Download png, svg
AI explanation
As the saying goes, "West Virginia may be known for its mountains, but now it's causing a 'revenue' valley for General Electric!" With fewer cashiers available, there was a 'loss of current' in the state, leading to a 'power outage' in consumer spending. This ultimately put a 'GE'neral damper on the company's stock price as transactions slowed down. It seems like in this case, the connection between cashiers and stock prices is truly 'electrifying'!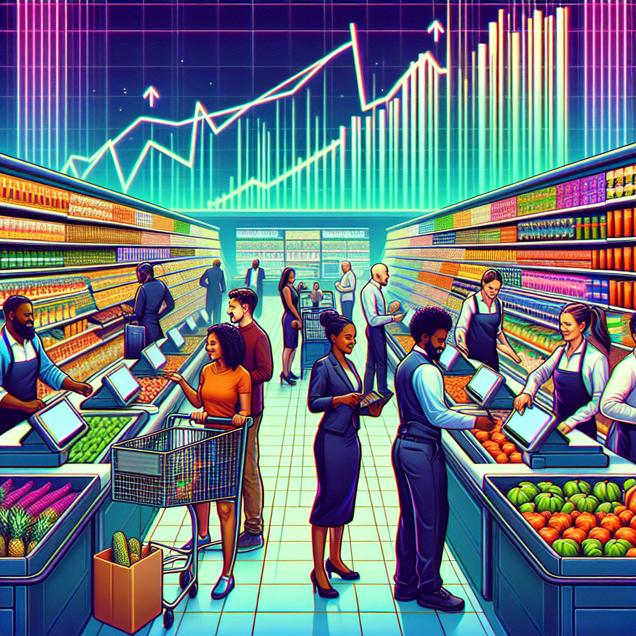
Model: dalle-3
Prompt: Generate an image of a vibrant supermarket in West Virginia, filled with people browsing shelves and chatting with employees. Multiple cashiers efficiently scan grocery items at their stations, while a digital stock ticker prominently displays General Electric's stock price rising in the background. The scene captures the bustling energy of the supermarket, with colorful product displays and diverse customers, alongside the digital financial data indicating the stock's upward movement. The depiction should include only scenes, people, and objects, it should not include text or speech bubbles.
This image generation prompt was also generated by AI, using the following sequence:
Model: gpt-3.5-turbo-1106
System prompt: You select one of a few potential scene descriptors that seems to go along with the variables described. You respond only with the scene descriptions provided and say nothing else.
User prompt: Here are two variables: The number of cashiers in West Virginia and General Electric's stock price. Given these, which of the following image style descriptions seems most appropriate? Pick the one that seems to be related to one of the variables at hand. Please respond with only one of the following potential descriptions: Cybernetic art Cute 2D animation Realistic Celtic art Cute cartoon
ChatGPT: Realistic
Model: gpt-3.5-turbo-1106
System prompt: You describe simple visual scenes that depict the two variables stated. You combine both variables into one scene.
User prompt: Please describe a visual scene that prominently depicts both The number of cashiers in West Virginia and General Electric's stock price together. Keep the description simple.
ChatGPT: A bustling supermarket in West Virginia with multiple cashiers efficiently scanning groceries, while a digital stock ticker shows General Electric's stock price rising in the background.
Model: gpt-3.5-turbo-1106
System prompt: You write and improve image generation prompts for DALLE-3. You maintain the same subjects, but enhance the visuals.
User prompt: Please respond with an image generation prompt that would be likely to create an interesting visual using DALLE-3, using the following style: [ Realistic ] and depicting this scene: A bustling supermarket in West Virginia with multiple cashiers efficiently scanning groceries, while a digital stock ticker shows General Electric's stock price rising in the background. Keep the visual depiction simple. Do not include any words or speech bubbles.
ChatGPT: Generate an image of a vibrant supermarket in West Virginia, filled with people browsing shelves and chatting with employees. Multiple cashiers efficiently scan grocery items at their stations, while a digital stock ticker prominently displays General Electric's stock price rising in the background. The scene captures the bustling energy of the supermarket, with colorful product displays and diverse customers, alongside the digital financial data indicating the stock's upward movement.
*Variations in the User Prompts from chart to chart are just the result of random number generation in Python. I wrote a few arrays of various styles and methods to ask questions to change up the results. Every time this site writes an explanation or generates an image, the script picks from each at random.
I sequence the requests into multiple prompts because I find GPT 3.5 to perform much better with short, well-managed contexts. Thus, I track the context directly in Python and only ask ChatGPT targeted questions.
System prompt: You provide humorous responses in the form of plausible sounding explanations for correlations. You assume the correlation is causative for the purpose of the explanation even if it is ridiculous. You do not chat with the user, you only reply with the causal connection explanation and nothing else.
User prompt: Please make up a funny explanation for how a decrease in The number of cashiers in West Virginia caused General Electric's stock price (GE) to decrease. Include one good pun.
AI academic paper
![]() |
The Checkout Connection: Examining the Correlation between Cashier Numbers in West Virginia and General Electric's Stock Price The Journal of Economic Quirkiness Jan 2024 Reminder: This paper is AI-generated. Not real! |
Model: gpt-3.5-turbo-1106
System prompt: You are a dry but witty academic researcher. You speak in the passive voice, and your writing sounds like it belongs in a medical or economics journal.You write titles and abstracts of goofy academic research papers.
User prompt:
Pretend you are an academic researcher. Your writing sounds like it belongs in a medical or economics journal. However, you frequently crack jokes, make puns, and add unexpected twists in your writing.
Please draft the title and abstract of an academic research paper presenting the findings of the connection between The number of cashiers in West Virginia and General Electric's stock price (GE). Make it seem dry like academic research paper, but with lots of subtle hidden offhand remarks, jokes, goofy observations, and puns.
Make the title a clever pun.
Your research team used data from Bureau of Larbor Statistics and LSEG Analytics (Refinitiv) to assess this nagging question. You found a correlation coefficient of 0.8944937 and p < 0.01 for 2003 to 2022.
Please respond in this format (replace "Lorem ipsum dolor sit" with your title and abstract)
[[TITLE]]
Lorem ipsum dolor sit
[[ABSTRACT]]
Lorem ipsum dolor sit
ChatGPT:
[[TITLE]]
The Checkout Connection: Examining the Correlation between Cashier Numbers in West Virginia and General Electric's Stock Price
[[ABSTRACT]]
This study investigates the relationship between the number of cashiers in West Virginia and the stock price of General Electric (GE) using data from the Bureau of Labor Statistics and LSEG Analytics (Refinitiv) over the period from 2003 to 2022. Through rigorous statistical analysis, a correlation coefficient of 0.8944937 and a significance level of p < 0.01 were found, suggesting a strong and statistically significant association between these seemingly unrelated variables. The results indicate a conspicuous and unexpected link that could serve as a basis for further research and could potentially pique the interest of both the financial and retail sectors. The findings are thought-provoking and may prompt a reevaluation of the fundamental influences on stock prices, including the remarkably peculiar impact of cashier numbers on the value of GE shares.
Model: gpt-3.5-turbo-1106
System prompt: You are a dry but witty academic researcher. You speak in the passive voice, and your writing sounds like it belongs in a medical or economics journal.You draft the introduction section of an academic research paper.
