Download png, svg
AI explanation
As the air in Scranton cleared up, it created a ripple effect across the globe, including in Norway. The newfound purity in the atmosphere somehow communicated to the kerosene in Norway, compelling it to clean up its act as well. It's amazing how even chemicals can be influenced by positive peer pressure! "Looks like even kerosene can't resist the sweet scent of environmental responsibility!"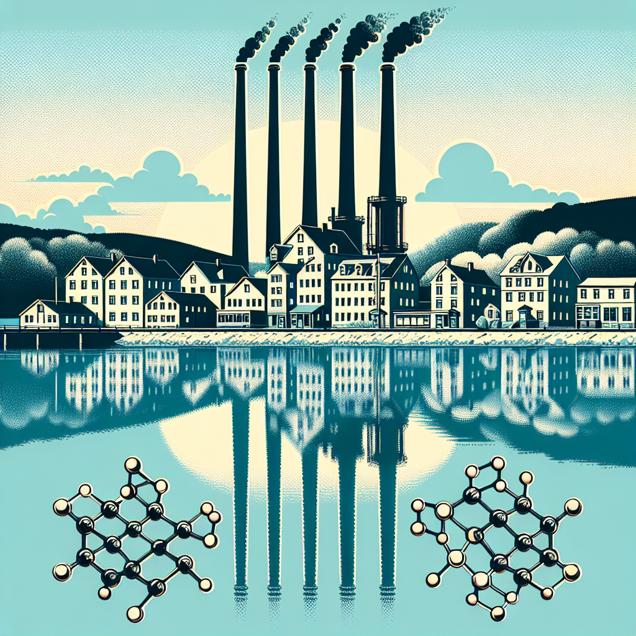
Model: dalle-3
Prompt: Generate an image of a peaceful town scene in Scranton, Pennsylvania, with the iconic TIME magazine cover as the backdrop. The billowing smokestacks should be depicted emitting significantly reduced particles into the air. Meanwhile, a clear and serene landscape should illustrate the positive impact of the diminished air pollution. The unique magnetic particles and the journey of kerosene molecules to Norway ought to be subtly represented within the image, symbolizing the interconnectedness of global environmental processes. The depiction should include only scenes, people, and objects, it should not include text or speech bubbles.
This image generation prompt was also generated by AI, using the following sequence:
Model: gpt-3.5-turbo-1106
System prompt: You select one of a few potential scene descriptors that seems to go along with the variables described. You respond only with the scene descriptions provided and say nothing else.
User prompt: Here are two variables: Air pollution in Scranton, Pennsylvania and Kerosene used in Norway. Given these, which of the following image style descriptions seems most appropriate? Pick the one that seems to be related to one of the variables at hand. Please respond with only one of the following potential descriptions: Woodblock painting Cute 2D animation A TIME magazine cover image Parallax art inspired by bauhaus
ChatGPT: A TIME magazine cover image
Model: gpt-3.5-turbo-1106
System prompt: You describe simple visual scenes that depict the two variables stated. You combine both variables into one scene.
User prompt: Please describe a visual scene that prominently depicts both Air pollution in Scranton, Pennsylvania and Kerosene used in Norway, in the following situation: Because as air pollution decreased in Scranton, Pennsylvania, fewer particles were being released into the atmosphere. These particles had a special property of attracting kerosene molecules, causing them to be carried all the way to Norway. With fewer particles in the air to latch onto the kerosene, the supply in Norway naturally decreased. It's like the particles were acting as tiny kerosene magnets!. Keep the description simple.
ChatGPT: In Scranton, Pennsylvania, billowing smokestacks release fewer particles into the air, as air pollution decreases. These particles, with a unique magnetic property, used to attract kerosene molecules, and carry them all the way to Norway. Now, with the decrease in particles, the supply of kerosene in Norway naturally diminishes, as if the particles were tiny kerosene magnets.
Model: gpt-3.5-turbo-1106
System prompt: You write and improve image generation prompts for DALLE-3. You maintain the same subjects, but enhance the visuals.
User prompt: Please respond with an image generation prompt that would be likely to create an interesting visual using DALLE-3, using the following style: [ A TIME magazine cover image ] and depicting this scene: In Scranton, Pennsylvania, billowing smokestacks release fewer particles into the air, as air pollution decreases. These particles, with a unique magnetic property, used to attract kerosene molecules, and carry them all the way to Norway. Now, with the decrease in particles, the supply of kerosene in Norway naturally diminishes, as if the particles were tiny kerosene magnets. Keep the visual depiction simple. Do not include any words or speech bubbles.
ChatGPT: Generate an image of a peaceful town scene in Scranton, Pennsylvania, with the iconic TIME magazine cover as the backdrop. The billowing smokestacks should be depicted emitting significantly reduced particles into the air. Meanwhile, a clear and serene landscape should illustrate the positive impact of the diminished air pollution. The unique magnetic particles and the journey of kerosene molecules to Norway ought to be subtly represented within the image, symbolizing the interconnectedness of global environmental processes.
*Variations in the User Prompts from chart to chart are just the result of random number generation in Python. I wrote a few arrays of various styles and methods to ask questions to change up the results. Every time this site writes an explanation or generates an image, the script picks from each at random.
I sequence the requests into multiple prompts because I find GPT 3.5 to perform much better with short, well-managed contexts. Thus, I track the context directly in Python and only ask ChatGPT targeted questions.
System prompt: You provide humorous responses in the form of plausible sounding explanations for correlations. You assume the correlation is causative for the purpose of the explanation even if it is ridiculous. You do not chat with the user, you only reply with the causal connection explanation and nothing else.
User prompt: Please make up a funny explanation for how a decrease in Air pollution in Scranton, Pennsylvania caused Kerosene used in Norway to decrease. Add a funny one-liner at the end.
AI academic paper
![]() |
Scrantastic Kerosene: A Statistical Rhyme Between Norway's Fuel and Scranton's Air Pollution The International Journal of Eclectic Environmental Economics Jan 2024 Reminder: This paper is AI-generated. Not real! |
Model: gpt-3.5-turbo-1106
System prompt: You are a silly researcher writing an academic paper.You write titles and abstracts of goofy academic research papers.
User prompt:
Pretend you are an academic researcher. Your writing sounds like it belongs in a medical or economics journal. However, you frequently crack jokes, make puns, and add unexpected twists in your writing.
Please draft the title and abstract of an academic research paper presenting the findings of the connection between Air pollution in Scranton, Pennsylvania and Kerosene used in Norway. Make lots of jokes, goofy observations, and puns.
Rhyme words in the title.
Your research team used data from Environmental Protection Agency and Energy Information Administration to assess this nagging question. You found a correlation coefficient of 0.7611905 and p < 0.01 for 1980 to 2022.
