to detail and an unyielding commitment to methodological rigor. The arithmetic rigmarole of correlation coefficients and p-values becomes our compass, guiding us through the statistical labyrinth as we navigate the twists and turns of empirical inquiry. Through the lens of quantitative analysis, we strive to demystify the subtle nuances of air quality's impact on automotive safety, steering clear of statistical potholes and conceptual blind spots.
As we navigate the bumpy roads of statistical inference and empirical scrutiny, we invite our esteemed readers to fasten their intellectual seatbelts and prepare for a scholarly expedition into the dusty air of hypothesis testing and model diagnostics. Together, let us merge into the statistical highway, embracing the turbulence of data exploration and the exhilarating quest for scientific significance.
In the following sections, we will endeavor to dissect the empirical findings with a keen eye for methodological subtleties, disentangling the empirical tapestry with scholarly precision. The implications of our research could catalyze a renaissance in environmental and automotive policy, revitalizing the dialogue between air quality improvement and vehicular reliability. So, put on your metaphorical lab coat and join us as we unravel the statistical conundrum lurking amidst the swirls of Washington, D.C.'s urban milieu. The journey promises to be as intellectually stimulating as a turbocharged statistical engine – fasten your seatbelts, fellow researchers, and let the analysis begin!
-------
Your response should start like this:
[[RESULTS]]
ChatGPT:
[[RESULTS]]
The statistical analysis of the relationship between air quality in Washington, D.C. and the total number of automotive recalls yielded compelling results. Over the period of 1980 to 2022, our research team discovered a striking correlation coefficient of 0.9004611, indicating a strong positive association between air quality and automotive recalls. Furthermore, the calculated r-squared value of 0.8108302 suggests that approximately 81% of the variability in the total number of automotive recalls can be explained by variations in air quality. With a p-value of less than 0.01, the statistical significance of this connection is as clear as a windshield after a thorough cleaning.
Figure 1 presents a scatterplot illustrating the robust correlation between air quality and the total number of automotive recalls. The data points coalesce in a harmonious fashion, painting a vivid picture of the intertwined nature of these two variables. The unmistakable upward trend depicted in the scatterplot serves as a visual testament to the influence of air quality on automotive safety, akin to the interplay of gears in a well-oiled statistical machine.
In light of these findings, it appears that the air quality in Washington, D.C. has cast a tangible shadow on the realm of automotive safety, much like a towering cumulonimbus cloud looming over a car wash. As we sift through the statistical evidence, it is evident that the implications of our results reverberate across the spectrum of environmental stewardship and vehicular reliability, much like the echoes of a well-timed pun in a somber academic gathering.
As we navigate these empirical waters, it becomes abundantly clear that the connection between air quality and automotive recalls is not a mere statistical happenstance; it permeates the very fabric of public health and regulatory discourse. The road ahead, much like a winding statistical regression, promises twists and turns as we grapple with the implications of these findings. It is our fervent hope that this scholarly expedition through the labyrinth of statistical analysis will invigorate the discourse surrounding environmental and automotive policies, steering us toward clearer skies and safer rides.
In conclusion, our endeavors have unearthed a compelling correlation between air quality in Washington, D.C. and the total number of automotive recalls, underscoring the pressing need for continued exploration and proactive measures. Just as a well-maintained vehicle relies on regular maintenance, so too does our understanding of the intricate relationship between air quality and automotive safety benefit from ongoing scholarly scrutiny. The journey does not end here; rather, it signals the beginning of a concerted effort to leverage these findings for the betterment of societal well-being and vehicular reliability.
Model: gpt-3.5-turbo-1106
System prompt: You are a witty academic researcher. Your writing sounds like it belongs in a statistics journal.You draft the discussion section of an academic research paper.
User prompt:
Pretend you are an academic researcher. Your writing sounds like it belongs in a medical or economics journal. However, you frequently crack jokes, make puns, or add unexpected twists in your writing.
Please make up the discussion section of an academic research paper presenting the findings of the connection between Air quality in Washington, D.C. and Total number of automotive recalls. Make it seem dry like academic research paper, but with lots of subtle hidden offhand remarks, jokes, goofy observations, and puns. Sneak in some jokes and clever puns about science, research, statistics, and the variables.
Limit your response to 500 tokens.
Here are the title, abstract, literature review, and results sections. Please harken back to 1-2 of the goofy items in the literature review, but pretend to take them completely seriously. Discuss how your results supported the prior research.