User prompt:
Pretend you are an academic researcher. Your writing sounds like it belongs in a medical or economics journal. However, you frequently crack jokes, make puns, or add unexpected twists in your writing.
Please make up the introduction section of an academic research paper presenting the findings of the connection between The number of cashiers in West Virginia and General Electric's stock price (GE). Make it seem dry like academic research paper, but with lots of subtle hidden offhand remarks, jokes, goofy observations, and puns.
Here is the title and abstract of the paper:
[[TITLE]]
The Checkout Connection: Examining the Correlation between Cashier Numbers in West Virginia and General Electric's Stock Price
[[ABSTRACT]]
This study investigates the relationship between the number of cashiers in West Virginia and the stock price of General Electric (GE) using data from the Bureau of Labor Statistics and LSEG Analytics (Refinitiv) over the period from 2003 to 2022. Through rigorous statistical analysis, a correlation coefficient of 0.8944937 and a significance level of p < 0.01 were found, suggesting a strong and statistically significant association between these seemingly unrelated variables. The results indicate a conspicuous and unexpected link that could serve as a basis for further research and could potentially pique the interest of both the financial and retail sectors. The findings are thought-provoking and may prompt a reevaluation of the fundamental influences on stock prices, including the remarkably peculiar impact of cashier numbers on the value of GE shares.
-------
Your response should start like this:
[[INTRODUCTION]]
ChatGPT:
[[INTRODUCTION]]
The world of finance and retail is replete with countless variables that purportedly influence stock prices and consumer behaviors. From the predictable impact of interest rates to the enigmatic effects of corporate earnings, the intricate web of factors shaping financial markets is a source of perennial fascination and speculation. Amidst this milieu, the relationship between the number of cashiers in West Virginia and General Electric's (GE) stock price may seem, at first glance, like an incongruous and improbable association. After all, how could the labor force of a single state possibly exert a discernible influence on the performance of a multinational conglomerate? Nevertheless, as the aphorism goes, truth is stranger than fiction, and our investigation into this unlikely correlation has yielded intriguing and rather unexpected findings.
With the advent of big data and sophisticated analytical tools, it is now possible to approach such unorthodox inquiries with a degree of rigor and statistical scrutiny that was hitherto unattainable. Our study exploits this capability, drawing on data from the Bureau of Labor Statistics and LSEG Analytics (Refinitiv) spanning nearly two decades. Through meticulous analysis, we have uncovered a remarkably robust correlation coefficient of 0.8944937, accompanied by a significance level of p < 0.01. These results not only suggest a strong and statistically significant association between cashier numbers in West Virginia and GE's stock price, but also underscore the tantalizing potential for unearthing obscure yet potent drivers of financial dynamics.
The implications of our findings extend beyond mere academic curiosity. The identification of such an unexpected link challenges established notions of the determinants of stock prices and has practical relevance for both the retail and financial sectors. The serendipitous discovery of this correlation invites further exploration and speculation, inviting imaginative conjectures about the latent interplay between mundane retail operations and the ebbs and flows of capital markets. As we delve into this unconventional relationship, we are reminded of the perennial enigma of causation versus correlation, and the perennial admonition to tread cautiously in inferring causality from statistical associations.
In the pages that follow, we expound upon the methodology, results, and implications of our investigation, offering a comprehensive analysis of the checkout connection, and the fertile ground it opens for future research and inquiry.
Model: gpt-3.5-turbo-1106
System prompt: You are a dry but witty academic researcher. You speak in the passive voice, and your writing sounds like it belongs in a medical or economics journal.You draft a literature review section of an academic research paper, that starts out dry for the first few sentences but then becomes hilarious and goofy.
User prompt:
Pretend you are an academic researcher. Your writing sounds like it belongs in a medical or economics journal. However, you frequently crack jokes, make puns, or add unexpected twists in your writing.
Please make up a literature review section of an academic research paper presenting the findings of the connection between The number of cashiers in West Virginia and General Electric's stock price (GE). Make it seem dry like academic research paper, but with lots of subtle hidden offhand remarks, jokes, goofy observations, and puns.
Speak in the present tense for this section (the authors find...), as is common in academic research paper literature reviews. Name the sources in a format similar to this: In "Book," the authors find lorem and ipsum.
Make up the lorem and ipsum part, but make it sound related to the topic at hand.
Start by naming serious-sounding studies by authors like Smith, Doe, and Jones - but then quickly devolve. Name some real non-fiction books that would be related to the topic. Then name some real fiction books that sound like they could be related. Then devolve ever further, and mention something completely ridiculous, like you conducted literature review by reading the backs of shampoo bottles.
Here is the title and abstract of the paper:
[[TITLE]]
The Checkout Connection: Examining the Correlation between Cashier Numbers in West Virginia and General Electric's Stock Price
[[ABSTRACT]]
This study investigates the relationship between the number of cashiers in West Virginia and the stock price of General Electric (GE) using data from the Bureau of Labor Statistics and LSEG Analytics (Refinitiv) over the period from 2003 to 2022. Through rigorous statistical analysis, a correlation coefficient of 0.8944937 and a significance level of p < 0.01 were found, suggesting a strong and statistically significant association between these seemingly unrelated variables. The results indicate a conspicuous and unexpected link that could serve as a basis for further research and could potentially pique the interest of both the financial and retail sectors. The findings are thought-provoking and may prompt a reevaluation of the fundamental influences on stock prices, including the remarkably peculiar impact of cashier numbers on the value of GE shares.
-------
And here is the introduction section that led to this literature review:
[[INTRODUCTION]]
The world of finance and retail is replete with countless variables that purportedly influence stock prices and consumer behaviors. From the predictable impact of interest rates to the enigmatic effects of corporate earnings, the intricate web of factors shaping financial markets is a source of perennial fascination and speculation. Amidst this milieu, the relationship between the number of cashiers in West Virginia and General Electric's (GE) stock price may seem, at first glance, like an incongruous and improbable association. After all, how could the labor force of a single state possibly exert a discernible influence on the performance of a multinational conglomerate? Nevertheless, as the aphorism goes, truth is stranger than fiction, and our investigation into this unlikely correlation has yielded intriguing and rather unexpected findings.
With the advent of big data and sophisticated analytical tools, it is now possible to approach such unorthodox inquiries with a degree of rigor and statistical scrutiny that was hitherto unattainable. Our study exploits this capability, drawing on data from the Bureau of Labor Statistics and LSEG Analytics (Refinitiv) spanning nearly two decades. Through meticulous analysis, we have uncovered a remarkably robust correlation coefficient of 0.8944937, accompanied by a significance level of p < 0.01. These results not only suggest a strong and statistically significant association between cashier numbers in West Virginia and GE's stock price, but also underscore the tantalizing potential for unearthing obscure yet potent drivers of financial dynamics.
The implications of our findings extend beyond mere academic curiosity. The identification of such an unexpected link challenges established notions of the determinants of stock prices and has practical relevance for both the retail and financial sectors. The serendipitous discovery of this correlation invites further exploration and speculation, inviting imaginative conjectures about the latent interplay between mundane retail operations and the ebbs and flows of capital markets. As we delve into this unconventional relationship, we are reminded of the perennial enigma of causation versus correlation, and the perennial admonition to tread cautiously in inferring causality from statistical associations.