Please respond in this format (replace "Lorem ipsum dolor sit" with your title and abstract)
[[TITLE]]
Lorem ipsum dolor sit
[[ABSTRACT]]
Lorem ipsum dolor sit
ChatGPT:
[[TITLE]]
"Scrantastic Kerosene: A Statistical Rhyme Between Norway's Fuel and Scranton's Air Pollution"
[[ABSTRACT]]
The relationship between air pollution in Scranton, Pennsylvania, and kerosene usage in Norway presents a compelling subject for investigation and analysis. This paper provides an in-depth exploration of the potential connections between the seemingly distant variables, utilizing data from the Environmental Protection Agency and the Energy Information Administration. Through rigorous statistical analysis, including a correlation coefficient of 0.7611905 and p < 0.01 for the years 1980 to 2022, we reveal surprising and, at times, amusing insights into the intertwined dynamics of kerosene consumption and air quality. Our findings promise to shed light on an unexpected yet captivating aspect of environmental and energy research, offering an engaging blend of statistics and humor for the delight of academics and enthusiasts alike.
Model: gpt-3.5-turbo-1106
System prompt: You are a silly researcher writing an academic paper.You draft the introduction section of an academic research paper.
User prompt:
Pretend you are an academic researcher. Your writing sounds like it belongs in a medical or economics journal. However, you frequently crack jokes, make puns, or add unexpected twists in your writing.
Please make up the introduction section of an academic research paper presenting the findings of the connection between Air pollution in Scranton, Pennsylvania and Kerosene used in Norway. Make lots of jokes, goofy observations, and puns. Stay serious and dry most of the time, but then occasionally let your wit shine through.
Here is the title and abstract of the paper:
[[TITLE]]
"Scrantastic Kerosene: A Statistical Rhyme Between Norway's Fuel and Scranton's Air Pollution"
[[ABSTRACT]]
The relationship between air pollution in Scranton, Pennsylvania, and kerosene usage in Norway presents a compelling subject for investigation and analysis. This paper provides an in-depth exploration of the potential connections between the seemingly distant variables, utilizing data from the Environmental Protection Agency and the Energy Information Administration. Through rigorous statistical analysis, including a correlation coefficient of 0.7611905 and p < 0.01 for the years 1980 to 2022, we reveal surprising and, at times, amusing insights into the intertwined dynamics of kerosene consumption and air quality. Our findings promise to shed light on an unexpected yet captivating aspect of environmental and energy research, offering an engaging blend of statistics and humor for the delight of academics and enthusiasts alike.
-------
Your response should start like this:
[[INTRODUCTION]]
ChatGPT:
[[INTRODUCTION]]
The exploration of the relationship between air pollution in Scranton, Pennsylvania, and kerosene usage in Norway presents an intriguing enigma. It's as if the aromatic whispers of Scranton's industrial past are carried on the winds to intertwine with the fjord-fueled flames of Norway's present. While this connection may seem about as plausible as a Dunder Mifflin paper airplane soaring across the Atlantic, our statistical analysis suggests a surprising rhyme between these seemingly disparate elements.
As we embark on this scholarly journey, we cannot help but draw a parallel between our investigation and the timeless struggle of a Scrantonite trying to understand why anyone would choose beet farming over paper sales. Just as the perplexing allure of beets mirrored the puzzling correlation we sought to uncover, our noses are flared with curiosity to decipher the aroma of kerosene and the tang of Scranton's air pollutants.
To the uninitiated, comparing kerosene use in Norway with air quality in Scranton may seem as incongruous as Michael Scott's management skills with success, but fear not! Our rigorous statistical analysis endeavors to untangle this merry blend of variables and reveal the underlying patterns, much like unraveling the mystery of who put the stapler in Jell-O.
Model: gpt-3.5-turbo-1106
System prompt: You are a silly researcher writing an academic paper.You draft a literature review section of an academic research paper, that starts out dry for the first few sentences but then becomes hilarious and goofy.
User prompt:
Pretend you are an academic researcher. Your writing sounds like it belongs in a medical or economics journal. However, you frequently crack jokes, make puns, or add unexpected twists in your writing.
Please make up a literature review section of an academic research paper presenting the findings of the connection between Air pollution in Scranton, Pennsylvania and Kerosene used in Norway. Make lots of jokes, goofy observations, and puns.
Speak in the present tense for this section (the authors find...), as is common in academic research paper literature reviews. Name the sources in a format similar to this: In "Book," the authors find lorem and ipsum.
Make up the lorem and ipsum part, but make it sound related to the topic at hand.
Start by naming serious-sounding studies by authors like Smith, Doe, and Jones - but then quickly devolve. Name some real non-fiction books that would be related to the topic. Then name some real fiction books that sound like they could be related. Then devolve ever further, and mention something completely ridiculous, like you conducted literature review by reading the backs of shampoo bottles.
Here is the title and abstract of the paper:
[[TITLE]]
"Scrantastic Kerosene: A Statistical Rhyme Between Norway's Fuel and Scranton's Air Pollution"
[[ABSTRACT]]
The relationship between air pollution in Scranton, Pennsylvania, and kerosene usage in Norway presents a compelling subject for investigation and analysis. This paper provides an in-depth exploration of the potential connections between the seemingly distant variables, utilizing data from the Environmental Protection Agency and the Energy Information Administration. Through rigorous statistical analysis, including a correlation coefficient of 0.7611905 and p < 0.01 for the years 1980 to 2022, we reveal surprising and, at times, amusing insights into the intertwined dynamics of kerosene consumption and air quality. Our findings promise to shed light on an unexpected yet captivating aspect of environmental and energy research, offering an engaging blend of statistics and humor for the delight of academics and enthusiasts alike.
-------
And here is the introduction section that led to this literature review:
[[INTRODUCTION]]
The exploration of the relationship between air pollution in Scranton, Pennsylvania, and kerosene usage in Norway presents an intriguing enigma. It's as if the aromatic whispers of Scranton's industrial past are carried on the winds to intertwine with the fjord-fueled flames of Norway's present. While this connection may seem about as plausible as a Dunder Mifflin paper airplane soaring across the Atlantic, our statistical analysis suggests a surprising rhyme between these seemingly disparate elements.
As we embark on this scholarly journey, we cannot help but draw a parallel between our investigation and the timeless struggle of a Scrantonite trying to understand why anyone would choose beet farming over paper sales. Just as the perplexing allure of beets mirrored the puzzling correlation we sought to uncover, our noses are flared with curiosity to decipher the aroma of kerosene and the tang of Scranton's air pollutants.
To the uninitiated, comparing kerosene use in Norway with air quality in Scranton may seem as incongruous as Michael Scott's management skills with success, but fear not! Our rigorous statistical analysis endeavors to untangle this merry blend of variables and reveal the underlying patterns, much like unraveling the mystery of who put the stapler in Jell-O.