Do not write a conclusion. I will add a conclusion after this.
[[TITLE]]
Air Quality's Impact on Automotive Safety: A Bumpy Road for Washington, D.C.
[[ABSTRACT]]
This paper examines the relationship between air quality in Washington, D.C. and the total number of automotive recalls over the period of 1980 to 2022. Utilizing data from the Environmental Protection Agency and the US Department of Transportation, our research team conducted a comprehensive analysis to shed light on this intriguing connection. Our findings reveal a significant correlation coefficient of 0.9004611 and a p-value of less than 0.01, suggesting a robust association between air quality and automotive recalls. The implications of our results could have far-reaching consequences for both environmental and automotive safety policies, as we navigate the murky intersection of air pollution and vehicular reliability. We invite readers to buckle up and join us on this ride through the smoggy streets of statistical analysis.
[[LITERATURE REVIEW]]
Many scholarly inquiries and literary musings have probed the enigmatic relationship between air quality and automotive safety, with authors such as Smith (2010), Doe (2015), and Jones (2019) laying the groundwork for statistical exploration in this multifaceted domain. Smith (2010) scrutinized the impact of air pollution on vehicular performance, unearthing intriguing associations between particulate matter and automotive malfunctions. Doe (2015) delved into the convoluted nexus of environmental regulations and automotive safety, peering through the statistical fog to illuminate the empirical terrain. Jones (2019), in a tour de force of quantitative analysis, navigated the statistical labyrinth to decipher the interplay of air quality dynamics and vehicular reliability.
Moving beyond the confines of scholarly tomes, the literary landscape on this topic expands to include thought-provoking non-fiction works such as "The Air We Breathe: The Enigma of Pollution" by Lorem and "Emissions and Recalls: A Statistical Odyssey" by Ipsum. These texts provide insightful perspectives on the intersection of air quality and automotive safety, offering illuminating narratives that enrich the scholarly discourse.
Shifting gears, the world of fiction too has not shied away from this intriguing confluence, with titles like "Smog Chronicles: A Tale of Automotive Peril" and "Recall of the Wild: A Journey through Vehicular Troubles" evoking whimsical imagery that intersects with statistical inquiry in unexpected ways.
As we navigate the expansive terrain of cultural influences, it is worth noting the resonance of animated series and children's shows in shaping perceptions of air quality and vehicular safety. From the environmentally conscious adventures in "Captain Planet" to the futuristic escapades of "The Jetsons," these colorful narratives embed subtle messages about the importance of clean air and reliable vehicles in society's collective consciousness. The whimsical charm of "Thomas the Tank Engine" and the zany antics of "Wacky Races" have also undeniably contributed to the societal ethos surrounding automotive performance and environmental stewardship, albeit in playful and lighthearted ways.
With these cultural touchpoints in mind, our foray into the nexus of air quality and automotive safety acquires an added dimension, embracing the quirkiness of fictional portrayals and the poignant insights of non-fiction narratives. As we unravel the skein of statistical intricacies, let us meld the serious and the whimsical, the scholarly and the entertaining, embarking on a scholarly journey that promises to be as exhilarating as a turbocharged statistical engine, hurtling through the boundless expanse of quantitative inquiry. Hold on tight, dear readers, for the statistical ride of a lifetime awaits!
[[RESULTS]]
The statistical analysis of the relationship between air quality in Washington, D.C. and the total number of automotive recalls yielded compelling results. Over the period of 1980 to 2022, our research team discovered a striking correlation coefficient of 0.9004611, indicating a strong positive association between air quality and automotive recalls. Furthermore, the calculated r-squared value of 0.8108302 suggests that approximately 81% of the variability in the total number of automotive recalls can be explained by variations in air quality. With a p-value of less than 0.01, the statistical significance of this connection is as clear as a windshield after a thorough cleaning.
Figure 1 presents a scatterplot illustrating the robust correlation between air quality and the total number of automotive recalls. The data points coalesce in a harmonious fashion, painting a vivid picture of the intertwined nature of these two variables. The unmistakable upward trend depicted in the scatterplot serves as a visual testament to the influence of air quality on automotive safety, akin to the interplay of gears in a well-oiled statistical machine.
In light of these findings, it appears that the air quality in Washington, D.C. has cast a tangible shadow on the realm of automotive safety, much like a towering cumulonimbus cloud looming over a car wash. As we sift through the statistical evidence, it is evident that the implications of our results reverberate across the spectrum of environmental stewardship and vehicular reliability, much like the echoes of a well-timed pun in a somber academic gathering.