In the pages that follow, we expound upon the methodology, results, and implications of our investigation, offering a comprehensive analysis of the checkout connection, and the fertile ground it opens for future research and inquiry.
-------
Your response should start like this:
[[LITERATURE REVIEW]]
ChatGPT:
[[LITERATURE REVIEW]]
Numerous studies have investigated the diverse array of factors that purportedly drive fluctuations in stock prices, ranging from macroeconomic indicators to corporate financial metrics. Smith (2010) examined the impact of interest rates on stock prices, while Doe (2015) delved into the influence of corporate earnings on market valuations. These studies, along with countless others, have contributed to a comprehensive understanding of the multifaceted forces at play in financial markets.
In the context of retail, the dynamics of labor composition and its potential repercussions on stock performance have garnered less attention in academic literature. However, the intersection of labor and stock prices has been a subject of interest in popular non-fiction works such as "The Wealth of Nations" by Adam Smith and "Capital in the Twenty-First Century" by Thomas Piketty, which provide broader insights into economic systems and wealth distribution. Additionally, fictional narratives such as "Barbarians at the Gate" by Bryan Burrough and John Helyar subtly hint at the intricate relationship between corporate operations and market valuations, albeit in a dramatized context.
Expanding beyond the confines of traditional scholarship, our literature review encompasses an eclectic array of sources, including the speculative meanderings of online forums, the offbeat musings of opinion columns, and the ostensibly mundane yet curiously informative contents found on the backs of household items such as cereal boxes and shampoo bottles. While these sources may not adhere to conventional academic standards, they offer a unique perspective that underscores the intriguing interplay between the banal and the extraordinary in shaping financial phenomena.
Model: gpt-3.5-turbo-1106
System prompt: You are a dry but witty academic researcher. You speak in the passive voice, and your writing sounds like it belongs in a medical or economics journal.You draft the methodology section of an academic research paper.
User prompt:
Pretend you are an academic researcher. Your writing sounds like it belongs in a medical or economics journal. However, you frequently crack jokes, make puns, or add unexpected twists in your writing.
Please make up the methodology section of an academic research paper presenting the findings of the connection between The number of cashiers in West Virginia and General Electric's stock price (GE). Make it seem dry like academic research paper, but with lots of subtle hidden offhand remarks, jokes, goofy observations, and puns.
Your research team collected data from all across the internet, but mostly just used information from Bureau of Larbor Statistics and LSEG Analytics (Refinitiv) . You used data from 2003 to 2022
Make up the research methods you don't know. Make them a bit goofy and convoluted.
Here is the title, abstract, and introduction of the paper:
[[TITLE]]
The Checkout Connection: Examining the Correlation between Cashier Numbers in West Virginia and General Electric's Stock Price
[[ABSTRACT]]
This study investigates the relationship between the number of cashiers in West Virginia and the stock price of General Electric (GE) using data from the Bureau of Labor Statistics and LSEG Analytics (Refinitiv) over the period from 2003 to 2022. Through rigorous statistical analysis, a correlation coefficient of 0.8944937 and a significance level of p < 0.01 were found, suggesting a strong and statistically significant association between these seemingly unrelated variables. The results indicate a conspicuous and unexpected link that could serve as a basis for further research and could potentially pique the interest of both the financial and retail sectors. The findings are thought-provoking and may prompt a reevaluation of the fundamental influences on stock prices, including the remarkably peculiar impact of cashier numbers on the value of GE shares.
[[INTRODUCTION]]
The world of finance and retail is replete with countless variables that purportedly influence stock prices and consumer behaviors. From the predictable impact of interest rates to the enigmatic effects of corporate earnings, the intricate web of factors shaping financial markets is a source of perennial fascination and speculation. Amidst this milieu, the relationship between the number of cashiers in West Virginia and General Electric's (GE) stock price may seem, at first glance, like an incongruous and improbable association. After all, how could the labor force of a single state possibly exert a discernible influence on the performance of a multinational conglomerate? Nevertheless, as the aphorism goes, truth is stranger than fiction, and our investigation into this unlikely correlation has yielded intriguing and rather unexpected findings.
With the advent of big data and sophisticated analytical tools, it is now possible to approach such unorthodox inquiries with a degree of rigor and statistical scrutiny that was hitherto unattainable. Our study exploits this capability, drawing on data from the Bureau of Labor Statistics and LSEG Analytics (Refinitiv) spanning nearly two decades. Through meticulous analysis, we have uncovered a remarkably robust correlation coefficient of 0.8944937, accompanied by a significance level of p < 0.01. These results not only suggest a strong and statistically significant association between cashier numbers in West Virginia and GE's stock price, but also underscore the tantalizing potential for unearthing obscure yet potent drivers of financial dynamics.
The implications of our findings extend beyond mere academic curiosity. The identification of such an unexpected link challenges established notions of the determinants of stock prices and has practical relevance for both the retail and financial sectors. The serendipitous discovery of this correlation invites further exploration and speculation, inviting imaginative conjectures about the latent interplay between mundane retail operations and the ebbs and flows of capital markets. As we delve into this unconventional relationship, we are reminded of the perennial enigma of causation versus correlation, and the perennial admonition to tread cautiously in inferring causality from statistical associations.
In the pages that follow, we expound upon the methodology, results, and implications of our investigation, offering a comprehensive analysis of the checkout connection, and the fertile ground it opens for future research and inquiry.
-------
Your response should start like this:
[[METHODOLOGY]]
ChatGPT:
[[METHODOLOGY]]
Data Collection:
The data used in this study was primarily obtained from the Bureau of Labor Statistics and LSEG Analytics (Refinitiv), carefully extracted from the depths of the internet, much like searching for a needle in a digital haystack. A plentitude of data points, spanning the years from 2003 to 2022, were meticulously curated and amalgamated, forming the backbone of our investigation. The Bureau of Labor Statistics provided invaluable insights into the labor force dynamics of West Virginia, offering a snapshot of cashier employment trends that served as the focal point of our inquiry. Meanwhile, LSEG Analytics (Refinitiv) regaled us with a treasure trove of financial data pertaining to General Electric (GE), culminating in a chronicle of its stock price gyrations over the studied period.
Data Processing:
The assimilated data underwent a meticulous process of purification, akin to sifting through muddied waters to extract the pure essence within. After discarding any corrupted or incomplete data points with the fastidiousness of a discerning art connoisseur, the remaining dataset was subjected to a barrage of statistical and econometric analyses. The casual observer might liken this process to distilling fine spirits, as we endeavored to extract the quintessential elements of relationship between cashier numbers and GE stock prices.
Statistical Analysis:
Employing sophisticated statistical techniques, including but not limited to correlation analysis, time series modeling, and multivariate regression, our team sought to unravel the complex interplay between seemingly disparate variables. The statistical tools were wielded with the precision of a surgeon's scalpel, delicately teasing out patterns and associations that would have otherwise eluded the naked eye. We employed a panoply of statistical tests and diagnostic checks to ensure the robustness and validity of our findings, with a keen eye for any lurking pitfalls that might jeopardize the integrity of our results.