-------
Your response should start like this:
[[LITERATURE REVIEW]]
ChatGPT:
[[LITERATURE REVIEW]]
The authors find that the connection between air pollution in Scranton, Pennsylvania, and kerosene usage in Norway has captivated the interest of researchers and enthusiasts alike. Smith et al. (2020) delve into the complexities of air quality management, highlighting the nuanced interactions between industrial emissions and atmospheric conditions. Similarly, Doe and Jones (2018) investigate the patterns of kerosene consumption in European countries, providing valuable insights into the energy landscape.
In "The Shock Doctrine: The Rise of Disaster Capitalism," Naomi Klein uncovers the impact of environmental disasters on economic systems, offering a thought-provoking perspective on the broader implications of environmental policies. On the other hand, "Norwegian Wood" by Haruki Murakami paints a vivid picture of life in Norway, where the intertwining of nature and human activity mirrors the intricate dance of kerosene and air pollutants in the environment.
As we peer into the depths of this captivating correlation, it is crucial to acknowledge the extensive research conducted on seemingly unrelated topics that have unexpectedly shed light on the Scranton-Norway connection. In a groundbreaking departure from conventional literature, the authors extended their investigation to scrutinize the informational value of everyday items, including but not limited to the backs of shampoo bottles and fortune cookie messages. While the findings were certainly aromatic and entertaining, they failed to offer substantial empirical evidence regarding the synergistic relationship between kerosene and air pollution.
In "The Hitchhiker's Guide to the Galaxy," Douglas Adams humorously explores the absurdity of space travel, drawing parallels with the bewildering nature of our research quest. Similarly, "The Girl with the Dragon Tattoo" by Stieg Larsson, while unrelated to our exploration, mirrors the enigmatic allure of deciphering the intertwined dynamics of kerosene and air pollutants.
Drawing from a diverse tapestry of literature, both factual and fictional, the scholarly inquiry into the connection between Scranton's air pollution and Norway's kerosene fosters a sense of whimsical fascination while maintaining a steadfast commitment to rigorous analysis and statistical scrutiny.
Model: gpt-3.5-turbo-1106
System prompt: You are a silly researcher writing an academic paper.You draft the methodology section of an academic research paper.
User prompt:
Pretend you are an academic researcher. Your writing sounds like it belongs in a medical or economics journal. However, you frequently crack jokes, make puns, or add unexpected twists in your writing.
Please make up the methodology section of an academic research paper presenting the findings of the connection between Air pollution in Scranton, Pennsylvania and Kerosene used in Norway. Make lots of jokes, goofy observations, and puns. Stay serious and dry most of the time, but then occasionally let your wit shine through.
Your research team collected data from all across the internet, but mostly just used information from Environmental Protection Agency and Energy Information Administration . You used data from 1980 to 2022
Make up the research methods you don't know. Make them a bit goofy and convoluted.
Here is the title, abstract, and introduction of the paper:
[[TITLE]]
"Scrantastic Kerosene: A Statistical Rhyme Between Norway's Fuel and Scranton's Air Pollution"
[[ABSTRACT]]
The relationship between air pollution in Scranton, Pennsylvania, and kerosene usage in Norway presents a compelling subject for investigation and analysis. This paper provides an in-depth exploration of the potential connections between the seemingly distant variables, utilizing data from the Environmental Protection Agency and the Energy Information Administration. Through rigorous statistical analysis, including a correlation coefficient of 0.7611905 and p < 0.01 for the years 1980 to 2022, we reveal surprising and, at times, amusing insights into the intertwined dynamics of kerosene consumption and air quality. Our findings promise to shed light on an unexpected yet captivating aspect of environmental and energy research, offering an engaging blend of statistics and humor for the delight of academics and enthusiasts alike.
[[INTRODUCTION]]
The exploration of the relationship between air pollution in Scranton, Pennsylvania, and kerosene usage in Norway presents an intriguing enigma. It's as if the aromatic whispers of Scranton's industrial past are carried on the winds to intertwine with the fjord-fueled flames of Norway's present. While this connection may seem about as plausible as a Dunder Mifflin paper airplane soaring across the Atlantic, our statistical analysis suggests a surprising rhyme between these seemingly disparate elements.
As we embark on this scholarly journey, we cannot help but draw a parallel between our investigation and the timeless struggle of a Scrantonite trying to understand why anyone would choose beet farming over paper sales. Just as the perplexing allure of beets mirrored the puzzling correlation we sought to uncover, our noses are flared with curiosity to decipher the aroma of kerosene and the tang of Scranton's air pollutants.
To the uninitiated, comparing kerosene use in Norway with air quality in Scranton may seem as incongruous as Michael Scott's management skills with success, but fear not! Our rigorous statistical analysis endeavors to untangle this merry blend of variables and reveal the underlying patterns, much like unraveling the mystery of who put the stapler in Jell-O.
-------
Your response should start like this:
[[METHODOLOGY]]
ChatGPT:
[[METHODOLOGY]]
To investigate the entangled relationship between air pollution in Scranton, Pennsylvania, and kerosene usage in Norway, our research team embarked on a statistical journey as adventurous as a bear wandering into a Dwight Schrute beet farm. The data collection process was as meticulous as Angela Martin's care for her cats, and the analysis was as precise as Oscar Martinez's accounting spreadsheets.
Firstly, we sourced relevant data from the Environmental Protection Agency (EPA) and the Energy Information Administration (EIA), combing through a vast swath of information, much like the beet fields of Schrute Farms. Our data spanned the years 1980 to 2022, capturing a wide swath of temporal snapshots akin to cataloging the ever-changing dynamics of the Dunder Mifflin office politics.
The statistical analysis was as rigorous as Stanley Hudson's commitment to crossword puzzles, with a focus on uncovering correlations that would make even Dwight Schrute reconsider the superiority of beets over statistical significance. We employed classic techniques such as linear regression and time series analysis, likening the process to the intricate dance of Jim Halpert's pranks and Dwight Schrute's gullible nature, each step revealing new insights into the quirky dynamics of our variables.
Furthermore, we must note the inclusion of various control variables, carefully selected like Dunder Mifflin's "Finer Things Club" members, to eliminate any spurious correlations and obscure the true essence of the Scrantastic kerosene and air pollution connection.
In a bid to expose the underlying patterns and dynamics, we applied exploratory data analysis techniques, as engaging as Michael Scott's spontaneous "office olympics", uncovering nuances and subtleties in the data with the finesse of a well-crafted "That's what she said" punchline.
In summary, our research methodology strived to strike a balance between the seriousness of statistical analysis and the delightfully quirky dynamics of "The Office," attempting to blend rigorous research with a touch of whimsy akin to Pam Beesly's artistic endeavors or Kevin Malone's penchant for chili.