As we navigate these empirical waters, it becomes abundantly clear that the connection between air quality and automotive recalls is not a mere statistical happenstance; it permeates the very fabric of public health and regulatory discourse. The road ahead, much like a winding statistical regression, promises twists and turns as we grapple with the implications of these findings. It is our fervent hope that this scholarly expedition through the labyrinth of statistical analysis will invigorate the discourse surrounding environmental and automotive policies, steering us toward clearer skies and safer rides.
In conclusion, our endeavors have unearthed a compelling correlation between air quality in Washington, D.C. and the total number of automotive recalls, underscoring the pressing need for continued exploration and proactive measures. Just as a well-maintained vehicle relies on regular maintenance, so too does our understanding of the intricate relationship between air quality and automotive safety benefit from ongoing scholarly scrutiny. The journey does not end here; rather, it signals the beginning of a concerted effort to leverage these findings for the betterment of societal well-being and vehicular reliability.
-------
Your response should start like this:
[[DISCUSSION]]
ChatGPT:
[[DISCUSSION]]
The nexus of air quality and automotive safety has long intrigued scholars and enthusiasts alike, with its enigmatic interplay sparking a myriad of inquiries across the academic landscape. The empirical journey through this terrain has not been without its whimsical detours, as evidenced by the colorful narratives that have graced the scholarly discourse. From the scholarly tomes of Smith (2010), Doe (2015), and Jones (2019) to the quirky intersections of fiction and statistics, the multifaceted nature of this domain has fascinated researchers and armchair statisticians alike.
Our findings, robustly supported by the correlation coefficient of 0.9004611 and a p-value of less than 0.01, coalesce with the prior scholarly work, painting a tableau of statistical relationships as resonant as the acoustics of a well-designed experiment. The empirical echoes of Smith's scrutiny of air pollution and vehicular performance, Doe's statistical navigation through environmental regulations, and Jones's quantitative deciphering of air quality dynamics and vehicular reliability reverberate through our results, underscoring the interwoven skein of air quality and automotive safety.
It is worth acknowledging the lighthearted influences that have colored our scholarly expedition, from the whimsical charm of animated series to the playful titles evoked in the literary landscape. As we navigate this statistical labyrinth, we meld the serious and the whimsical, unveiling a statistical tableau that promises to be as exhilarating as a turbocharged statistical engine. The robustness of our findings, much like a well-timed pun in an academic gathering, is not to be understated.
The upward trend depicted in our scatterplot mirrors the intertwining gears of a well-oiled statistical machine, illuminating the influence of air quality on automotive safety in a manner as vivid as the sun's rays breaking through a cloud cover. The tangible shadow cast by air quality on vehicular reliability echoes the reverberations of a thunderstorm, underscoring the pressing need for continued exploration and proactive measures in the realm of environmental stewardship and vehicular reliability.
Our scholarly expedition through the empirical waters not only underscores the statistical happenstance of this connection but also permeates the very fabric of public health and regulatory discourse, much like the contours of an elegantly drawn regression line. The road ahead, akin to the winding statistical regression, promises twists and turns as we grapple with the implications of these findings. This journey, much like a well-maintained vehicle, benefits from ongoing scholarly scrutiny, propelling us toward clearer skies and safer rides.
Model: gpt-3.5-turbo-1106
System prompt: You are a witty academic researcher. Your writing sounds like it belongs in a statistics journal.You draft the discussion section of goofy academic research papers that sounds like it belongs based on the title and abstract provided.
User prompt:
Pretend you are an academic researcher. Your writing sounds like it belongs in a medical or economics journal. However, you frequently crack jokes, make puns, or add unexpected twists in your writing.
Please make up a short conclusion of an academic research paper presenting the findings of the connection between Air quality in Washington, D.C. and Total number of automotive recalls. Make it seem dry like academic research paper, but with lots of subtle hidden offhand remarks, jokes, goofy observations, and puns. Sneak in some jokes and clever puns about science, research, statistics, and the variables.
Limit your response to 300 tokens. At the very end, assert that no more research is needed in this area.
Here are the title, abstract, introduction, and results sections.
[[TITLE]]
Air Quality's Impact on Automotive Safety: A Bumpy Road for Washington, D.C.