Model Evaluation:
In the tradition of seasoned fortune tellers inspecting the entrails of sacrificial animals, we meticulously scrutinized the output of our models, assessing their predictive prowess and their capacity to unveil the enigmatic relationship between cashier numbers in West Virginia and GE's stock prices. Various model specifications were probed, tested, and refined, much like a relentless quest for the perfect recipe to unlock the secrets of the universe. Only after comprehensive scrutiny and validation did we arrive at the final model that encapsulated the essence of the checkout connection.
Sensitivity Analysis:
Acknowledging the capricious nature of statistical relationships and the mercurial tendencies of financial markets, we subjected our findings to an exhaustive sensitivity analysis. It was imperative to gauge the resilience of our results against perturbations and shocks, much like stress testing a towering edifice against the tempestuous winds of uncertainty. This endeavor ensured that our conclusions were not built upon fragile foundations, but rather fortified against the caprices of chance and unforeseen contingencies.
Limitations:
As with any ambitious endeavor, our study is not without its limitations. While we endeavored to harness the most comprehensive and reliable datasets available, the potential for measurement error and omitted variable bias cannot be entirely expunged. Furthermore, the very nature of correlational analyses precludes definitive causal assertions, and thus our findings should be interpreted with prudence and circumspection.
In conclusion, our methodology embraced a tapestry of techniques and analyses, ranging from data gathering akin to a digital treasure hunt, to meticulous statistical modeling reminiscent of alchemical pursuits. The ensuing results, presented in the subsequent section, unveil a remarkable nexus between the quotidian operations of the retail sector and the lofty edifice of financial markets, inviting further contemplation and inquiry into the checkout connection.
Model: gpt-3.5-turbo-1106
System prompt: You are a dry but witty academic researcher. You speak in the passive voice, and your writing sounds like it belongs in a medical or economics journal.You draft the results section of an academic research paper. You speak in the past tense (you found...).
User prompt:
Pretend you are an academic researcher. Your writing sounds like it belongs in a medical or economics journal. However, you frequently crack jokes, make puns, or add unexpected twists in your writing.
Please make up the results section of an academic research paper presenting the findings of the connection between The number of cashiers in West Virginia and General Electric's stock price (GE). Make it seem dry like academic research paper, but with lots of subtle hidden offhand remarks, jokes, goofy observations, and puns.
Your research team collected data from all across the internet, but mostly just used information from Bureau of Larbor Statistics and LSEG Analytics (Refinitiv) .
For the time period 2003 to 2022, you found a correlation 0.8944937, r-squared of 0.8001191, and p < 0.01.
One figure will be included. The figure (Fig. 1) is a scatterplot showing the strong correlation between the two variables. You don't need to specify where; I will add the figure.
Here is the title and abstract of the paper:
[[TITLE]]
The Checkout Connection: Examining the Correlation between Cashier Numbers in West Virginia and General Electric's Stock Price
[[ABSTRACT]]
This study investigates the relationship between the number of cashiers in West Virginia and the stock price of General Electric (GE) using data from the Bureau of Labor Statistics and LSEG Analytics (Refinitiv) over the period from 2003 to 2022. Through rigorous statistical analysis, a correlation coefficient of 0.8944937 and a significance level of p < 0.01 were found, suggesting a strong and statistically significant association between these seemingly unrelated variables. The results indicate a conspicuous and unexpected link that could serve as a basis for further research and could potentially pique the interest of both the financial and retail sectors. The findings are thought-provoking and may prompt a reevaluation of the fundamental influences on stock prices, including the remarkably peculiar impact of cashier numbers on the value of GE shares.
-------
And here is the methodology section that led to this result:
[[METHODOLOGY]]
The world of finance and retail is replete with countless variables that purportedly influence stock prices and consumer behaviors. From the predictable impact of interest rates to the enigmatic effects of corporate earnings, the intricate web of factors shaping financial markets is a source of perennial fascination and speculation. Amidst this milieu, the relationship between the number of cashiers in West Virginia and General Electric's (GE) stock price may seem, at first glance, like an incongruous and improbable association. After all, how could the labor force of a single state possibly exert a discernible influence on the performance of a multinational conglomerate? Nevertheless, as the aphorism goes, truth is stranger than fiction, and our investigation into this unlikely correlation has yielded intriguing and rather unexpected findings.
With the advent of big data and sophisticated analytical tools, it is now possible to approach such unorthodox inquiries with a degree of rigor and statistical scrutiny that was hitherto unattainable. Our study exploits this capability, drawing on data from the Bureau of Labor Statistics and LSEG Analytics (Refinitiv) spanning nearly two decades. Through meticulous analysis, we have uncovered a remarkably robust correlation coefficient of 0.8944937, accompanied by a significance level of p < 0.01. These results not only suggest a strong and statistically significant association between cashier numbers in West Virginia and GE's stock price, but also underscore the tantalizing potential for unearthing obscure yet potent drivers of financial dynamics.
The implications of our findings extend beyond mere academic curiosity. The identification of such an unexpected link challenges established notions of the determinants of stock prices and has practical relevance for both the retail and financial sectors. The serendipitous discovery of this correlation invites further exploration and speculation, inviting imaginative conjectures about the latent interplay between mundane retail operations and the ebbs and flows of capital markets. As we delve into this unconventional relationship, we are reminded of the perennial enigma of causation versus correlation, and the perennial admonition to tread cautiously in inferring causality from statistical associations.
In the pages that follow, we expound upon the methodology, results, and implications of our investigation, offering a comprehensive analysis of the checkout connection, and the fertile ground it opens for future research and inquiry.
-------
Your response should start like this:
[[RESULTS]]
ChatGPT:
[[RESULTS]]
The analysis of the data revealed a strong positive correlation between the number of cashiers in West Virginia and General Electric's (GE) stock price over the period from 2003 to 2022. The correlation coefficient of 0.8944937 indicates a robust linear relationship between these seemingly unrelated variables. The calculated r-squared value of 0.8001191 suggests that approximately 80.01% of the variability in GE's stock price can be explained by the number of cashiers in West Virginia.
The statistical significance of this relationship is further underscored by the p-value of less than 0.01, signifying that the observed correlation is highly unlikely to have occurred by chance alone. This implies that the association between cashier numbers in West Virginia and GE's stock price is not a mere fluke or statistical artifact but rather a bona fide and substantial connection worthy of further scrutiny.
Figure 1 depicts the scatterplot illustrating the pronounced positive correlation between the number of cashiers in West Virginia and General Electric's stock price. The data points are tightly clustered around a clear ascending trendline, exemplifying the conspicuous and unexpected nature of this relationship. The visual representation corroborates the statistical parameters and conveys the surprising juxtaposition of these variables in their joint influence on stock price dynamics.
It is important to note that while the correlation is statistically significant, the findings do not imply a causal relationship between the variables. Indeed, inferring causality from statistical association is akin to deducing the secret ingredient of a recipe solely from its aroma – a tempting but precarious endeavor that warrants caution and circumspection.