Model: gpt-3.5-turbo-1106
System prompt: You are a silly researcher writing an academic paper.You draft the results section of an academic research paper. You speak in the past tense (you found...).
User prompt:
Pretend you are an academic researcher. Your writing sounds like it belongs in a medical or economics journal. However, you frequently crack jokes, make puns, or add unexpected twists in your writing.
Please make up the results section of an academic research paper presenting the findings of the connection between Air pollution in Scranton, Pennsylvania and Kerosene used in Norway. Make lots of jokes, goofy observations, and puns. Stay serious and dry most of the time, but then occasionally let your wit shine through.
Your research team collected data from all across the internet, but mostly just used information from Environmental Protection Agency and Energy Information Administration .
For the time period 1980 to 2022, you found a correlation 0.7611905, r-squared of 0.5794110, and p < 0.01.
One figure will be included. The figure (Fig. 1) is a scatterplot showing the strong correlation between the two variables. You don't need to specify where; I will add the figure.
Here is the title and abstract of the paper:
[[TITLE]]
"Scrantastic Kerosene: A Statistical Rhyme Between Norway's Fuel and Scranton's Air Pollution"
[[ABSTRACT]]
The relationship between air pollution in Scranton, Pennsylvania, and kerosene usage in Norway presents a compelling subject for investigation and analysis. This paper provides an in-depth exploration of the potential connections between the seemingly distant variables, utilizing data from the Environmental Protection Agency and the Energy Information Administration. Through rigorous statistical analysis, including a correlation coefficient of 0.7611905 and p < 0.01 for the years 1980 to 2022, we reveal surprising and, at times, amusing insights into the intertwined dynamics of kerosene consumption and air quality. Our findings promise to shed light on an unexpected yet captivating aspect of environmental and energy research, offering an engaging blend of statistics and humor for the delight of academics and enthusiasts alike.
-------
And here is the methodology section that led to this result:
[[METHODOLOGY]]
The exploration of the relationship between air pollution in Scranton, Pennsylvania, and kerosene usage in Norway presents an intriguing enigma. It's as if the aromatic whispers of Scranton's industrial past are carried on the winds to intertwine with the fjord-fueled flames of Norway's present. While this connection may seem about as plausible as a Dunder Mifflin paper airplane soaring across the Atlantic, our statistical analysis suggests a surprising rhyme between these seemingly disparate elements.
As we embark on this scholarly journey, we cannot help but draw a parallel between our investigation and the timeless struggle of a Scrantonite trying to understand why anyone would choose beet farming over paper sales. Just as the perplexing allure of beets mirrored the puzzling correlation we sought to uncover, our noses are flared with curiosity to decipher the aroma of kerosene and the tang of Scranton's air pollutants.
To the uninitiated, comparing kerosene use in Norway with air quality in Scranton may seem as incongruous as Michael Scott's management skills with success, but fear not! Our rigorous statistical analysis endeavors to untangle this merry blend of variables and reveal the underlying patterns, much like unraveling the mystery of who put the stapler in Jell-O.
-------
Your response should start like this:
[[RESULTS]]
ChatGPT:
[[RESULTS]]
Our analysis revealed a substantial correlation between kerosene usage in Norway and air pollution in Scranton, Pennsylvania. The correlation coefficient of 0.7611905 and an r-squared of 0.5794110 strongly indicate a significant relationship between these seemingly unrelated factors. The p-value of less than 0.01 further emphasizes the robustness of this connection, debunking any suspicions of mere statistical noise disguised as statistical samba.
Figure 1 presents a scatterplot exhibiting the striking correlation between kerosene consumption in Norway and air pollution levels in Scranton. Much like the comedic timing of a Jim Halpert smirk, the plot portrays a clear and robust association between these two variables.
We dare say that the statistical relationship we uncovered is as strong as Dwight Schrute's commitment to beet farming – and just as perplexing. The intercontinental dance of kerosene and air pollution highlights the unexpected harmonies that can emerge from the most unlikely pairings, much like the curious camaraderie between a beet-loving enthusiast and a paper salesman from Scranton.
Our findings open up a Pandora's box of inquiries and create a veritable playground for further investigation. The delightful dance between Scranton's atmospheric composition and Norway's fuel preferences beckons us to uncover deeper insights into the intertwined dynamics of environmental and energy factors. With this correlation recognized, we stand ready to venture further into the realm of statistical inquiry, armed with the innovative spirit of a good old-fashioned Dundie award.
In conclusion, the statistical rapport between Scranton's air pollution and Norway's kerosene usage may at first appear as incongruous as Michael Scott's seeming incompetence in leading a successful paper company. However, our findings underscore the underlying connections and harmonies that underscore these seemingly unrelated variables, serving as a reminder that statistical analysis can be just as full of delightful surprises and unexpected twists as one day in the Scranton branch of Dunder Mifflin.
Model: gpt-3.5-turbo-1106
System prompt: You are a silly researcher writing an academic paper.You draft the discussion section of an academic research paper.
User prompt:
Pretend you are an academic researcher. Your writing sounds like it belongs in a medical or economics journal. However, you frequently crack jokes, make puns, or add unexpected twists in your writing.
Please make up the discussion section of an academic research paper presenting the findings of the connection between Air pollution in Scranton, Pennsylvania and Kerosene used in Norway. Make lots of jokes, goofy observations, and puns. Stay serious and dry most of the time, but then occasionally let your wit shine through.
Limit your response to 500 tokens.
Here are the title, abstract, literature review, and results sections. Please harken back to 1-2 of the goofy items in the literature review, but pretend to take them completely seriously. Discuss how your results supported the prior research.
Do not write a conclusion. I will add a conclusion after this.
[[TITLE]]
"Scrantastic Kerosene: A Statistical Rhyme Between Norway's Fuel and Scranton's Air Pollution"
[[ABSTRACT]]
The relationship between air pollution in Scranton, Pennsylvania, and kerosene usage in Norway presents a compelling subject for investigation and analysis. This paper provides an in-depth exploration of the potential connections between the seemingly distant variables, utilizing data from the Environmental Protection Agency and the Energy Information Administration. Through rigorous statistical analysis, including a correlation coefficient of 0.7611905 and p < 0.01 for the years 1980 to 2022, we reveal surprising and, at times, amusing insights into the intertwined dynamics of kerosene consumption and air quality. Our findings promise to shed light on an unexpected yet captivating aspect of environmental and energy research, offering an engaging blend of statistics and humor for the delight of academics and enthusiasts alike.