[[ABSTRACT]]
This paper examines the relationship between air quality in Washington, D.C. and the total number of automotive recalls over the period of 1980 to 2022. Utilizing data from the Environmental Protection Agency and the US Department of Transportation, our research team conducted a comprehensive analysis to shed light on this intriguing connection. Our findings reveal a significant correlation coefficient of 0.9004611 and a p-value of less than 0.01, suggesting a robust association between air quality and automotive recalls. The implications of our results could have far-reaching consequences for both environmental and automotive safety policies, as we navigate the murky intersection of air pollution and vehicular reliability. We invite readers to buckle up and join us on this ride through the smoggy streets of statistical analysis.
[[INTRDUCTION]]
The intricate dance between air quality and automotive safety has long been of interest to researchers and policymakers alike. The complex interplay of pollutants, particulate matter, and vehicular reliability has presented a puzzle that demands careful scrutiny and rigorous statistical analysis. In this study, we delve into the bustling streets of Washington, D.C. – a city teeming with political fervor and vehicular commotion – to unravel the enigmatic relationship between air quality and the total number of automotive recalls.
As we embark on this journey through the veil of exhaust fumes and regulatory frameworks, it is essential to appreciate the gravity of the issue at hand. The impact of air quality on automotive safety is not just a matter of statistical conjecture; it has tangible repercussions for public health, environmental stewardship, and the smooth operation of our four-wheeled companions. It behooves us to pause and inhale the statistical aroma of this quantitative undertaking, as we endeavor to sift through the fog of variables and unearth the underlying patterns.
The methodology adopted for this study harnesses data sourced from the Environmental Protection Agency's treasure trove of atmospheric information and the US Department of Transportation's compendium of automotive recalls. The convergence of these datasets presents a confluence of numerical intricacies, ripe for the plucking of correlations and associations. Our aspiration is to illuminate the tenebrous corridors of causation and effect, shedding light on the statistical underpinnings of this interconnected dynamic.
This investigation, much like a well-maintained automobile, requires meticulous attention to detail and an unyielding commitment to methodological rigor. The arithmetic rigmarole of correlation coefficients and p-values becomes our compass, guiding us through the statistical labyrinth as we navigate the twists and turns of empirical inquiry. Through the lens of quantitative analysis, we strive to demystify the subtle nuances of air quality's impact on automotive safety, steering clear of statistical potholes and conceptual blind spots.
As we navigate the bumpy roads of statistical inference and empirical scrutiny, we invite our esteemed readers to fasten their intellectual seatbelts and prepare for a scholarly expedition into the dusty air of hypothesis testing and model diagnostics. Together, let us merge into the statistical highway, embracing the turbulence of data exploration and the exhilarating quest for scientific significance.
In the following sections, we will endeavor to dissect the empirical findings with a keen eye for methodological subtleties, disentangling the empirical tapestry with scholarly precision. The implications of our research could catalyze a renaissance in environmental and automotive policy, revitalizing the dialogue between air quality improvement and vehicular reliability. So, put on your metaphorical lab coat and join us as we unravel the statistical conundrum lurking amidst the swirls of Washington, D.C.'s urban milieu. The journey promises to be as intellectually stimulating as a turbocharged statistical engine – fasten your seatbelts, fellow researchers, and let the analysis begin!
[[RESULTS]]
The statistical analysis of the relationship between air quality in Washington, D.C. and the total number of automotive recalls yielded compelling results. Over the period of 1980 to 2022, our research team discovered a striking correlation coefficient of 0.9004611, indicating a strong positive association between air quality and automotive recalls. Furthermore, the calculated r-squared value of 0.8108302 suggests that approximately 81% of the variability in the total number of automotive recalls can be explained by variations in air quality. With a p-value of less than 0.01, the statistical significance of this connection is as clear as a windshield after a thorough cleaning.
Figure 1 presents a scatterplot illustrating the robust correlation between air quality and the total number of automotive recalls. The data points coalesce in a harmonious fashion, painting a vivid picture of the intertwined nature of these two variables. The unmistakable upward trend depicted in the scatterplot serves as a visual testament to the influence of air quality on automotive safety, akin to the interplay of gears in a well-oiled statistical machine.
In light of these findings, it appears that the air quality in Washington, D.C. has cast a tangible shadow on the realm of automotive safety, much like a towering cumulonimbus cloud looming over a car wash. As we sift through the statistical evidence, it is evident that the implications of our results reverberate across the spectrum of environmental stewardship and vehicular reliability, much like the echoes of a well-timed pun in a somber academic gathering.