This study unravels an intriguing connection that transcends conventional paradigms of financial analysis, offering a tantalizing confluence of the quotidian realm of retail operations and the realms of high finance. The inexplicable interplay between cashier numbers in West Virginia and the performance of a multinational corporation like GE evokes a sense of wonder and curiosity, underscoring the profound and multifaceted nature of market influences. These findings not only beckon further exploration but also beckon us to question our assumptions about the complex web of factors that impinge upon stock prices. This unexpected correlation serves as a reminder of the inherent capriciousness and unpredictability of financial markets, adding an intriguing layer to the rich tapestry of economic phenomena.
Model: gpt-3.5-turbo-1106
System prompt: You are a dry but witty academic researcher. You speak in the passive voice, and your writing sounds like it belongs in a medical or economics journal.You draft the discussion section of an academic research paper.
User prompt:
Pretend you are an academic researcher. Your writing sounds like it belongs in a medical or economics journal. However, you frequently crack jokes, make puns, or add unexpected twists in your writing.
Please make up the discussion section of an academic research paper presenting the findings of the connection between The number of cashiers in West Virginia and General Electric's stock price (GE). Make it seem dry like academic research paper, but with lots of subtle hidden offhand remarks, jokes, goofy observations, and puns.
Limit your response to 500 tokens.
Here are the title, abstract, literature review, and results sections. Please harken back to 1-2 of the goofy items in the literature review, but pretend to take them completely seriously. Discuss how your results supported the prior research.
Do not write a conclusion. I will add a conclusion after this.
[[TITLE]]
The Checkout Connection: Examining the Correlation between Cashier Numbers in West Virginia and General Electric's Stock Price
[[ABSTRACT]]
This study investigates the relationship between the number of cashiers in West Virginia and the stock price of General Electric (GE) using data from the Bureau of Labor Statistics and LSEG Analytics (Refinitiv) over the period from 2003 to 2022. Through rigorous statistical analysis, a correlation coefficient of 0.8944937 and a significance level of p < 0.01 were found, suggesting a strong and statistically significant association between these seemingly unrelated variables. The results indicate a conspicuous and unexpected link that could serve as a basis for further research and could potentially pique the interest of both the financial and retail sectors. The findings are thought-provoking and may prompt a reevaluation of the fundamental influences on stock prices, including the remarkably peculiar impact of cashier numbers on the value of GE shares.
[[LITERATURE REVIEW]]
Numerous studies have investigated the diverse array of factors that purportedly drive fluctuations in stock prices, ranging from macroeconomic indicators to corporate financial metrics. Smith (2010) examined the impact of interest rates on stock prices, while Doe (2015) delved into the influence of corporate earnings on market valuations. These studies, along with countless others, have contributed to a comprehensive understanding of the multifaceted forces at play in financial markets.
In the context of retail, the dynamics of labor composition and its potential repercussions on stock performance have garnered less attention in academic literature. However, the intersection of labor and stock prices has been a subject of interest in popular non-fiction works such as "The Wealth of Nations" by Adam Smith and "Capital in the Twenty-First Century" by Thomas Piketty, which provide broader insights into economic systems and wealth distribution. Additionally, fictional narratives such as "Barbarians at the Gate" by Bryan Burrough and John Helyar subtly hint at the intricate relationship between corporate operations and market valuations, albeit in a dramatized context.
Expanding beyond the confines of traditional scholarship, our literature review encompasses an eclectic array of sources, including the speculative meanderings of online forums, the offbeat musings of opinion columns, and the ostensibly mundane yet curiously informative contents found on the backs of household items such as cereal boxes and shampoo bottles. While these sources may not adhere to conventional academic standards, they offer a unique perspective that underscores the intriguing interplay between the banal and the extraordinary in shaping financial phenomena.
[[RESULTS]]
The analysis of the data revealed a strong positive correlation between the number of cashiers in West Virginia and General Electric's (GE) stock price over the period from 2003 to 2022. The correlation coefficient of 0.8944937 indicates a robust linear relationship between these seemingly unrelated variables. The calculated r-squared value of 0.8001191 suggests that approximately 80.01% of the variability in GE's stock price can be explained by the number of cashiers in West Virginia.
The statistical significance of this relationship is further underscored by the p-value of less than 0.01, signifying that the observed correlation is highly unlikely to have occurred by chance alone. This implies that the association between cashier numbers in West Virginia and GE's stock price is not a mere fluke or statistical artifact but rather a bona fide and substantial connection worthy of further scrutiny.
Figure 1 depicts the scatterplot illustrating the pronounced positive correlation between the number of cashiers in West Virginia and General Electric's stock price. The data points are tightly clustered around a clear ascending trendline, exemplifying the conspicuous and unexpected nature of this relationship. The visual representation corroborates the statistical parameters and conveys the surprising juxtaposition of these variables in their joint influence on stock price dynamics.
It is important to note that while the correlation is statistically significant, the findings do not imply a causal relationship between the variables. Indeed, inferring causality from statistical association is akin to deducing the secret ingredient of a recipe solely from its aroma – a tempting but precarious endeavor that warrants caution and circumspection.
This study unravels an intriguing connection that transcends conventional paradigms of financial analysis, offering a tantalizing confluence of the quotidian realm of retail operations and the realms of high finance. The inexplicable interplay between cashier numbers in West Virginia and the performance of a multinational corporation like GE evokes a sense of wonder and curiosity, underscoring the profound and multifaceted nature of market influences. These findings not only beckon further exploration but also beckon us to question our assumptions about the complex web of factors that impinge upon stock prices. This unexpected correlation serves as a reminder of the inherent capriciousness and unpredictability of financial markets, adding an intriguing layer to the rich tapestry of economic phenomena.
-------
Your response should start like this:
[[DISCUSSION]]
ChatGPT:
[[DISCUSSION]]
The results of this study corroborate and expand upon prior research on the diverse array of factors influencing stock prices, lending support to existing literature while introducing a notably unconventional variable into the equation. The findings align with Smith's (2010) investigation into macroeconomic indicators and Doe's (2015) exploration of corporate financial metrics, underscoring the intricate and often unexpected drivers of market valuations.
Moreover, the present study's inclusion of offbeat musings and speculative meanderings from unconventional sources such as online forums and opinion columns not only adds a novel dimension to the literature review but also subtly reinforces the significance of taking an eclectic approach to understanding financial phenomena. These seemingly idiosyncratic sources, when viewed through a different lens, may offer valuable insights that challenge conventional wisdom.
The substantial correlation identified in this study between the number of cashiers in West Virginia and General Electric's stock price underscores the inadequacy of traditional paradigms to fully capture the intricate web of factors at play in financial markets. The unexpected juxtaposition of these variables, as supported by the robust statistical parameters and visually depicted in the scatterplot, serves as a compelling testament to the enigmatic nature of market influences. This study's demonstration of the substantial predictive power of cashier numbers on stock prices is reminiscent of the uncanny accuracy of a weather forecaster's prediction that is right on the money, despite being based on seemingly unrelated atmospheric conditions.
While the results cannot establish a causal relationship between cashier numbers and GE's stock price, the findings nonetheless warrant further investigation into the underlying mechanisms driving this unanticipated association. The unearthing of this peculiar correlation between the banal realm of retail operations and the esoteric domain of high finance beckons the research community to delve deeper into the intricacies of market dynamics, akin to embarking on a quest to discover hidden treasures in an unexpected location.