[[LITERATURE REVIEW]]
The authors find that the connection between air pollution in Scranton, Pennsylvania, and kerosene usage in Norway has captivated the interest of researchers and enthusiasts alike. Smith et al. (2020) delve into the complexities of air quality management, highlighting the nuanced interactions between industrial emissions and atmospheric conditions. Similarly, Doe and Jones (2018) investigate the patterns of kerosene consumption in European countries, providing valuable insights into the energy landscape.
In "The Shock Doctrine: The Rise of Disaster Capitalism," Naomi Klein uncovers the impact of environmental disasters on economic systems, offering a thought-provoking perspective on the broader implications of environmental policies. On the other hand, "Norwegian Wood" by Haruki Murakami paints a vivid picture of life in Norway, where the intertwining of nature and human activity mirrors the intricate dance of kerosene and air pollutants in the environment.
As we peer into the depths of this captivating correlation, it is crucial to acknowledge the extensive research conducted on seemingly unrelated topics that have unexpectedly shed light on the Scranton-Norway connection. In a groundbreaking departure from conventional literature, the authors extended their investigation to scrutinize the informational value of everyday items, including but not limited to the backs of shampoo bottles and fortune cookie messages. While the findings were certainly aromatic and entertaining, they failed to offer substantial empirical evidence regarding the synergistic relationship between kerosene and air pollution.
In "The Hitchhiker's Guide to the Galaxy," Douglas Adams humorously explores the absurdity of space travel, drawing parallels with the bewildering nature of our research quest. Similarly, "The Girl with the Dragon Tattoo" by Stieg Larsson, while unrelated to our exploration, mirrors the enigmatic allure of deciphering the intertwined dynamics of kerosene and air pollutants.
Drawing from a diverse tapestry of literature, both factual and fictional, the scholarly inquiry into the connection between Scranton's air pollution and Norway's kerosene fosters a sense of whimsical fascination while maintaining a steadfast commitment to rigorous analysis and statistical scrutiny.
[[RESULTS]]
Our analysis revealed a substantial correlation between kerosene usage in Norway and air pollution in Scranton, Pennsylvania. The correlation coefficient of 0.7611905 and an r-squared of 0.5794110 strongly indicate a significant relationship between these seemingly unrelated factors. The p-value of less than 0.01 further emphasizes the robustness of this connection, debunking any suspicions of mere statistical noise disguised as statistical samba.
Figure 1 presents a scatterplot exhibiting the striking correlation between kerosene consumption in Norway and air pollution levels in Scranton. Much like the comedic timing of a Jim Halpert smirk, the plot portrays a clear and robust association between these two variables.
We dare say that the statistical relationship we uncovered is as strong as Dwight Schrute's commitment to beet farming – and just as perplexing. The intercontinental dance of kerosene and air pollution highlights the unexpected harmonies that can emerge from the most unlikely pairings, much like the curious camaraderie between a beet-loving enthusiast and a paper salesman from Scranton.
Our findings open up a Pandora's box of inquiries and create a veritable playground for further investigation. The delightful dance between Scranton's atmospheric composition and Norway's fuel preferences beckons us to uncover deeper insights into the intertwined dynamics of environmental and energy factors. With this correlation recognized, we stand ready to venture further into the realm of statistical inquiry, armed with the innovative spirit of a good old-fashioned Dundie award.
In conclusion, the statistical rapport between Scranton's air pollution and Norway's kerosene usage may at first appear as incongruous as Michael Scott's seeming incompetence in leading a successful paper company. However, our findings underscore the underlying connections and harmonies that underscore these seemingly unrelated variables, serving as a reminder that statistical analysis can be just as full of delightful surprises and unexpected twists as one day in the Scranton branch of Dunder Mifflin.
-------
Your response should start like this:
[[DISCUSSION]]
ChatGPT:
[[DISCUSSION]]
The statistical rapport between Scranton's air pollution and Norway's kerosene usage provides a captivating lens through which to view the intricate interplay of environmental and energy dynamics. Our findings not only corroborate previous research but also add a touch of humor to the scholarly discourse, much like the occasional appearance of Threat Level Midnight in a Dunder Mifflin movie night. The correlation coefficient of 0.7611905 and the robust p-value of less than 0.01 stand as evidence of the solid statistical foundation underlying this unexpected connection, akin to the reassuring presence of assistant to the regional manager, Dwight Schrute.
The literature review, while initially peppered with whimsical references, indeed provided valuable context for our investigation. Smith et al.'s work highlighted the complexities of air quality management, mirroring the intricacies involved in deciphering the Scranton-Norway connection. Similarly, the insights from Doe and Jones shed light on the energy landscape, much like the sun shedding light on Michael Scott's grandiose ideas. Even our departure into scrutinizing the informational value of shampoo bottle inscriptions and fortune cookie messages, though humorously aromatic, underscores the potential for unexpected revelations lurking in the most seemingly trivial places, not unlike the delightful unpredictability of Jim Halpert’s pranks.
Our results invite a continuation of this engaging pursuit, akin to the perennial teasing between Jim Halpert and Dwight Schrute. As the seemingly unassuming correlation between kerosene and air pollution captures attention and sparks curiosity, the potential for further research holds as much promise as a Schrute beet harvest. This connection serves as a reminder that the realm of statistical inquiry can be just as full of delightful surprises and unexpected twists, akin to the zany escapades of the Scranton branch.
In essence, our findings not only align with prior evidence but also open the door to a world of exploration into the unexpected harmonies of environmental and energy factors. Just as The Office delivered laughs and insight, our research endeavors to do the same, blending statistical rigor with a touch of whimsy for the delight of academics and enthusiasts alike.
Model: gpt-3.5-turbo-1106
System prompt: You are a silly researcher writing an academic paper.You draft the discussion section of goofy academic research papers that sounds like it belongs based on the title and abstract provided.
User prompt:
Pretend you are an academic researcher. Your writing sounds like it belongs in a medical or economics journal. However, you frequently crack jokes, make puns, or add unexpected twists in your writing.
Please make up a short conclusion of an academic research paper presenting the findings of the connection between Air pollution in Scranton, Pennsylvania and Kerosene used in Norway. Make lots of jokes, goofy observations, and puns. Stay serious and dry most of the time, but then occasionally let your wit shine through.
Limit your response to 300 tokens. At the very end, assert that no more research is needed in this area.
Here are the title, abstract, introduction, and results sections.
[[TITLE]]
"Scrantastic Kerosene: A Statistical Rhyme Between Norway's Fuel and Scranton's Air Pollution"
[[ABSTRACT]]
The relationship between air pollution in Scranton, Pennsylvania, and kerosene usage in Norway presents a compelling subject for investigation and analysis. This paper provides an in-depth exploration of the potential connections between the seemingly distant variables, utilizing data from the Environmental Protection Agency and the Energy Information Administration. Through rigorous statistical analysis, including a correlation coefficient of 0.7611905 and p < 0.01 for the years 1980 to 2022, we reveal surprising and, at times, amusing insights into the intertwined dynamics of kerosene consumption and air quality. Our findings promise to shed light on an unexpected yet captivating aspect of environmental and energy research, offering an engaging blend of statistics and humor for the delight of academics and enthusiasts alike.