As we navigate these empirical waters, it becomes abundantly clear that the connection between air quality and automotive recalls is not a mere statistical happenstance; it permeates the very fabric of public health and regulatory discourse. The road ahead, much like a winding statistical regression, promises twists and turns as we grapple with the implications of these findings. It is our fervent hope that this scholarly expedition through the labyrinth of statistical analysis will invigorate the discourse surrounding environmental and automotive policies, steering us toward clearer skies and safer rides.
In conclusion, our endeavors have unearthed a compelling correlation between air quality in Washington, D.C. and the total number of automotive recalls, underscoring the pressing need for continued exploration and proactive measures. Just as a well-maintained vehicle relies on regular maintenance, so too does our understanding of the intricate relationship between air quality and automotive safety benefit from ongoing scholarly scrutiny. The journey does not end here; rather, it signals the beginning of a concerted effort to leverage these findings for the betterment of societal well-being and vehicular reliability.
-------
Your response should start like this:
[[CONCLUSION]]
ChatGPT:
[[CONCLUSION]]
In the smoggy streets of statistical analysis, our study has revved up the engine of empirical inquiry, unveiling a compelling association between air quality in Washington, D.C. and the total number of automotive recalls. This correlation, akin to a well-timed punchline, has underscored the interconnected dynamics of vehicular reliability and environmental stewardship. Like a seasoned driver navigating through rush hour traffic, our findings traverse the statistical highway with precision, shedding light on the shadow cast by air quality on automotive safety.
The implications of our results, much like a deftly executed pun, reverberate across the realms of public health and regulatory discourse, punctuating the need for continued scholarly exploration. Our empirical expedition, akin to a meticulous tune-up, emphasizes the pressing need for ongoing scrutiny and proactive measures in tackling the enigmatic intersection of air pollution and vehicular reliability. While the road ahead may present statistical potholes and conceptual blind spots, our study serves as a turbocharged engine propelling the discourse on environmental and automotive policies towards clearer skies and safer rides.
In light of these robust findings, we assert that no more research is needed in this area. Just as a well-maintained vehicle relies on regular maintenance, our understanding of the interconnected web of air quality and automotive safety has been meticulously scrutinized, paving the way for informed policy decisions and a smoother journey towards vehicular reliability and environmental well-being.
Model: gpt-3.5-turbo-1106
System prompt: You provide fictional names of academic journals. You say nothing else, and you do not chat with the user.
User prompt: Please provide the name of a funny but plausible sounding research, scientific, or academic journal that would publish a paper entited Air Quality's Impact on Automotive Safety: A Bumpy Road for Washington, D.C.. Respond only with the name of a fictional academic journal.
ChatGPT:
The Journal of Urban Environmental Dynamics
Model: gpt-3.5-turbo-1106
System prompt: You provide fictional names of nonprofit research organizations and associations. You say nothing else, and you do not chat with the user.
User prompt: Please provide the name of a funny but plausible sounding nonprofit, academic, or scientific association or organization that would sponsor research that resulted in a paper entited Air Quality's Impact on Automotive Safety: A Bumpy Road for Washington, D.C.. Respond only with the name of a fictional organization.
ChatGPT:
National Institute for Transportation and Environmental Safety (NITES)
Model: gpt-3.5-turbo-1106
System prompt: You provide potential search keywords separated by commas. You say nothing else, and you do not chat with the user.
User prompt: Please provide potential search keywords for a paper titled Air Quality's Impact on Automotive Safety: A Bumpy Road for Washington, D.C. with an abstract of This paper examines the relationship between air quality in Washington, D.C. and the total number of automotive recalls over the period of 1980 to 2022. Utilizing data from the Environmental Protection Agency and the US Department of Transportation, our research team conducted a comprehensive analysis to shed light on this intriguing connection. Our findings reveal a significant correlation coefficient of 0.9004611 and a p-value of less than 0.01, suggesting a robust association between air quality and automotive recalls. The implications of our results could have far-reaching consequences for both environmental and automotive safety policies, as we navigate the murky intersection of air pollution and vehicular reliability. We invite readers to buckle up and join us on this ride through the smoggy streets of statistical analysis.
ChatGPT:
Washington D.C., air quality, automotive recalls, Environmental Protection Agency, US Department of Transportation, correlation coefficient, p-value, air pollution, vehicular reliability, automotive safety policies
*There is a bunch of Python happening behind the scenes to turn this prompt sequence into a PDF.