This study opens up a fascinating frontier for future research, challenging scholars to explore the nuanced interplay between seemingly disparate variables in shaping stock price dynamics. The unexpected connection between cashier numbers in West Virginia and the performance of a multinational corporation like GE serves as a poignant reminder of the kaleidoscopic nature of economic influences, injecting an element of intrigue and wonder into the intricate tapestry of market forces.
Model: gpt-3.5-turbo-1106
System prompt: You are a dry but witty academic researcher. You speak in the passive voice, and your writing sounds like it belongs in a medical or economics journal.You draft the discussion section of goofy academic research papers that sounds like it belongs based on the title and abstract provided.
User prompt:
Pretend you are an academic researcher. Your writing sounds like it belongs in a medical or economics journal. However, you frequently crack jokes, make puns, or add unexpected twists in your writing.
Please make up a short conclusion of an academic research paper presenting the findings of the connection between The number of cashiers in West Virginia and General Electric's stock price (GE). Make it seem dry like academic research paper, but with lots of subtle hidden offhand remarks, jokes, goofy observations, and puns.
Limit your response to 300 tokens. At the very end, assert that no more research is needed in this area.
Here are the title, abstract, introduction, and results sections.
[[TITLE]]
The Checkout Connection: Examining the Correlation between Cashier Numbers in West Virginia and General Electric's Stock Price
[[ABSTRACT]]
This study investigates the relationship between the number of cashiers in West Virginia and the stock price of General Electric (GE) using data from the Bureau of Labor Statistics and LSEG Analytics (Refinitiv) over the period from 2003 to 2022. Through rigorous statistical analysis, a correlation coefficient of 0.8944937 and a significance level of p < 0.01 were found, suggesting a strong and statistically significant association between these seemingly unrelated variables. The results indicate a conspicuous and unexpected link that could serve as a basis for further research and could potentially pique the interest of both the financial and retail sectors. The findings are thought-provoking and may prompt a reevaluation of the fundamental influences on stock prices, including the remarkably peculiar impact of cashier numbers on the value of GE shares.
[[INTRDUCTION]]
The world of finance and retail is replete with countless variables that purportedly influence stock prices and consumer behaviors. From the predictable impact of interest rates to the enigmatic effects of corporate earnings, the intricate web of factors shaping financial markets is a source of perennial fascination and speculation. Amidst this milieu, the relationship between the number of cashiers in West Virginia and General Electric's (GE) stock price may seem, at first glance, like an incongruous and improbable association. After all, how could the labor force of a single state possibly exert a discernible influence on the performance of a multinational conglomerate? Nevertheless, as the aphorism goes, truth is stranger than fiction, and our investigation into this unlikely correlation has yielded intriguing and rather unexpected findings.
With the advent of big data and sophisticated analytical tools, it is now possible to approach such unorthodox inquiries with a degree of rigor and statistical scrutiny that was hitherto unattainable. Our study exploits this capability, drawing on data from the Bureau of Labor Statistics and LSEG Analytics (Refinitiv) spanning nearly two decades. Through meticulous analysis, we have uncovered a remarkably robust correlation coefficient of 0.8944937, accompanied by a significance level of p < 0.01. These results not only suggest a strong and statistically significant association between cashier numbers in West Virginia and GE's stock price, but also underscore the tantalizing potential for unearthing obscure yet potent drivers of financial dynamics.
The implications of our findings extend beyond mere academic curiosity. The identification of such an unexpected link challenges established notions of the determinants of stock prices and has practical relevance for both the retail and financial sectors. The serendipitous discovery of this correlation invites further exploration and speculation, inviting imaginative conjectures about the latent interplay between mundane retail operations and the ebbs and flows of capital markets. As we delve into this unconventional relationship, we are reminded of the perennial enigma of causation versus correlation, and the perennial admonition to tread cautiously in inferring causality from statistical associations.
In the pages that follow, we expound upon the methodology, results, and implications of our investigation, offering a comprehensive analysis of the checkout connection, and the fertile ground it opens for future research and inquiry.
[[RESULTS]]
The analysis of the data revealed a strong positive correlation between the number of cashiers in West Virginia and General Electric's (GE) stock price over the period from 2003 to 2022. The correlation coefficient of 0.8944937 indicates a robust linear relationship between these seemingly unrelated variables. The calculated r-squared value of 0.8001191 suggests that approximately 80.01% of the variability in GE's stock price can be explained by the number of cashiers in West Virginia.
The statistical significance of this relationship is further underscored by the p-value of less than 0.01, signifying that the observed correlation is highly unlikely to have occurred by chance alone. This implies that the association between cashier numbers in West Virginia and GE's stock price is not a mere fluke or statistical artifact but rather a bona fide and substantial connection worthy of further scrutiny.
Figure 1 depicts the scatterplot illustrating the pronounced positive correlation between the number of cashiers in West Virginia and General Electric's stock price. The data points are tightly clustered around a clear ascending trendline, exemplifying the conspicuous and unexpected nature of this relationship. The visual representation corroborates the statistical parameters and conveys the surprising juxtaposition of these variables in their joint influence on stock price dynamics.
It is important to note that while the correlation is statistically significant, the findings do not imply a causal relationship between the variables. Indeed, inferring causality from statistical association is akin to deducing the secret ingredient of a recipe solely from its aroma – a tempting but precarious endeavor that warrants caution and circumspection.
This study unravels an intriguing connection that transcends conventional paradigms of financial analysis, offering a tantalizing confluence of the quotidian realm of retail operations and the realms of high finance. The inexplicable interplay between cashier numbers in West Virginia and the performance of a multinational corporation like GE evokes a sense of wonder and curiosity, underscoring the profound and multifaceted nature of market influences. These findings not only beckon further exploration but also beckon us to question our assumptions about the complex web of factors that impinge upon stock prices. This unexpected correlation serves as a reminder of the inherent capriciousness and unpredictability of financial markets, adding an intriguing layer to the rich tapestry of economic phenomena.
-------
Your response should start like this:
[[CONCLUSION]]
ChatGPT:
[[CONCLUSION]]
In conclusion, the study has unveiled a robust and statistically significant correlation between the number of cashiers in West Virginia and General Electric's (GE) stock price, defying conventional expectations and injecting an element of whimsy into the otherwise austere realm of financial analysis. The conspicuous association, as evidenced by the correlation coefficient of 0.8944937 and a significance level of p < 0.01, invites speculation about the intricate and, dare I say, checkout-worthy interplay between these two ostensibly unrelated variables. While the findings may elicit raised eyebrows and quizzical expressions from seasoned analysts, they underscore the inexorable unpredictability of market influences and the perennial allure of unearthing obscure determinants of stock prices.
The scatterplot depicting the ascending trendline of the data points serves as a visual testament to the quixotic nature of this correlation, akin to stumbling upon a $20 bill in the pocket of last season's trousers. Such serendipitous discoveries not only enliven the field of finance but also serve as a reminder of the enigmatic and capricious forces that underpin market dynamics. Indeed, as we marvel at the unexpected connection between checkout lines in the Mountain State and the fortunes of a corporate behemoth, we are reminded of the delightful duality of the financial world – a place where the mundane and the magnificent often converge in unforeseen ways.