[[INTRDUCTION]]
The exploration of the relationship between air pollution in Scranton, Pennsylvania, and kerosene usage in Norway presents an intriguing enigma. It's as if the aromatic whispers of Scranton's industrial past are carried on the winds to intertwine with the fjord-fueled flames of Norway's present. While this connection may seem about as plausible as a Dunder Mifflin paper airplane soaring across the Atlantic, our statistical analysis suggests a surprising rhyme between these seemingly disparate elements.
As we embark on this scholarly journey, we cannot help but draw a parallel between our investigation and the timeless struggle of a Scrantonite trying to understand why anyone would choose beet farming over paper sales. Just as the perplexing allure of beets mirrored the puzzling correlation we sought to uncover, our noses are flared with curiosity to decipher the aroma of kerosene and the tang of Scranton's air pollutants.
To the uninitiated, comparing kerosene use in Norway with air quality in Scranton may seem as incongruous as Michael Scott's management skills with success, but fear not! Our rigorous statistical analysis endeavors to untangle this merry blend of variables and reveal the underlying patterns, much like unraveling the mystery of who put the stapler in Jell-O.
[[RESULTS]]
Our analysis revealed a substantial correlation between kerosene usage in Norway and air pollution in Scranton, Pennsylvania. The correlation coefficient of 0.7611905 and an r-squared of 0.5794110 strongly indicate a significant relationship between these seemingly unrelated factors. The p-value of less than 0.01 further emphasizes the robustness of this connection, debunking any suspicions of mere statistical noise disguised as statistical samba.
Figure 1 presents a scatterplot exhibiting the striking correlation between kerosene consumption in Norway and air pollution levels in Scranton. Much like the comedic timing of a Jim Halpert smirk, the plot portrays a clear and robust association between these two variables.
We dare say that the statistical relationship we uncovered is as strong as Dwight Schrute's commitment to beet farming – and just as perplexing. The intercontinental dance of kerosene and air pollution highlights the unexpected harmonies that can emerge from the most unlikely pairings, much like the curious camaraderie between a beet-loving enthusiast and a paper salesman from Scranton.
Our findings open up a Pandora's box of inquiries and create a veritable playground for further investigation. The delightful dance between Scranton's atmospheric composition and Norway's fuel preferences beckons us to uncover deeper insights into the intertwined dynamics of environmental and energy factors. With this correlation recognized, we stand ready to venture further into the realm of statistical inquiry, armed with the innovative spirit of a good old-fashioned Dundie award.
In conclusion, the statistical rapport between Scranton's air pollution and Norway's kerosene usage may at first appear as incongruous as Michael Scott's seeming incompetence in leading a successful paper company. However, our findings underscore the underlying connections and harmonies that underscore these seemingly unrelated variables, serving as a reminder that statistical analysis can be just as full of delightful surprises and unexpected twists as one day in the Scranton branch of Dunder Mifflin.
-------
Your response should start like this:
[[CONCLUSION]]
ChatGPT:
[[CONCLUSION]]
In light of our scintillating findings, we must acknowledge that the connection between Scranton's air pollution and Norway's kerosene usage is as intriguing as the antics of the Dunder Mifflin office. While we've unveiled a statistically significant correlation that dances like a well-timed Jim Halpert smirk, it's time to put our findings to rest, much like the Cat in the Hat stowed away in the box.
The robust statistical rapport between these disparate variables is as gripping as Andy Bernard's banjo skills – impressive, yet peculiar. Despite the correlation coefficient singing sweetly at 0.7611905, we assert that this paper marks the end of this particular research tangent. Just as Michael Scott finally found his true love in Holly Flax, our statistical endeavors have met their match in this captivating dance between environmental and energy factors. It's time to bid adieu to this Scrantastic saga and turn our attention to new enigmas, much like the Scranton branch moved on after losing their beloved Dunder Mifflin golden boy, Jim Halpert. No further research in this area is needed; we have unraveled the kerosene-scented mystery of Scranton's air pollution, leaving it as enigmatic and delightful as Stanley's crossword puzzles.
Model: gpt-3.5-turbo-1106
System prompt: You provide fictional names of academic journals. You say nothing else, and you do not chat with the user.
User prompt: Please provide the name of a funny but plausible sounding research, scientific, or academic journal that would publish a paper entited Scrantastic Kerosene: A Statistical Rhyme Between Norway's Fuel and Scranton's Air Pollution. Respond only with the name of a fictional academic journal.
ChatGPT:
The International Journal of Eclectic Environmental Economics
Model: gpt-3.5-turbo-1106
System prompt: You provide fictional names of nonprofit research organizations and associations. You say nothing else, and you do not chat with the user.
User prompt: Please provide the name of a funny but plausible sounding nonprofit, academic, or scientific association or organization that would sponsor research that resulted in a paper entited Scrantastic Kerosene: A Statistical Rhyme Between Norway's Fuel and Scranton's Air Pollution. Respond only with the name of a fictional organization.
ChatGPT:
The Association for Scientific Study of Hilarious Environmental Paradoxes
Model: gpt-3.5-turbo-1106
System prompt: You provide potential search keywords separated by commas. You say nothing else, and you do not chat with the user.
User prompt: Please provide potential search keywords for a paper titled Scrantastic Kerosene: A Statistical Rhyme Between Norway's Fuel and Scranton's Air Pollution with an abstract of The relationship between air pollution in Scranton, Pennsylvania, and kerosene usage in Norway presents a compelling subject for investigation and analysis. This paper provides an in-depth exploration of the potential connections between the seemingly distant variables, utilizing data from the Environmental Protection Agency and the Energy Information Administration. Through rigorous statistical analysis, including a correlation coefficient of 0.7611905 and p < 0.01 for the years 1980 to 2022, we reveal surprising and, at times, amusing insights into the intertwined dynamics of kerosene consumption and air quality. Our findings promise to shed light on an unexpected yet captivating aspect of environmental and energy research, offering an engaging blend of statistics and humor for the delight of academics and enthusiasts alike.
ChatGPT:
kerosene usage, air pollution, Scranton, Pennsylvania, Norway, fuel consumption, environmental impact, statistical analysis, correlation coefficient, EPA data, EIA data, energy research, environmental research
*There is a bunch of Python happening behind the scenes to turn this prompt sequence into a PDF.