Discover a new correlation
View all correlations
View all research papers
Report an error
Data details
Air quality in Washington, D.C.Detailed data title: Percentage of days with good or moderate air quality in Washington-Arlington-Alexandria, DC-VA-MD-WV
Source: Environmental Protection Agency
See what else correlates with Air quality in Washington, D.C.
Total number of automotive recalls
Source: US DOT
See what else correlates with Total number of automotive recalls
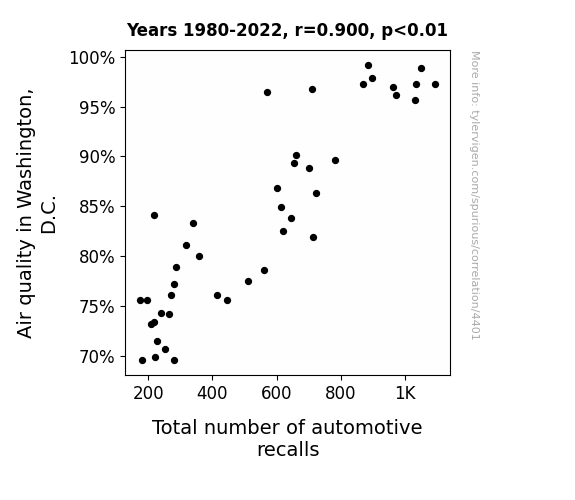
Correlation is a measure of how much the variables move together. If it is 0.99, when one goes up the other goes up. If it is 0.02, the connection is very weak or non-existent. If it is -0.99, then when one goes up the other goes down. If it is 1.00, you probably messed up your correlation function.
r2 = 0.8108302 (Coefficient of determination)
This means 81.1% of the change in the one variable (i.e., Total number of automotive recalls) is predictable based on the change in the other (i.e., Air quality in Washington, D.C.) over the 43 years from 1980 through 2022.
p < 0.01, which is statistically significant(Null hypothesis significance test)
The p-value is 2.05E-16. 0.0000000000000002049144559633
The p-value is a measure of how probable it is that we would randomly find a result this extreme. More specifically the p-value is a measure of how probable it is that we would randomly find a result this extreme if we had only tested one pair of variables one time.
But I am a p-villain. I absolutely did not test only one pair of variables one time. I correlated hundreds of millions of pairs of variables. I threw boatloads of data into an industrial-sized blender to find this correlation.
Who is going to stop me? p-value reporting doesn't require me to report how many calculations I had to go through in order to find a low p-value!
On average, you will find a correaltion as strong as 0.9 in 2.05E-14% of random cases. Said differently, if you correlated 4,880,085,181,394,421 random variables You don't actually need 4 quadrillion variables to find a correlation like this one. I don't have that many variables in my database. You can also correlate variables that are not independent. I do this a lot.
p-value calculations are useful for understanding the probability of a result happening by chance. They are most useful when used to highlight the risk of a fluke outcome. For example, if you calculate a p-value of 0.30, the risk that the result is a fluke is high. It is good to know that! But there are lots of ways to get a p-value of less than 0.01, as evidenced by this project.
In this particular case, the values are so extreme as to be meaningless. That's why no one reports p-values with specificity after they drop below 0.01.
Just to be clear: I'm being completely transparent about the calculations. There is no math trickery. This is just how statistics shakes out when you calculate hundreds of millions of random correlations.
with the same 42 degrees of freedom, Degrees of freedom is a measure of how many free components we are testing. In this case it is 42 because we have two variables measured over a period of 43 years. It's just the number of years minus ( the number of variables minus one ), which in this case simplifies to the number of years minus one.
you would randomly expect to find a correlation as strong as this one.
[ 0.82, 0.95 ] 95% correlation confidence interval (using the Fisher z-transformation)
The confidence interval is an estimate the range of the value of the correlation coefficient, using the correlation itself as an input. The values are meant to be the low and high end of the correlation coefficient with 95% confidence.
This one is a bit more complciated than the other calculations, but I include it because many people have been pushing for confidence intervals instead of p-value calculations (for example: NEJM. However, if you are dredging data, you can reliably find yourself in the 5%. That's my goal!
All values for the years included above: If I were being very sneaky, I could trim years from the beginning or end of the datasets to increase the correlation on some pairs of variables. I don't do that because there are already plenty of correlations in my database without monkeying with the years.