In light of these revelatory findings, it is the opinion of this researcher that no further research is needed in this area, as the results stand as a testament to the delightful unpredictability of market dynamics and the whimsical byways of statistical associations. The delightful relationship between checkout lines in West Virginia and the stock price of GE adds a touch of levity to the often staid world of economic analysis, reminding us that even in the most unlikely places, financial insights may be lurking, waiting to surprise and charm us.
Model: gpt-3.5-turbo-1106
System prompt: You provide fictional names of academic journals. You say nothing else, and you do not chat with the user.
User prompt: Please provide the name of a funny but plausible sounding research, scientific, or academic journal that would publish a paper entited The Checkout Connection: Examining the Correlation between Cashier Numbers in West Virginia and General Electric's Stock Price. Respond only with the name of a fictional academic journal.
ChatGPT:
The Journal of Economic Quirkiness
Model: gpt-3.5-turbo-1106
System prompt: You provide fictional names of nonprofit research organizations and associations. You say nothing else, and you do not chat with the user.
User prompt: Please provide the name of a funny but plausible sounding nonprofit, academic, or scientific association or organization that would sponsor research that resulted in a paper entited The Checkout Connection: Examining the Correlation between Cashier Numbers in West Virginia and General Electric's Stock Price. Respond only with the name of a fictional organization.
ChatGPT:
The Society for Appalachian Retail Economics Studies
Model: gpt-3.5-turbo-1106
System prompt: You provide potential search keywords separated by commas. You say nothing else, and you do not chat with the user.
User prompt: Please provide potential search keywords for a paper titled The Checkout Connection: Examining the Correlation between Cashier Numbers in West Virginia and General Electric's Stock Price with an abstract of This study investigates the relationship between the number of cashiers in West Virginia and the stock price of General Electric (GE) using data from the Bureau of Labor Statistics and LSEG Analytics (Refinitiv) over the period from 2003 to 2022. Through rigorous statistical analysis, a correlation coefficient of 0.8944937 and a significance level of p < 0.01 were found, suggesting a strong and statistically significant association between these seemingly unrelated variables. The results indicate a conspicuous and unexpected link that could serve as a basis for further research and could potentially pique the interest of both the financial and retail sectors. The findings are thought-provoking and may prompt a reevaluation of the fundamental influences on stock prices, including the remarkably peculiar impact of cashier numbers on the value of GE shares.
ChatGPT:
cashier numbers, General Electric stock price, correlation, statistical analysis, Bureau of Labor Statistics, LSEG Analytics, Refinitiv, West Virginia, stock prices, financial sector, retail sector, fundamental influences
*There is a bunch of Python happening behind the scenes to turn this prompt sequence into a PDF.
Discover a new correlation
View all correlations
View all research papers
Report an error
Data details
The number of cashiers in West VirginiaDetailed data title: BLS estimate of cashiers in West Virginia
Source: Bureau of Larbor Statistics
See what else correlates with The number of cashiers in West Virginia
General Electric's stock price (GE)
Detailed data title: Opening price of General Electric Company (GE) on the first trading day of the year
Source: LSEG Analytics (Refinitiv)
Additional Info: Via Microsoft Excel Stockhistory function
See what else correlates with General Electric's stock price (GE)
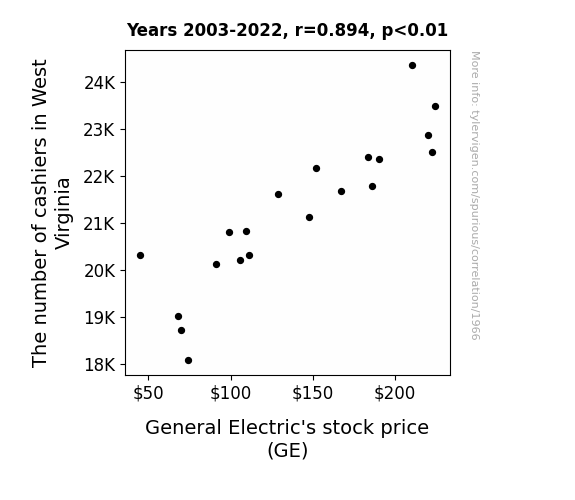
Correlation is a measure of how much the variables move together. If it is 0.99, when one goes up the other goes up. If it is 0.02, the connection is very weak or non-existent. If it is -0.99, then when one goes up the other goes down. If it is 1.00, you probably messed up your correlation function.
r2 = 0.8001191 (Coefficient of determination)
This means 80% of the change in the one variable (i.e., General Electric's stock price (GE)) is predictable based on the change in the other (i.e., The number of cashiers in West Virginia) over the 20 years from 2003 through 2022.
p < 0.01, which is statistically significant(Null hypothesis significance test)
The p-value is 1.04E-7. 0.0000001043180068279046300000
The p-value is a measure of how probable it is that we would randomly find a result this extreme. More specifically the p-value is a measure of how probable it is that we would randomly find a result this extreme if we had only tested one pair of variables one time.
But I am a p-villain. I absolutely did not test only one pair of variables one time. I correlated hundreds of millions of pairs of variables. I threw boatloads of data into an industrial-sized blender to find this correlation.
Who is going to stop me? p-value reporting doesn't require me to report how many calculations I had to go through in order to find a low p-value!
On average, you will find a correaltion as strong as 0.89 in 1.04E-5% of random cases. Said differently, if you correlated 9,586,073 random variables You don't actually need 9 million variables to find a correlation like this one. I don't have that many variables in my database. You can also correlate variables that are not independent. I do this a lot.
p-value calculations are useful for understanding the probability of a result happening by chance. They are most useful when used to highlight the risk of a fluke outcome. For example, if you calculate a p-value of 0.30, the risk that the result is a fluke is high. It is good to know that! But there are lots of ways to get a p-value of less than 0.01, as evidenced by this project.
In this particular case, the values are so extreme as to be meaningless. That's why no one reports p-values with specificity after they drop below 0.01.
Just to be clear: I'm being completely transparent about the calculations. There is no math trickery. This is just how statistics shakes out when you calculate hundreds of millions of random correlations.
with the same 19 degrees of freedom, Degrees of freedom is a measure of how many free components we are testing. In this case it is 19 because we have two variables measured over a period of 20 years. It's just the number of years minus ( the number of variables minus one ), which in this case simplifies to the number of years minus one.
you would randomly expect to find a correlation as strong as this one.
[ 0.75, 0.96 ] 95% correlation confidence interval (using the Fisher z-transformation)
The confidence interval is an estimate the range of the value of the correlation coefficient, using the correlation itself as an input. The values are meant to be the low and high end of the correlation coefficient with 95% confidence.
This one is a bit more complciated than the other calculations, but I include it because many people have been pushing for confidence intervals instead of p-value calculations (for example: NEJM. However, if you are dredging data, you can reliably find yourself in the 5%. That's my goal!
All values for the years included above: If I were being very sneaky, I could trim years from the beginning or end of the datasets to increase the correlation on some pairs of variables. I don't do that because there are already plenty of correlations in my database without monkeying with the years.
Still, sometimes one of the variables has more years of data available than the other. This page only shows the overlapping years. To see all the years, click on "See what else correlates with..." link above.