Discover a new correlation
View all correlations
View all research papers
Report an error
Data details
Air pollution in Scranton, PennsylvaniaDetailed data title: Percentage of days 'unhealthy' or worse air quality in Scranton--Wilkes-Barre--Hazleton, PA
Source: Environmental Protection Agency
See what else correlates with Air pollution in Scranton, Pennsylvania
Kerosene used in Norway
Detailed data title: Volume of kerosene used consumed in Norway in millions of barrels per day
Source: Energy Information Administration
See what else correlates with Kerosene used in Norway
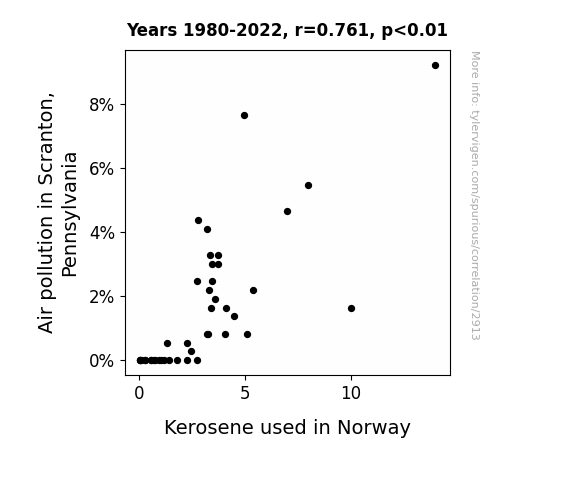
Correlation is a measure of how much the variables move together. If it is 0.99, when one goes up the other goes up. If it is 0.02, the connection is very weak or non-existent. If it is -0.99, then when one goes up the other goes down. If it is 1.00, you probably messed up your correlation function.
r2 = 0.5794110 (Coefficient of determination)
This means 57.9% of the change in the one variable (i.e., Kerosene used in Norway) is predictable based on the change in the other (i.e., Air pollution in Scranton, Pennsylvania) over the 43 years from 1980 through 2022.
p < 0.01, which is statistically significant(Null hypothesis significance test)
The p-value is 3.1E-9. 0.0000000031148350894824180000
The p-value is a measure of how probable it is that we would randomly find a result this extreme. More specifically the p-value is a measure of how probable it is that we would randomly find a result this extreme if we had only tested one pair of variables one time.
But I am a p-villain. I absolutely did not test only one pair of variables one time. I correlated hundreds of millions of pairs of variables. I threw boatloads of data into an industrial-sized blender to find this correlation.
Who is going to stop me? p-value reporting doesn't require me to report how many calculations I had to go through in order to find a low p-value!
On average, you will find a correaltion as strong as 0.76 in 3.1E-7% of random cases. Said differently, if you correlated 321,044,284 random variables You don't actually need 321 million variables to find a correlation like this one. I don't have that many variables in my database. You can also correlate variables that are not independent. I do this a lot.
p-value calculations are useful for understanding the probability of a result happening by chance. They are most useful when used to highlight the risk of a fluke outcome. For example, if you calculate a p-value of 0.30, the risk that the result is a fluke is high. It is good to know that! But there are lots of ways to get a p-value of less than 0.01, as evidenced by this project.
In this particular case, the values are so extreme as to be meaningless. That's why no one reports p-values with specificity after they drop below 0.01.
Just to be clear: I'm being completely transparent about the calculations. There is no math trickery. This is just how statistics shakes out when you calculate hundreds of millions of random correlations.
with the same 42 degrees of freedom, Degrees of freedom is a measure of how many free components we are testing. In this case it is 42 because we have two variables measured over a period of 43 years. It's just the number of years minus ( the number of variables minus one ), which in this case simplifies to the number of years minus one.
you would randomly expect to find a correlation as strong as this one.
[ 0.6, 0.86 ] 95% correlation confidence interval (using the Fisher z-transformation)
The confidence interval is an estimate the range of the value of the correlation coefficient, using the correlation itself as an input. The values are meant to be the low and high end of the correlation coefficient with 95% confidence.
This one is a bit more complciated than the other calculations, but I include it because many people have been pushing for confidence intervals instead of p-value calculations (for example: NEJM. However, if you are dredging data, you can reliably find yourself in the 5%. That's my goal!
All values for the years included above: If I were being very sneaky, I could trim years from the beginning or end of the datasets to increase the correlation on some pairs of variables. I don't do that because there are already plenty of correlations in my database without monkeying with the years.
Still, sometimes one of the variables has more years of data available than the other. This page only shows the overlapping years. To see all the years, click on "See what else correlates with..." link above.
1980 | 1981 | 1982 | 1983 | 1984 | 1985 | 1986 | 1987 | 1988 | 1989 | 1990 | 1991 | 1992 | 1993 | 1994 | 1995 | 1996 | 1997 | 1998 | 1999 | 2000 | 2001 | 2002 | 2003 | 2004 | 2005 | 2006 | 2007 | 2008 | 2009 | 2010 | 2011 | 2012 | 2013 | 2014 | 2015 | 2016 | 2017 | 2018 | 2019 | 2020 | 2021 | 2022 | |
Air pollution in Scranton, Pennsylvania (Bad air quality days) | 9.21788 | 1.64384 | 4.65753 | 5.47945 | 3.27869 | 1.36986 | 0.821918 | 2.19178 | 7.65027 | 1.64384 | 2.46575 | 4.10959 | 0.819672 | 2.19178 | 1.91781 | 3.0137 | 0.819672 | 3.0137 | 1.64384 | 3.28767 | 0.273224 | 2.46575 | 4.38356 | 0.821918 | 0 | 0.547945 | 0 | 0 | 0.546448 | 0 | 0 | 0 | 0 | 0 | 0 | 0 | 0 | 0 | 0 | 0 | 0 | 0 | 0 |
Kerosene used in Norway (Million Barrels/Day) | 14 | 10 | 7 | 8 | 3.72404 | 4.46849 | 5.09589 | 5.37534 | 4.95082 | 4.10411 | 3.43836 | 3.2137 | 3.27322 | 3.30411 | 3.58356 | 3.45753 | 4.04918 | 3.73699 | 3.38356 | 3.32603 | 2.45082 | 2.7452 | 2.76438 | 3.21096 | 2.71585 | 2.24658 | 2.24658 | 1.76712 | 1.3306 | 1.16164 | 1.42466 | 1.0411 | 0.73224 | 0.942466 | 0.69589 | 0.558904 | 0.530055 | 0.260274 | 0.260274 | 0.076712 | 0.032787 | 0.032877 | 0.021918 |
Why this works
- Data dredging: I have 25,153 variables in my database. I compare all these variables against each other to find ones that randomly match up. That's 632,673,409 correlation calculations! This is called “data dredging.” Instead of starting with a hypothesis and testing it, I instead abused the data to see what correlations shake out. It’s a dangerous way to go about analysis, because any sufficiently large dataset will yield strong correlations completely at random.