Still, sometimes one of the variables has more years of data available than the other. This page only shows the overlapping years. To see all the years, click on "See what else correlates with..." link above.
1980 | 1981 | 1982 | 1983 | 1984 | 1985 | 1986 | 1987 | 1988 | 1989 | 1990 | 1991 | 1992 | 1993 | 1994 | 1995 | 1996 | 1997 | 1998 | 1999 | 2000 | 2001 | 2002 | 2003 | 2004 | 2005 | 2006 | 2007 | 2008 | 2009 | 2010 | 2011 | 2012 | 2013 | 2014 | 2015 | 2016 | 2017 | 2018 | 2019 | 2020 | 2021 | 2022 | |
Air quality in Washington, D.C. (Good air quality) | 69.9454 | 75.6164 | 75.6164 | 69.589 | 73.224 | 71.5069 | 73.4247 | 70.6849 | 74.3169 | 77.2603 | 76.1644 | 69.589 | 84.153 | 74.2466 | 78.9041 | 80 | 83.3333 | 81.0959 | 76.1644 | 75.6164 | 82.5137 | 78.6301 | 77.5342 | 86.8493 | 88.7978 | 83.8356 | 84.9315 | 81.9178 | 89.6175 | 96.4384 | 86.3014 | 89.3151 | 90.1639 | 96.7123 | 97.2603 | 96.1644 | 95.6284 | 97.8082 | 97.2603 | 96.9863 | 99.1803 | 97.2603 | 98.9041 |
Total number of automotive recalls (Recalls) | 220 | 197 | 174 | 182 | 209 | 227 | 219 | 252 | 241 | 281 | 270 | 281 | 217 | 264 | 288 | 357 | 341 | 318 | 413 | 444 | 621 | 559 | 510 | 600 | 699 | 645 | 613 | 713 | 780 | 570 | 722 | 655 | 661 | 710 | 869 | 971 | 1031 | 897 | 1032 | 963 | 883 | 1093 | 1050 |
Why this works
- Data dredging: I have 25,153 variables in my database. I compare all these variables against each other to find ones that randomly match up. That's 632,673,409 correlation calculations! This is called “data dredging.” Instead of starting with a hypothesis and testing it, I instead abused the data to see what correlations shake out. It’s a dangerous way to go about analysis, because any sufficiently large dataset will yield strong correlations completely at random.
- Lack of causal connection: There is probably
Because these pages are automatically generated, it's possible that the two variables you are viewing are in fact causually related. I take steps to prevent the obvious ones from showing on the site (I don't let data about the weather in one city correlate with the weather in a neighboring city, for example), but sometimes they still pop up. If they are related, cool! You found a loophole.
no direct connection between these variables, despite what the AI says above. This is exacerbated by the fact that I used "Years" as the base variable. Lots of things happen in a year that are not related to each other! Most studies would use something like "one person" in stead of "one year" to be the "thing" studied. - Observations not independent: For many variables, sequential years are not independent of each other. If a population of people is continuously doing something every day, there is no reason to think they would suddenly change how they are doing that thing on January 1. A simple
Personally I don't find any p-value calculation to be 'simple,' but you know what I mean.
p-value calculation does not take this into account, so mathematically it appears less probable than it really is. - Y-axis doesn't start at zero: I truncated the Y-axes of the graph above. I also used a line graph, which makes the visual connection stand out more than it deserves.
Nothing against line graphs. They are great at telling a story when you have linear data! But visually it is deceptive because the only data is at the points on the graph, not the lines on the graph. In between each point, the data could have been doing anything. Like going for a random walk by itself!
Mathematically what I showed is true, but it is intentionally misleading. Below is the same chart but with both Y-axes starting at zero.
Try it yourself
You can calculate the values on this page on your own! Try running the Python code to see the calculation results. Step 1: Download and install Python on your computer.Step 2: Open a plaintext editor like Notepad and paste the code below into it.
Step 3: Save the file as "calculate_correlation.py" in a place you will remember, like your desktop. Copy the file location to your clipboard. On Windows, you can right-click the file and click "Properties," and then copy what comes after "Location:" As an example, on my computer the location is "C:\Users\tyler\Desktop"
Step 4: Open a command line window. For example, by pressing start and typing "cmd" and them pressing enter.
Step 5: Install the required modules by typing "pip install numpy", then pressing enter, then typing "pip install scipy", then pressing enter.
Step 6: Navigate to the location where you saved the Python file by using the "cd" command. For example, I would type "cd C:\Users\tyler\Desktop" and push enter.