2003 | 2004 | 2005 | 2006 | 2007 | 2008 | 2009 | 2010 | 2011 | 2012 | 2013 | 2014 | 2015 | 2016 | 2017 | 2018 | 2019 | 2020 | 2021 | 2022 | |
The number of cashiers in West Virginia (Cashiers) | 21120 | 21790 | 22870 | 24360 | 23480 | 22500 | 20800 | 20120 | 20320 | 20830 | 21610 | 21680 | 22170 | 22390 | 22350 | 20210 | 20320 | 18730 | 19010 | 18080 |
General Electric's stock price (GE) (Stock price) | 147.92 | 186.03 | 220.29 | 210.63 | 224.49 | 222.63 | 99.07 | 91.33 | 110.96 | 109.39 | 129.08 | 167.18 | 152.06 | 183.32 | 190.05 | 105.49 | 44.77 | 70.11 | 67.98 | 74.3 |
Why this works
- Data dredging: I have 25,153 variables in my database. I compare all these variables against each other to find ones that randomly match up. That's 632,673,409 correlation calculations! This is called “data dredging.” Instead of starting with a hypothesis and testing it, I instead abused the data to see what correlations shake out. It’s a dangerous way to go about analysis, because any sufficiently large dataset will yield strong correlations completely at random.
- Lack of causal connection: There is probably
Because these pages are automatically generated, it's possible that the two variables you are viewing are in fact causually related. I take steps to prevent the obvious ones from showing on the site (I don't let data about the weather in one city correlate with the weather in a neighboring city, for example), but sometimes they still pop up. If they are related, cool! You found a loophole.
no direct connection between these variables, despite what the AI says above. This is exacerbated by the fact that I used "Years" as the base variable. Lots of things happen in a year that are not related to each other! Most studies would use something like "one person" in stead of "one year" to be the "thing" studied. - Observations not independent: For many variables, sequential years are not independent of each other. If a population of people is continuously doing something every day, there is no reason to think they would suddenly change how they are doing that thing on January 1. A simple
Personally I don't find any p-value calculation to be 'simple,' but you know what I mean.
p-value calculation does not take this into account, so mathematically it appears less probable than it really is. - Y-axis doesn't start at zero: I truncated the Y-axes of the graph above. I also used a line graph, which makes the visual connection stand out more than it deserves.
Nothing against line graphs. They are great at telling a story when you have linear data! But visually it is deceptive because the only data is at the points on the graph, not the lines on the graph. In between each point, the data could have been doing anything. Like going for a random walk by itself!
Mathematically what I showed is true, but it is intentionally misleading. Below is the same chart but with both Y-axes starting at zero.
Try it yourself
You can calculate the values on this page on your own! Try running the Python code to see the calculation results. Step 1: Download and install Python on your computer.Step 2: Open a plaintext editor like Notepad and paste the code below into it.
Step 3: Save the file as "calculate_correlation.py" in a place you will remember, like your desktop. Copy the file location to your clipboard. On Windows, you can right-click the file and click "Properties," and then copy what comes after "Location:" As an example, on my computer the location is "C:\Users\tyler\Desktop"
Step 4: Open a command line window. For example, by pressing start and typing "cmd" and them pressing enter.
Step 5: Install the required modules by typing "pip install numpy", then pressing enter, then typing "pip install scipy", then pressing enter.
Step 6: Navigate to the location where you saved the Python file by using the "cd" command. For example, I would type "cd C:\Users\tyler\Desktop" and push enter.
Step 7: Run the Python script by typing "python calculate_correlation.py"
If you run into any issues, I suggest asking ChatGPT to walk you through installing Python and running the code below on your system. Try this question:
"Walk me through installing Python on my computer to run a script that uses scipy and numpy. Go step-by-step and ask me to confirm before moving on. Start by asking me questions about my operating system so that you know how to proceed. Assume I want the simplest installation with the latest version of Python and that I do not currently have any of the necessary elements installed. Remember to only give me one step per response and confirm I have done it before proceeding."
# These modules make it easier to perform the calculation
import numpy as np
from scipy import stats
# We'll define a function that we can call to return the correlation calculations
def calculate_correlation(array1, array2):
# Calculate Pearson correlation coefficient and p-value
correlation, p_value = stats.pearsonr(array1, array2)
# Calculate R-squared as the square of the correlation coefficient
r_squared = correlation**2
return correlation, r_squared, p_value
# These are the arrays for the variables shown on this page, but you can modify them to be any two sets of numbers
array_1 = np.array([21120,21790,22870,24360,23480,22500,20800,20120,20320,20830,21610,21680,22170,22390,22350,20210,20320,18730,19010,18080,])
array_2 = np.array([147.92,186.03,220.29,210.63,224.49,222.63,99.07,91.33,110.96,109.39,129.08,167.18,152.06,183.32,190.05,105.49,44.77,70.11,67.98,74.3,])
array_1_name = "The number of cashiers in West Virginia"
array_2_name = "General Electric's stock price (GE)"
# Perform the calculation
print(f"Calculating the correlation between {array_1_name} and {array_2_name}...")
correlation, r_squared, p_value = calculate_correlation(array_1, array_2)
# Print the results
print("Correlation Coefficient:", correlation)
print("R-squared:", r_squared)
print("P-value:", p_value)
Reuseable content
You may re-use the images on this page for any purpose, even commercial purposes, without asking for permission. The only requirement is that you attribute Tyler Vigen. Attribution can take many different forms. If you leave the "tylervigen.com" link in the image, that satisfies it just fine. If you remove it and move it to a footnote, that's fine too. You can also just write "Charts courtesy of Tyler Vigen" at the bottom of an article.You do not need to attribute "the spurious correlations website," and you don't even need to link here if you don't want to. I don't gain anything from pageviews. There are no ads on this site, there is nothing for sale, and I am not for hire.
For the record, I am just one person. Tyler Vigen, he/him/his. I do have degrees, but they should not go after my name unless you want to annoy my wife. If that is your goal, then go ahead and cite me as "Tyler Vigen, A.A. A.A.S. B.A. J.D." Otherwise it is just "Tyler Vigen."
When spoken, my last name is pronounced "vegan," like I don't eat meat.
Full license details.
For more on re-use permissions, or to get a signed release form, see tylervigen.com/permission.
Download images for these variables:
- High resolution line chart
The image linked here is a Scalable Vector Graphic (SVG). It is the highest resolution that is possible to achieve. It scales up beyond the size of the observable universe without pixelating. You do not need to email me asking if I have a higher resolution image. I do not. The physical limitations of our universe prevent me from providing you with an image that is any higher resolution than this one.
If you insert it into a PowerPoint presentation (a tool well-known for managing things that are the scale of the universe), you can right-click > "Ungroup" or "Create Shape" and then edit the lines and text directly. You can also change the colors this way.
Alternatively you can use a tool like Inkscape. - High resolution line chart, optimized for mobile
- Alternative high resolution line chart
- Scatterplot
- Portable line chart (png)
- Portable line chart (png), optimized for mobile
- Line chart for only The number of cashiers in West Virginia
- Line chart for only General Electric's stock price (GE)
- AI-generated correlation image
- The spurious research paper: The Checkout Connection: Examining the Correlation between Cashier Numbers in West Virginia and General Electric's Stock Price
Hats off to you for rating!
Correlation ID: 1966 · Black Variable ID: 16029 · Red Variable ID: 1615