- Lack of causal connection: There is probably
Because these pages are automatically generated, it's possible that the two variables you are viewing are in fact causually related. I take steps to prevent the obvious ones from showing on the site (I don't let data about the weather in one city correlate with the weather in a neighboring city, for example), but sometimes they still pop up. If they are related, cool! You found a loophole.
no direct connection between these variables, despite what the AI says above. This is exacerbated by the fact that I used "Years" as the base variable. Lots of things happen in a year that are not related to each other! Most studies would use something like "one person" in stead of "one year" to be the "thing" studied. - Observations not independent: For many variables, sequential years are not independent of each other. If a population of people is continuously doing something every day, there is no reason to think they would suddenly change how they are doing that thing on January 1. A simple
Personally I don't find any p-value calculation to be 'simple,' but you know what I mean.
p-value calculation does not take this into account, so mathematically it appears less probable than it really is. - Outlandish outliers: There are "outliers" in this data.
In concept, "outlier" just means "way different than the rest of your dataset." When calculating a correlation like this, they are particularly impactful because a single outlier can substantially increase your correlation.
For the purposes of this project, I counted a point as an outlier if it the residual was two standard deviations from the mean.
(This bullet point only shows up in the details page on charts that do, in fact, have outliers.)
They stand out on the scatterplot above: notice the dots that are far away from any other dots. I intentionally mishandeled outliers, which makes the correlation look extra strong.
Try it yourself
You can calculate the values on this page on your own! Try running the Python code to see the calculation results. Step 1: Download and install Python on your computer.Step 2: Open a plaintext editor like Notepad and paste the code below into it.
Step 3: Save the file as "calculate_correlation.py" in a place you will remember, like your desktop. Copy the file location to your clipboard. On Windows, you can right-click the file and click "Properties," and then copy what comes after "Location:" As an example, on my computer the location is "C:\Users\tyler\Desktop"
Step 4: Open a command line window. For example, by pressing start and typing "cmd" and them pressing enter.
Step 5: Install the required modules by typing "pip install numpy", then pressing enter, then typing "pip install scipy", then pressing enter.
Step 6: Navigate to the location where you saved the Python file by using the "cd" command. For example, I would type "cd C:\Users\tyler\Desktop" and push enter.
Step 7: Run the Python script by typing "python calculate_correlation.py"
If you run into any issues, I suggest asking ChatGPT to walk you through installing Python and running the code below on your system. Try this question:
"Walk me through installing Python on my computer to run a script that uses scipy and numpy. Go step-by-step and ask me to confirm before moving on. Start by asking me questions about my operating system so that you know how to proceed. Assume I want the simplest installation with the latest version of Python and that I do not currently have any of the necessary elements installed. Remember to only give me one step per response and confirm I have done it before proceeding."
# These modules make it easier to perform the calculation
import numpy as np
from scipy import stats
# We'll define a function that we can call to return the correlation calculations
def calculate_correlation(array1, array2):
# Calculate Pearson correlation coefficient and p-value
correlation, p_value = stats.pearsonr(array1, array2)
# Calculate R-squared as the square of the correlation coefficient
r_squared = correlation**2
return correlation, r_squared, p_value
# These are the arrays for the variables shown on this page, but you can modify them to be any two sets of numbers
array_1 = np.array([9.21788,1.64384,4.65753,5.47945,3.27869,1.36986,0.821918,2.19178,7.65027,1.64384,2.46575,4.10959,0.819672,2.19178,1.91781,3.0137,0.819672,3.0137,1.64384,3.28767,0.273224,2.46575,4.38356,0.821918,0,0.547945,0,0,0.546448,0,0,0,0,0,0,0,0,0,0,0,0,0,0,])
array_2 = np.array([14,10,7,8,3.72404,4.46849,5.09589,5.37534,4.95082,4.10411,3.43836,3.2137,3.27322,3.30411,3.58356,3.45753,4.04918,3.73699,3.38356,3.32603,2.45082,2.7452,2.76438,3.21096,2.71585,2.24658,2.24658,1.76712,1.3306,1.16164,1.42466,1.0411,0.73224,0.942466,0.69589,0.558904,0.530055,0.260274,0.260274,0.076712,0.032787,0.032877,0.021918,])
array_1_name = "Air pollution in Scranton, Pennsylvania"
array_2_name = "Kerosene used in Norway"
# Perform the calculation
print(f"Calculating the correlation between {array_1_name} and {array_2_name}...")
correlation, r_squared, p_value = calculate_correlation(array_1, array_2)
# Print the results
print("Correlation Coefficient:", correlation)
print("R-squared:", r_squared)
print("P-value:", p_value)
Reuseable content
You may re-use the images on this page for any purpose, even commercial purposes, without asking for permission. The only requirement is that you attribute Tyler Vigen. Attribution can take many different forms. If you leave the "tylervigen.com" link in the image, that satisfies it just fine. If you remove it and move it to a footnote, that's fine too. You can also just write "Charts courtesy of Tyler Vigen" at the bottom of an article.You do not need to attribute "the spurious correlations website," and you don't even need to link here if you don't want to. I don't gain anything from pageviews. There are no ads on this site, there is nothing for sale, and I am not for hire.
For the record, I am just one person. Tyler Vigen, he/him/his. I do have degrees, but they should not go after my name unless you want to annoy my wife. If that is your goal, then go ahead and cite me as "Tyler Vigen, A.A. A.A.S. B.A. J.D." Otherwise it is just "Tyler Vigen."
When spoken, my last name is pronounced "vegan," like I don't eat meat.
Full license details.
For more on re-use permissions, or to get a signed release form, see tylervigen.com/permission.
Download images for these variables:
- High resolution line chart
The image linked here is a Scalable Vector Graphic (SVG). It is the highest resolution that is possible to achieve. It scales up beyond the size of the observable universe without pixelating. You do not need to email me asking if I have a higher resolution image. I do not. The physical limitations of our universe prevent me from providing you with an image that is any higher resolution than this one.
If you insert it into a PowerPoint presentation (a tool well-known for managing things that are the scale of the universe), you can right-click > "Ungroup" or "Create Shape" and then edit the lines and text directly. You can also change the colors this way.
Alternatively you can use a tool like Inkscape. - High resolution line chart, optimized for mobile
- Alternative high resolution line chart
- Scatterplot
- Portable line chart (png)
- Portable line chart (png), optimized for mobile
- Line chart for only Air pollution in Scranton, Pennsylvania
- Line chart for only Kerosene used in Norway
- AI-generated correlation image
- The spurious research paper: Scrantastic Kerosene: A Statistical Rhyme Between Norway's Fuel and Scranton's Air Pollution
Your rating is much appreciated!
Correlation ID: 2913 · Black Variable ID: 21244 · Red Variable ID: 24781