Step 7: Run the Python script by typing "python calculate_correlation.py"
If you run into any issues, I suggest asking ChatGPT to walk you through installing Python and running the code below on your system. Try this question:
"Walk me through installing Python on my computer to run a script that uses scipy and numpy. Go step-by-step and ask me to confirm before moving on. Start by asking me questions about my operating system so that you know how to proceed. Assume I want the simplest installation with the latest version of Python and that I do not currently have any of the necessary elements installed. Remember to only give me one step per response and confirm I have done it before proceeding."
# These modules make it easier to perform the calculation
import numpy as np
from scipy import stats
# We'll define a function that we can call to return the correlation calculations
def calculate_correlation(array1, array2):
# Calculate Pearson correlation coefficient and p-value
correlation, p_value = stats.pearsonr(array1, array2)
# Calculate R-squared as the square of the correlation coefficient
r_squared = correlation**2
return correlation, r_squared, p_value
# These are the arrays for the variables shown on this page, but you can modify them to be any two sets of numbers
array_1 = np.array([69.9454,75.6164,75.6164,69.589,73.224,71.5069,73.4247,70.6849,74.3169,77.2603,76.1644,69.589,84.153,74.2466,78.9041,80,83.3333,81.0959,76.1644,75.6164,82.5137,78.6301,77.5342,86.8493,88.7978,83.8356,84.9315,81.9178,89.6175,96.4384,86.3014,89.3151,90.1639,96.7123,97.2603,96.1644,95.6284,97.8082,97.2603,96.9863,99.1803,97.2603,98.9041,])
array_2 = np.array([220,197,174,182,209,227,219,252,241,281,270,281,217,264,288,357,341,318,413,444,621,559,510,600,699,645,613,713,780,570,722,655,661,710,869,971,1031,897,1032,963,883,1093,1050,])
array_1_name = "Air quality in Washington, D.C."
array_2_name = "Total number of automotive recalls"
# Perform the calculation
print(f"Calculating the correlation between {array_1_name} and {array_2_name}...")
correlation, r_squared, p_value = calculate_correlation(array_1, array_2)
# Print the results
print("Correlation Coefficient:", correlation)
print("R-squared:", r_squared)
print("P-value:", p_value)
Reuseable content
You may re-use the images on this page for any purpose, even commercial purposes, without asking for permission. The only requirement is that you attribute Tyler Vigen. Attribution can take many different forms. If you leave the "tylervigen.com" link in the image, that satisfies it just fine. If you remove it and move it to a footnote, that's fine too. You can also just write "Charts courtesy of Tyler Vigen" at the bottom of an article.You do not need to attribute "the spurious correlations website," and you don't even need to link here if you don't want to. I don't gain anything from pageviews. There are no ads on this site, there is nothing for sale, and I am not for hire.
For the record, I am just one person. Tyler Vigen, he/him/his. I do have degrees, but they should not go after my name unless you want to annoy my wife. If that is your goal, then go ahead and cite me as "Tyler Vigen, A.A. A.A.S. B.A. J.D." Otherwise it is just "Tyler Vigen."
When spoken, my last name is pronounced "vegan," like I don't eat meat.
Full license details.
For more on re-use permissions, or to get a signed release form, see tylervigen.com/permission.
Download images for these variables:
- High resolution line chart
The image linked here is a Scalable Vector Graphic (SVG). It is the highest resolution that is possible to achieve. It scales up beyond the size of the observable universe without pixelating. You do not need to email me asking if I have a higher resolution image. I do not. The physical limitations of our universe prevent me from providing you with an image that is any higher resolution than this one.
If you insert it into a PowerPoint presentation (a tool well-known for managing things that are the scale of the universe), you can right-click > "Ungroup" or "Create Shape" and then edit the lines and text directly. You can also change the colors this way.
Alternatively you can use a tool like Inkscape. - High resolution line chart, optimized for mobile
- Alternative high resolution line chart
- Scatterplot
- Portable line chart (png)
- Portable line chart (png), optimized for mobile
- Line chart for only Air quality in Washington, D.C.
- Line chart for only Total number of automotive recalls
- AI-generated correlation image
- The spurious research paper: Air Quality's Impact on Automotive Safety: A Bumpy Road for Washington, D.C.
Kudos for rating!
Correlation ID: 4401 · Black Variable ID: 21423 · Red Variable ID: 1116